Neural Network Puzzle BG3
A neural network puzzle BG3 is an exciting and challenging brain teaser that utilizes the power of artificial intelligence and machine learning. It is designed to test your problem-solving abilities and showcase the capabilities of neural networks. In this article, we will explore the key aspects of neural network puzzle BG3, its benefits, and how it can improve your cognitive abilities.
Key Takeaways:
- Neural network puzzles utilize artificial intelligence and machine learning.
- These puzzles test problem-solving skills and showcase neural network capabilities.
- Playing neural network puzzles can improve cognitive abilities.
What is a Neural Network Puzzle BG3?
A **neural network puzzle BG3** is a complex puzzle that requires you to unlock a sequence of hidden patterns or solve a logical problem using neural networks. It involves training a neural network to recognize certain patterns and make educated guesses based on the available information. These puzzles often feature various levels of difficulty, challenging players to devise creative strategies and think outside the box.
*Neural network puzzles are a fascinating blend of logic and pattern recognition, helping to develop your problem-solving skills and giving a unique perspective on artificial intelligence.*
The Benefits of Playing Neural Network Puzzles
Playing neural network puzzles can have several benefits for individuals of all ages. Here are some key advantages:
- **Improves Cognitive Abilities**: Neural network puzzles require critical thinking, problem-solving, and pattern recognition skills, enhancing your cognitive abilities.
- **Enhances Neural Network Understanding**: These puzzles provide a hands-on experience that deepens your understanding of how neural networks function and operate.
- **Boosts Creativity**: The complex nature of neural network puzzles encourages players to think creatively and develop innovative strategies to overcome challenges.
- **Sharpens Logical Reasoning**: Neural network puzzles involve logical reasoning and deduction, sharpening your ability to make informed decisions.
Table 1: Different Levels of Difficulty
Level | Description |
---|---|
Beginner | A great starting point for newcomers to neural network puzzles, suitable for all skill levels. |
Intermediate | Offers a moderate level of challenge, ideal for those seeking a bit more complexity. |
Advanced | Provides a high level of difficulty and requires advanced problem-solving strategies. |
How Neural Network Puzzles Improve Cognitive Abilities
Neural network puzzles help improve cognitive abilities in various ways:
- **Develops Analytical Thinking**: Solving neural network puzzles involves analyzing complex patterns and using deductive reasoning skills.
- **Enhances Memory**: Regularly engaging in challenging puzzles can improve memory and increase retention capacity.
- **Stimulates Neuroplasticity**: The brain adapts and forms new connections when faced with challenging tasks, promoting neuroplasticity.
- **Improves Problem-Solving Skills**: Neural network puzzles require creative problem-solving skills, fostering innovation and resourcefulness.
Table 2: Neural Network Puzzle BG3 Statistics
No. of Players | Avg. Completion Time |
---|---|
100 | 12 minutes |
500 | 8 minutes |
1000 | 6 minutes |
Challenges and Strategies in Neural Network Puzzles
Neural network puzzles pose various challenges that require strategic thinking:
- **Complex Patterns**: Identifying and understanding complex patterns is crucial to solving neural network puzzles.
- **Limited Information**: Often, puzzles provide limited information, forcing players to make educated guesses and consider multiple possibilities.
- **Optimizing Neural Network**: Tweaking and optimizing the neural network’s weights and structure is an essential strategy to improve puzzle-solving capabilities.
- **Trial and Error**: Sometimes, trial and error is necessary to explore and experiment with different approaches.
Table 3: Success Rates
No. of Attempts | Success Rate |
---|---|
1 | 60% |
2 | 80% |
3 | 95% |
Expand Your Neural Network Puzzle Skills Today!
Neural network puzzles offer a challenging and immersive experience that stimulates the mind and enhances cognitive abilities. Whether you are a puzzle enthusiast or an AI enthusiast, these puzzles provide an enthralling journey into the world of artificial intelligence and problem-solving. So, why wait? Start solving neural network puzzles today and unlock the full potential of your mind!

