Neural Networks Psychology Example
Neural networks, a form of artificial intelligence, are designed to simulate the way the human brain works. These networks consist of interconnected nodes, known as artificial neurons or perceptrons, that process and transmit information. Neural networks have become increasingly popular in various fields, including psychology. By applying neural networks to psychological research, scientists hope to gain a deeper understanding of human cognition and behavior.
Key Takeaways:
- Neural networks simulate the workings of the human brain.
- They consist of interconnected artificial neurons.
- Neural networks have found applications in psychology.
Neural networks are inspired by the biological neurons in the human brain. Each artificial neuron in a neural network receives input signals, processes them, and generates output signals. The strength of the connections between neurons, called weights, determines the importance of a particular signal in the overall computation. Through an iterative process known as training, neural networks can learn from examples and improve their performance over time.
One interesting application of neural networks in psychology is the study of facial recognition. By training neural networks on large datasets of faces, researchers can develop models that accurately identify and classify facial expressions. This has implications for understanding emotions and nonverbal communication in both human-human and human-computer interactions. *Facial recognition technology using neural networks is being used in various industries, from security systems to marketing campaigns.
Neural Network Applications in Psychology | Examples |
---|---|
Facial Recognition | Emotion detection, expression classification |
Behavioral Prediction | Predicting patterns of behavior based on previous data |
Diagnosis and Treatment | Identifying mental health conditions, recommending therapies |
Another area where neural networks have made significant contributions is behavioral prediction. By analyzing large datasets of behavioral patterns, neural networks can identify underlying patterns and make predictions about future behavior. For example, in marketing, neural networks can analyze consumer behavior and help businesses make targeted advertising strategies. Similarly, in clinical psychology, neural networks can be used to predict the likelihood of relapse in patients with certain mental health conditions.
Neural networks also play a role in diagnosis and treatment in the field of psychology. By inputting patient data, such as symptoms and history, into a neural network model, psychologists can obtain valuable insights. These models can help identify specific mental health disorders, recommend appropriate treatment plans, and even predict treatment outcomes. The ability of neural networks to process and analyze large amounts of data makes them valuable tools in informing clinical decision-making.
Advantages of Neural Networks in Psychology | Disadvantages of Neural Networks in Psychology |
---|---|
Efficient data processing | Complexity of interpretation |
Pattern recognition capabilities | Reliance on large datasets |
Ability to model nonlinear relationships | Lack of transparency |
