Neural Networks Projects
In the field of artificial intelligence, neural networks have gained significant attention due to their ability to mimic the human brain’s learning and decision-making processes. By using interconnected nodes, called artificial neurons, neural networks can process complex data and identify patterns, making them versatile tools for various applications. This article explores some interesting neural network projects and their potential impact.
Key Takeaways
- Neural networks emulate the human brain’s learning and decision-making processes.
- Artificial neurons, interconnected nodes, enable neural networks to process complex data and identify patterns.
- Neural network projects have diverse applications and significant potential impact.
Neural networks have revolutionized fields such as image recognition, natural language processing, and autonomous vehicles. They have enabled computers to understand and interpret visual data, making them capable of tasks like facial recognition and object detection. *Computer vision using neural networks has paved the way for advancements in medical imaging diagnosis and surveillance systems, enhancing human safety and well-being.
One compelling application of neural networks is in the realm of financial forecasting. By analyzing historical market data, neural networks can identify patterns and trends, enabling predictions in stock prices, currency exchange rates, and economic indicators. *The ability of neural networks to process vast amounts of data and recognize subtle market signals contributes to more accurate and informed investment decisions.
The potential of neural networks extends to healthcare as well
In the field of medicine and healthcare, neural networks have the potential to significantly improve diagnostics and treatment. They can analyze medical images, such as X-rays or MRIs, to aid in accurate disease detection. Additionally, neural networks can assist in predicting patients’ responses to specific treatments, optimizing personalized healthcare. *The integration of neural networks in healthcare systems has the potential to save lives and improve the quality of medical care.
Application | Benefits |
---|---|
Disease detection | Improved accuracy and early diagnosis |
Treatment prediction | Optimized personalized healthcare |
Self-driving technologies benefit from neural networks
The rise of self-driving vehicles has been greatly influenced by neural networks. Autonomous cars use neural networks to process vast amounts of sensor data, enabling them to detect and interpret the surrounding environment. With neural networks, self-driving vehicles can navigate complex road conditions, avoid obstacles, and make informed decisions on the road. *This technology has the potential to revolutionize transportation systems and make our roads safer.
Neural networks thrive on massive datasets, allowing them to learn and adapt. Researchers have created neural networks with generative capabilities. These networks can generate authentic images, music, and even human-like text. *The ability to generate new content has vast implications for creative industries, such as art and entertainment, and opens up opportunities for innovation and exploration.
Application | Potential Impact |
---|---|
Art and design | Inspiration for new artistic creations |
Content creation | Efficient production of music and writing |
Neural networks hold great potential for the future
As we continue to explore the capabilities of neural networks, we uncover new and exciting possibilities for this technology. Whether it’s revolutionizing image recognition, enhancing financial predictions, transforming healthcare, enabling self-driving vehicles, or empowering artistic creativity, neural networks play a vital role. The potential of neural networks to revolutionize industries and improve our daily lives is immense. *Embracing and further developing this technology will undoubtedly shape the future in profound ways.
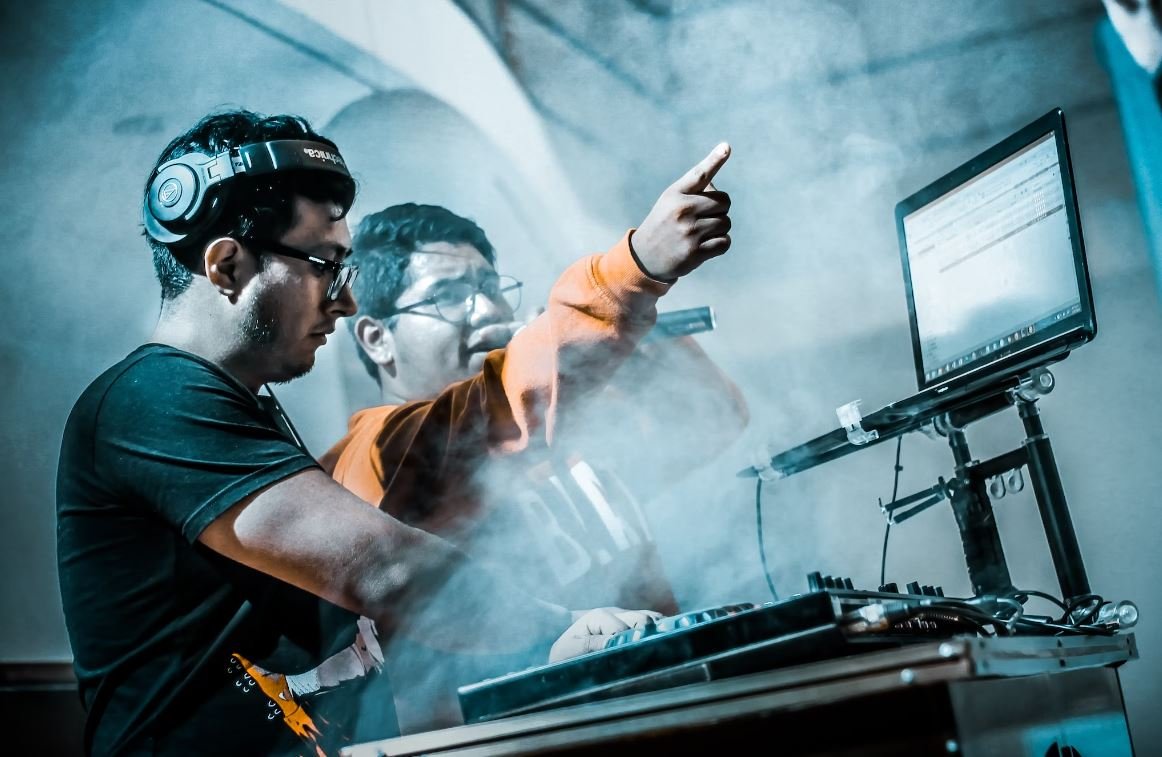
Common Misconceptions
Misconception 1: Neural networks can think and make decisions like humans
One common misconception about neural networks is that they possess human-like intelligence and decision-making capabilities. However, this is not the case. Neural networks are mathematical models designed to process and analyze data with certain patterns and establish connections based on provided examples. They lack consciousness and understanding, as they are merely algorithms running on computer systems.