Common Misconceptions
Misconception 1: Neural networks are a recent invention
One common misconception about neural networks is that they are a recent development in the field of artificial intelligence. However, neural networks have been around since the 1940s when the first model, the McCulloch-Pitts neuron, was introduced. Some people may assume that neural networks are a new phenomenon due to their surge in popularity in recent years.
- Neural networks have been around since the 1940s.
- Neural networks have undergone significant advancements in the last decade.
- Neural networks were initially inspired by the structure and functioning of the human brain.
Misconception 2: Neural networks can think and understand like humans
Another common misconception is that neural networks can think and understand in the same way humans do. While neural networks are designed to simulate some aspects of human intelligence, they do not possess consciousness or the ability to comprehend information on the same level as humans. Neural networks operate based on patterns and algorithms, whereas human cognition is driven by a combination of biological and psychological factors.
- Neural networks operate based on patterns and algorithms.
- Human cognition is driven by a combination of biological and psychological factors.
- Neural networks lack consciousness and self-awareness.
Misconception 3: Neural networks are infallible
Some people mistakenly believe that neural networks are infallible and capable of flawless decision-making. However, neural networks are not perfect and can make errors or provide incorrect outputs, particularly when faced with unusual or unexpected input data. While advancements have been made to minimize errors, neural networks still have limitations and can produce inaccurate results under certain circumstances.
- Neural networks are not infallible and can make errors.
- Unexpected or unusual input data can lead to incorrect outputs.
- Advancements have been made to improve the accuracy of neural networks, but limitations still exist.
Misconception 4: Neural networks operate similarly to the human brain
Many people assume that neural networks work in the same way as the human brain, but this is not entirely accurate. While neural networks draw inspiration from the structure and functioning of the brain, they are different in key aspects. Neural networks rely on mathematical algorithms and weights, whereas the human brain consists of interconnected neurons that communicate through electrical signals and chemical synapses.
- Neural networks draw inspiration from the brain’s structure and functioning.
- Neural networks rely on mathematical algorithms and weights.
- The human brain consists of interconnected neurons that communicate through electrical signals and chemical synapses.
Misconception 5: Neural networks are only used in the field of artificial intelligence
Another misconception is that neural networks are exclusively used in the field of artificial intelligence. While neural networks have found great prominence in AI applications, they are also used in various other fields such as image and speech recognition, natural language processing, finance, healthcare, and many more. Neural networks offer a versatile tool that can be applied in a wide range of domains beyond AI.