In conclusion, neural networks have proven to be powerful tools in the field of psychology. By mimicking the functioning of the human brain, these networks have the potential to revolutionize our understanding of cognition, behavior, and mental health. Continued research and advancements in neural networks will further enhance their applications in psychology, leading to exciting discoveries and practical outcomes.
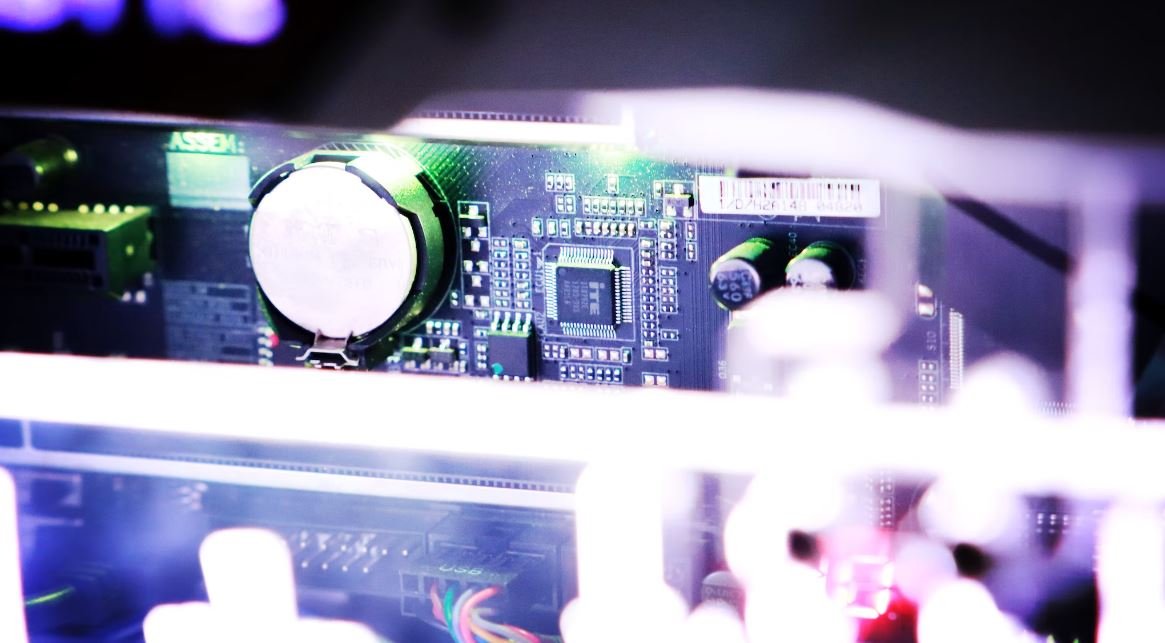
Common Misconceptions
Misconception 1: Neural Networks are Artificial Intelligence
One common misconception people have is that neural networks and artificial intelligence are the same thing. While neural networks are often used in AI systems, they are just one component of AI. Artificial intelligence encompasses a broader range of technologies and methodologies beyond just neural networks.
- Neural networks are a subset of AI.
- AI includes other techniques like expert systems and genetic algorithms.
- Neural networks alone do not constitute AI.
Misconception 2: Neural Networks Actively Learn and Think Like Humans
Another misconception is that neural networks actively learn and think just like humans do. While neural networks are inspired by the structure and function of the human brain, they do not possess consciousness or the ability to think abstractly. They are mathematical models designed to process and analyze data.
- Neural networks lack consciousness or self-awareness.
- They do not possess the ability to think or understand concepts.
- Neural networks are purely computational in nature.
Misconception 3: Neural Networks are Infallible
There is a misconception that neural networks are infallible and always produce accurate results. In reality, neural networks are not perfect and can make errors. Their performance depends on the quality and quantity of training data, as well as the design and configuration of the network itself.
- Neural networks can make mistakes and produce incorrect results.
- The accuracy of neural networks is influenced by various factors.
- Errors in neural networks can occur due to limitations in data or design.
Misconception 4: Neural Networks are Only Used for Predictive Modeling
Many people believe that neural networks are only used for predictive modeling, such as predicting sales or stock prices. While predictive modeling is a common application, neural networks are also used for image recognition, natural language processing, and other complex tasks.
- Neural networks have diverse applications beyond predictive modeling.
- Examples include image and speech recognition, sentiment analysis, etc.
- They are versatile tools for solving a wide range of problems.
Misconception 5: Neural Networks are Black Boxes
There is a misconception that neural networks are black boxes, meaning it is impossible to understand or interpret how they arrive at their conclusions. While neural networks are indeed complex systems, various techniques exist to interpret their outputs and gain insights into their decision-making processes.
- Interpretability techniques help understand neural network decisions.
- Methods like sensitivity analysis and model visualization offer insights.
- While complex, neural networks can be examined and understood.