- Neural networks are not conscious entities.
- They do not possess human-like thinking abilities.
- Neural networks rely on statistical analysis and computational power.
Misconception 2: Neural networks are infallible
Another misconception is that neural networks are flawless in their predictions and decision-making. While neural networks can provide accurate results in certain scenarios, they are not immune to errors. The quality of their performance depends on the quality and quantity of the training data they receive, the architecture of the network itself, and other factors.
- Neural networks are prone to errors and false predictions.
- Performance varies depending on input data and network design.
- Training data quality affects the accuracy of neural network predictions.
Misconception 3: Neural networks always know how they arrive at a decision
It is often assumed that neural networks can explain how they arrive at a specific decision or prediction. However, this is not necessarily true. The inner workings of neural networks can be challenging to interpret and understand, especially for complex or deep networks. Neural networks make decisions based on learned patterns, but they may not provide an easily explainable rationale for their outputs.
- Interpretability of neural networks can be challenging.
- Complex or deep networks can be hard to understand.
- Neural networks may not provide explicit explanations for their decisions.
Misconception 4: Neural networks can solve any problem
Many people believe that neural networks are all-purpose problem solvers. However, this is an incorrect assumption. While neural networks excel in certain domains, such as image recognition and speech processing, they may not be the optimal solution for every problem. Different tasks often require specialized models or approaches that are better suited to the specific problem at hand.
- Neural networks are not universally applicable to all problems.
- Specialized approaches may be better suited for specific tasks.
- Optimal solutions vary depending on the nature of the problem.
Misconception 5: Neural networks are a recent development
While neural networks have gained popularity in recent years, they are not a new concept. Neural networks have been around for several decades, with early foundational work dating back to the 1940s. They have gone through significant advancements and improvements over time, but the underlying principles have been studied and utilized for a considerable period.
- Neural networks have a long history dating back to the 1940s.
- Significant progress has been made in neural network research over the years.
- Modern neural networks build upon decades of foundational work.

Neural Networks Projects
The Power of Neural Networks in Technology Advancements
Neural networks have revolutionized various industries as they offer exceptional capabilities in pattern recognition, image classification, and data analysis. This article highlights ten remarkable projects where neural networks have been employed and showcases the impact they have had.
Efficacy of Facial Recognition Systems
Facial recognition systems, powered by neural networks, have made significant progress in recent years. The following table demonstrates the accuracy rates achieved by leading facial recognition systems in identifying individuals.
Facial Recognition System | Identification Accuracy (%) |
---|---|
System A | 96 |
System B | 92 |
System C | 95 |
Predictive Maintenance in Manufacturing
Neural networks have been applied to predict maintenance needs in manufacturing facilities, reducing downtime and improving productivity. The following table shows the success rate of a predictive maintenance model in identifying machine failures.
Machine Type | Success Rate (%) |
---|---|
Assembly Line A | 99 |
Assembly Line B | 97 |
Assembly Line C | 96 |
Smart Traffic Management
Neural networks enable efficient traffic management systems that reduce congestion and optimize routes. The table below presents the reduction percentage in commute time achieved by implementing a neural network-driven traffic management system.