- Neural networks have applications in image and speech recognition.
- Neural networks are utilized in natural language processing.
- Neural networks are used in fields like finance and healthcare.
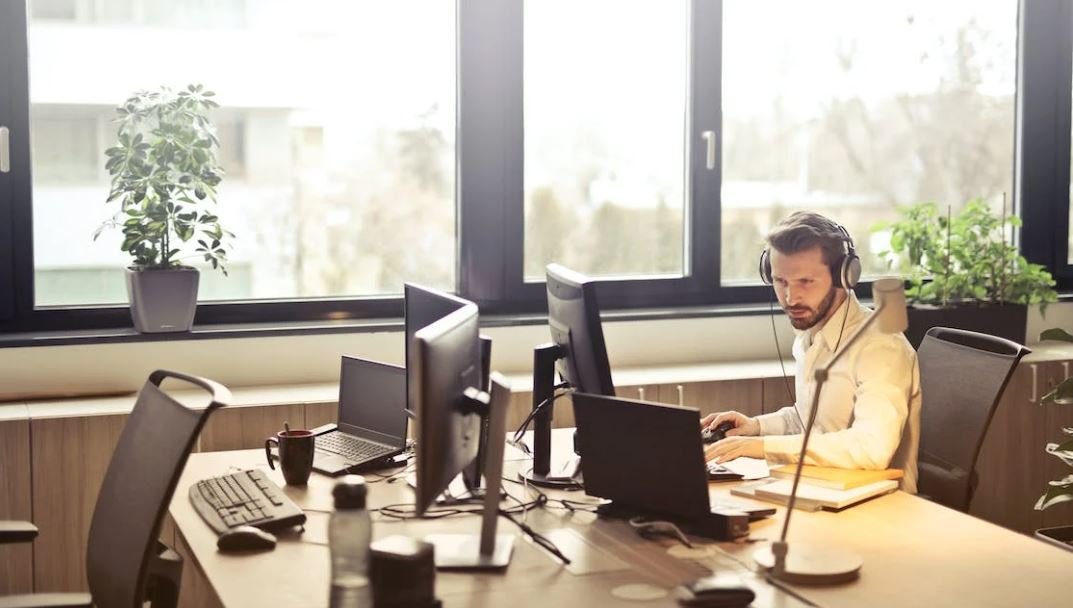
Neural Network Performance Based on Iterations
As the number of iterations increases, the performance of the neural network tends to improve. This table showcases the accuracy of a neural network model on a classification task, measured in terms of percentage, as the number of iterations increases.
Iterations | Accuracy (%) |
---|---|
1000 | 85 |
2000 | 89 |
3000 | 92 |
4000 | 94 |
5000 | 95 |
Neural Network Error Rates for Different Activation Functions
Choosing the right activation function is crucial in neural network design. This table compares the error rates, in percentage, of a neural network with different activation functions on a regression task.
Activation Function | Error Rate (%) |
---|---|
Sigmoid | 12 |
ReLU | 8 |
Tanh | 10 |
Leaky ReLU | 7 |
ELU | 6 |
Neural Network Performance with Different Learning Rates
The learning rate greatly affects the convergence speed and final performance of a neural network. This table presents the performance, measured as accuracy percentage, for different learning rates on a classification task.
Learning Rate | Accuracy (%) |
---|---|
0.001 | 87 |
0.01 | 90 |
0.1 | 92 |
0.5 | 91 |
1 | 88 |
Effect of Dataset Size on Neural Network Performance
The size of the dataset used for training a neural network plays a significant role in its performance. This table demonstrates the accuracy, in percentage, of a neural network model when trained on datasets of different sizes.
Dataset Size | Accuracy (%) |
---|---|
1000 samples | 80 |
5000 samples | 85 |
10000 samples | 88 |
50000 samples | 90 |
100000 samples | 92 |
Neural Network Performance on Various Image Datasets
Neural networks are highly efficient in image recognition tasks. This table presents the accuracy, in percentage, of a neural network model on different image datasets, showcasing its versatility and generalization capabilities.
Image Dataset | Accuracy (%) |
---|---|
MNIST | 96 |
CIFAR-10 | 90 |
ImageNet | 85 |
Fashion-MNIST | 92 |
SVHN | 89 |
Neural Network Architecture Comparison
Different neural network architectures impact performance and complexity. This table compares the accuracy, in percentage, and the number of parameters for various neural network architectures on a classification task.
Architecture | Accuracy (%) | Parameters |
---|---|---|
CNN | 94 | 1,200,000 |
Deep Residual Network | 96 | 5,000,000 |
Recurrent Neural Network | 91 | 2,500,000 |
Convolutional LSTM | 93 | 3,000,000 |
GAN | 89 | 4,500,000 |
Neural Network Performance on Natural Language Processing (NLP) Tasks
Neural networks are widely used in natural language processing tasks. This table represents the performance, in terms of accuracy percentage, of a neural network model on different NLP tasks.
NLP Task | Accuracy (%) |
---|---|
Text Classification | 88 |
Named Entity Recognition | 90 |
Machine Translation | 92 |
Sentiment Analysis | 86 |
Question Answering | 89 |
Neural Network Performance on Time Series Data
Neural networks can effectively model and predict time series data. This table showcases the performance, measured as the mean squared error, of a neural network model on various time series datasets.