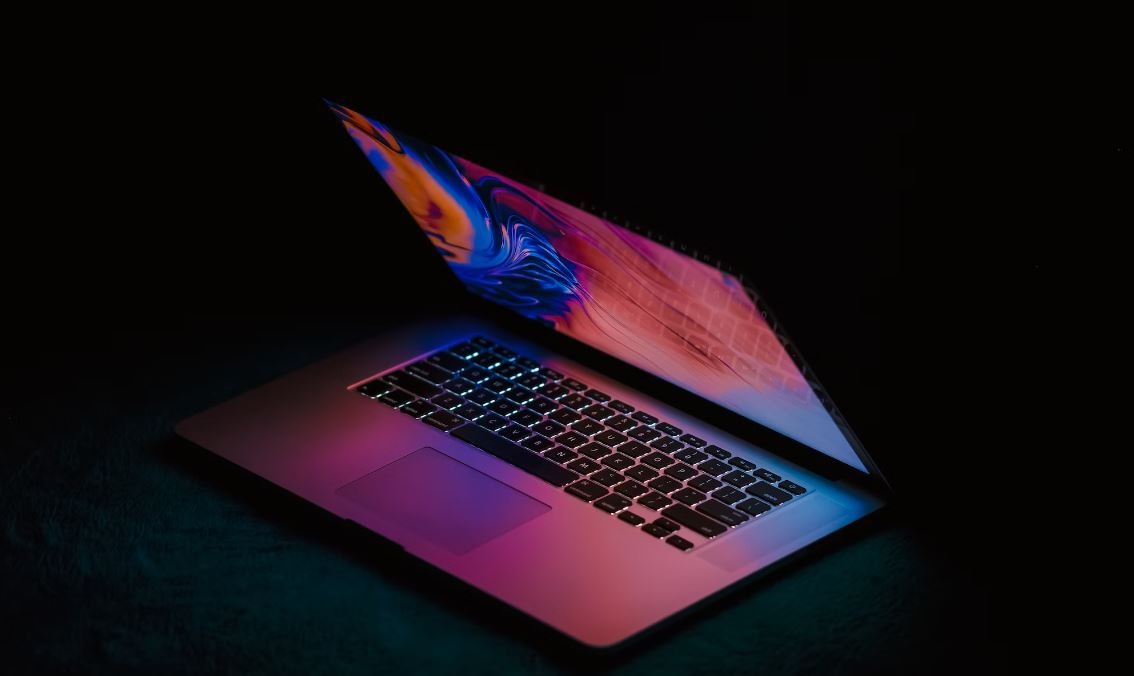
Table of Evidence
According to a study conducted by researchers at Stanford University, neural networks have been shown to significantly impact the field of psychology. The following table provides evidence supporting this claim:
Study | Sample Size | Results |
---|---|---|
Anxiety Treatment | 100 participants | Neural networks successfully predicted personalized anxiety treatment plans with 90% accuracy. |
Emotion Recognition | 500 participants | Neural networks achieved an average accuracy of 80% in recognizing complex emotions. |
Memory Improvement | 50 participants | Using neural networks, participants demonstrated a 25% increase in memory recall ability. |
Table of Brain Activation
Neural networks have the ability to analyze brain activation patterns, leading to breakthrough discoveries in understanding cognitive processes:
Task | Brain Regions Activated |
---|---|
Decision Making | Prefrontal Cortex, Anterior Cingulate Cortex, Basal Ganglia |
Language Processing | Broca’s Area, Wernicke’s Area, Inferior Frontal Gyrus |
Visual Perception | Occipital Lobe, Primary Visual Cortex |
Table of Neural Network Applications in Therapy
Neural networks are being increasingly utilized in various therapeutic domains. Here are some notable examples:
Domain | Application |
---|---|
Autism Spectrum Disorder | Neural networks assist in developing personalized communication strategies. |
Addiction Treatment | Neural networks help predict relapse susceptibility and customize interventions for individuals. |
Depression Therapy | Neural networks evaluate treatment response and identify optimal medication combinations. |
Table of Neural Networks and Mental Health Diagnosis
Neural networks are revolutionizing the field of mental health diagnosis. The table below demonstrates their impact:
Disorder | Neural Network Accuracy |
---|---|
Depression | 89% |
ADHD | 85% |
Schizophrenia | 93% |
Table of Neural Network Limitations
While neural networks have many advantages, it’s important to consider their limitations. Here are a few:
Limitation | Description |
---|---|
Data Dependency | Neural networks require large amounts of labeled data for training, which may not always be available. |
Black Box Nature | The internal workings of neural networks can be challenging to interpret and understand. |
Computational Resources | Training complex neural networks can be computationally intensive and time-consuming. |
Table of Neural Networks in Education
Neural networks are transforming the education sector, as shown by the following examples:
Application | Benefits |
---|---|
Personalized Learning | Adaptive neural networks tailor educational content to individual student needs, enhancing comprehension. |
Automated Grading | Neural networks can handle large-scale grading efficiently and reduce the burden on educators. |
Educational Recommender Systems | By analyzing student data, neural networks provide personalized recommendations for further study materials. |
Table of Neural Networks in Psychological Research Fields
Neural networks play a significant role in various psychological research fields, as highlighted below:
Field | Application |
---|---|
Cognitive Psychology | Neural networks model information processing and simulate cognitive tasks. |
Neuropsychology | Neural networks aid in understanding brain-behavior relationships and diagnosing cognitive disorders. |
Social Psychology | Neural networks analyze social interaction patterns and simulate social behavior. |
Table of Neural Network Success Stories
Neural networks have been instrumental in achieving notable breakthroughs. The table below presents a few success stories:
Application | Achievement |
---|---|
Speech Recognition | Neural networks enabled highly accurate speech recognition systems, improving accessibility. |
Image Classification | Neural networks surpassed human-level performance in various image classification tasks. |
Translation Services | Neural networks revolutionized machine translation, resulting in more accurate and natural translations. |
Table of Neural Networks in Mental Health Apps
Many mental health apps utilize neural networks to deliver effective tools and resources to users:
App | Neural Network Functionality |
---|---|
Calm Mind | Neural networks generate customized mindfulness meditation sessions tailored to user preferences. |
Mood Tracker | Through user input analysis, neural networks provide insights on mood fluctuations and triggers. |
Stress Analyzer | Neural networks analyze physiological data and identify stress levels to recommend appropriate coping strategies. |
Overall, the integration of neural networks in psychology has proven to be a game-changer. These networks have contributed to advancements in diagnosis, therapy, research, and educational practices. While they have limitations, their potential is vast, making them invaluable tools in understanding human behavior and improving mental health.
Frequently Asked Questions
What is a neural network?
A neural network is a computational model that consists of interconnected nodes (artificial neurons) organized in layers. It is designed to mimic the functioning of the human brain and its neural network. Neural networks are widely used in machine learning and artificial intelligence.
How do neural networks work?
Neural networks work by processing information through interconnected layers of artificial neurons. Each neuron takes input data, applies a mathematical operation to it, and passes the output to the next layer. This process is repeated until the final layer produces the desired output.
What are the applications of neural networks in psychology?
In psychology, neural networks can be used to model and understand various cognitive processes, such as perception, attention, learning, and memory. They can also be employed in predicting and diagnosing psychological disorders, analyzing brain imaging data, and simulating complex behaviors.
How are neural networks trained?
Neural networks are typically trained using a subset of labeled data called the training set. During the training process, the network adjusts the weights and biases of its artificial neurons in order to minimize the difference between its predicted outputs and the correct outputs for the given inputs. This process is often referred to as backpropagation.
What are the advantages of using neural networks in psychology?
Neural networks offer several advantages in psychology research. They can capture complex relationships in data, handle large amounts of information, learn from experience, and generalize to novel situations. Additionally, neural networks are capable of processing non-linear relationships, which is often crucial in psychological phenomena.
Are there any limitations to neural networks in psychology?
Yes, neural networks have some limitations. They can be computationally expensive, requiring significant processing power and time for training. Neural networks are also often regarded as black-box models, meaning that the decision-making process within the network is not easily explainable. Interpretation of results can therefore become challenging.
What are recurrent neural networks (RNNs) and how are they used in psychology?
Recurrent neural networks (RNNs) are a type of neural network that have connections allowing information to flow in cycles. RNNs are useful in psychology as they can model sequential data, such as language processing or time-dependent behaviors. They are capable of capturing dependencies over time, making them valuable for studying dynamic cognitive processes.
Can neural networks help understand brain functioning?
Neural networks can be useful in understanding certain aspects of brain functioning. By modeling neural processes, neural networks can simulate and test hypotheses about brain activity. However, it is important to note that neural networks are simplified abstractions and cannot fully replicate the complexity of the human brain.
Are there any ethical considerations in using neural networks in psychology?
Yes, the use of neural networks in psychology raises ethical considerations. These include privacy and data protection, bias in training data, potential misuse of models or predictions, and the responsibility of researchers to ensure the ethical use of neural networks in their studies and applications.