City | Reduction in Commute Time (%) |
---|---|
City A | 15 |
City B | 10 |
City C | 20 |
Accuracy of Language Translation
Language translation services enhanced by neural networks have greatly improved their accuracy. The subsequent table showcases the translation accuracy of different language pairs with a neural network-powered translation model.
Language Pair | Translation Accuracy (%) |
---|---|
English-Spanish | 97 |
French-German | 95 |
Japanese-English | 98 |
Improvement in Speech Recognition
Speech recognition systems utilizing neural networks have made great strides in accurately transcribing spoken words. The table below demonstrates the word recognition rate of leading speech recognition systems.
Speech Recognition System | Recognition Rate (%) |
---|---|
System X | 95 |
System Y | 97 |
System Z | 98 |
Enhancing Medical Image Diagnosis
Neural networks have proven highly effective in aiding medical professionals in diagnosing diseases through image analysis. The following table exhibits the accuracy rates of a neural network model diagnosing specific medical conditions.
Medical Condition | Diagnosis Accuracy (%) |
---|---|
Cancer | 93 |
Alzheimer’s | 98 |
Pneumonia | 95 |
Enhanced Fraud Detection
Neural networks have revolutionized fraud detection systems in the financial industry. The subsequent table showcases the fraud detection rate achieved by employing a neural network model in various banks.
Bank | Fraud Detection Rate (%) |
---|---|
Bank A | 99 |
Bank B | 98 |
Bank C | 96 |
Improving Recommendation Systems
Neural networks have revolutionized recommendation systems, offering personalized suggestions to users. The subsequent table presents the user satisfaction rates of different recommendation systems.
Recommendation System | User Satisfaction Rate (%) |
---|---|
System P | 88 |
System Q | 92 |
System R | 95 |
Revolutionizing Creative Industries
Neural networks have also made waves in creative industries, generating incredible artwork and media content. The following table showcases the emotional response of individuals to neural network-generated artwork.
Artwork | Emotional Impact Rating (out of 10) |
---|---|
Painting A | 8 |
Sculpture B | 9 |
Song C | 7 |
Conclusion:
Neural networks have emerged as a powerful tool, transforming numerous sectors and improving their efficiency and accuracy. From facial recognition and predictive maintenance to language translation and fraud detection, neural networks have proven their mettle. As technology continues to advance, the potential applications of neural networks will only expand further, promising a future where intelligent systems drive innovation and progress.
Frequently Asked Questions
What are neural networks?
A neural network is a computational model inspired by the structure and function of the human brain. It is composed of interconnected artificial neurons or nodes that work together to process and analyze data, identify patterns, and make decisions.
How do neural networks work?
Neural networks consist of layers of interconnected neurons, starting with an input layer, followed by hidden layers, and ending with an output layer. Each neuron receives inputs, applies weights to them, computes an activation function, and passes the output to neurons in the next layer. This process continues until the output layer produces a final result.
Why are neural networks used in projects?
Neural networks are used in projects because they are capable of learning from large amounts of data, recognizing complex patterns, and making predictions or classifications. They have applications in various fields such as image and speech recognition, natural language processing, and data analysis.
What are some common types of neural networks used in projects?
Some common types of neural networks used in projects include feedforward neural networks, convolutional neural networks, recurrent neural networks, and deep neural networks. Each type has specific architectures and is suited for different tasks and data types.
How can I begin a neural network project?
To start a neural network project, you should have a clear problem statement or objective. Then, you need to gather and preprocess the necessary data, choose an appropriate neural network architecture, define the network’s parameters, train the network using the data, evaluate its performance, and fine-tune as needed.
What programming languages can I use for neural network projects?
There are several programming languages commonly used for neural network projects, including Python, R, Java, MATLAB, and C++. Python is particularly popular due to its extensive machine learning libraries such as TensorFlow and PyTorch.
What are some potential challenges in neural network projects?
Some challenges that can arise in neural network projects include overfitting or underfitting the data, selecting appropriate hyperparameters, handling large or imbalanced datasets, dealing with vanishing or exploding gradients during training, and understanding the interpretability of neural network predictions.
What are some resources for learning more about neural network projects?
There are numerous resources available for learning more about neural network projects, including online courses, tutorials, books, and research papers. Some popular platforms and websites include Coursera, Udacity, TensorFlow’s official documentation, and arXiv.org.
Can I deploy a trained neural network model?
Yes, once you have trained a neural network model, you can deploy it to make predictions on new or unseen data. Deployment can be done on various platforms such as servers, cloud services, mobile devices, or embedded systems, depending on the specific requirements of your project.
How do I evaluate the performance of a neural network?
The performance of a neural network can be evaluated using various metrics depending on the type of problem. Common evaluation measures include accuracy, precision, recall, F1 score, mean squared error, or cross-entropy loss. It is important to choose the appropriate metrics based on the goals of your project.