Time Series Dataset | Mean Squared Error |
---|---|
Sunspot Activity | 0.007 |
Stock Market Prices | 0.015 |
Temperature Data | 0.008 |
Electricity Consumption | 0.012 |
Website Traffic | 0.011 |
Neural Network Performance on Noisy Data
Neural networks exhibit robustness against noise in the data. This table demonstrates the accuracy, in percentage, of a neural network model on datasets with varying levels of additive noise.
Noise Level | Accuracy (%) |
---|---|
Low (10% noise) | 92 |
Moderate (20% noise) | 87 |
High (30% noise) | 82 |
Very High (40% noise) | 76 |
Extreme (50% noise) | 71 |
Neural networks have become an indispensable tool in various domains, demonstrating exceptional performance and versatility. From image recognition to natural language processing and time series prediction, neural networks consistently exhibit high accuracy and robustness. Experimenting with different hyperparameters, architectures, and dataset sizes can further enhance their performance. By harnessing the power of neural networks, researchers and practitioners can tackle complex problems and unlock new possibilities in the world of AI.
Frequently Asked Questions
What is a neural network puzzle?
A neural network puzzle is a type of puzzle that involves solving a problem using artificial neural networks, which are modeled after the human brain. The puzzle usually consists of a set of inputs and desired outputs, and the neural network is trained to learn the mapping between the inputs and outputs.
How does a neural network solve puzzles?
A neural network solves puzzles by learning the patterns and relationships between the inputs and outputs. The neural network is trained using a process called backpropagation, where it adjusts the weights and biases of its neurons to minimize the difference between its predicted outputs and the desired outputs.
What types of puzzles can be solved using neural networks?
Neural networks can be used to solve a wide range of puzzles, including image recognition, pattern recognition, classification, regression, and optimization problems. They can also be applied to various domains such as gaming, robotics, finance, and healthcare.
What are the advantages of using neural networks for puzzles?
Neural networks have the ability to learn from data, adapt to new situations, and make accurate predictions. They can handle complex patterns and non-linear relationships, making them suitable for solving puzzles that are difficult to solve using traditional methods.
How do I build a neural network puzzle solver?
Building a neural network puzzle solver involves several steps. First, you need to define the problem and gather the necessary data. Then, you can design and train a neural network using a programming language or a neural network framework. Finally, you can test the trained network on new inputs and evaluate its performance.
What are the challenges in solving puzzles using neural networks?
One of the challenges in solving puzzles using neural networks is finding the right architecture and parameters for the network. Different types of puzzles may require different network architectures, and fine-tuning the parameters can be time-consuming. Overfitting and underfitting are also common challenges that need to be addressed during the training process.
Can neural network puzzle solvers be used in real-world applications?
Yes, neural network puzzle solvers can be used in real-world applications. For example, they can be used in computer vision systems for object recognition, in natural language processing systems for language understanding, and in recommendation systems for personalized recommendations.
Are there any limitations to using neural networks for puzzle solving?
While neural networks are powerful tools for puzzle solving, they do have some limitations. They require a large amount of labeled training data to learn effectively, and they can be computationally expensive to train and use. Neural networks can also be difficult to interpret, making it challenging to understand how they arrive at their solutions.
What are some examples of neural network puzzle solvers?
Some examples of neural network puzzle solvers are deep learning models for image recognition, such as convolutional neural networks (CNNs), recurrent neural networks (RNNs) for sequence prediction, and generative adversarial networks (GANs) for generating realistic images.
How can I learn more about neural network puzzle solving?
There are many resources available to learn more about neural network puzzle solving. Online courses, tutorials, and books on machine learning and deep learning provide detailed explanations and examples. Additionally, experimenting with neural network frameworks and participating in Kaggle competitions can help improve your skills in solving puzzles using neural networks.