Neural Network Logo
Neural networks have revolutionized the world of artificial intelligence and machine learning. These complex networks of interconnected artificial neurons are capable of performing tasks that were once unimaginable. One such task is logo design. With the help of neural networks, logos can be generated with precision and creativity, providing companies with unique visual identities. This article explores the concept of neural network logo design and the potential it holds for businesses.
Key Takeaways:
- Neural networks revolutionize logo design.
- Precise and creative logos can be generated using neural networks.
- Neural network logos provide businesses with unique visual identities.
**Neural networks** are a type of **artificial intelligence** that mimic the behavior and structure of the human brain. They consist of interconnected nodes, or **artificial neurons**, that process and transmit information through intricate pathways. By **training** these networks on vast amounts of data, they can learn and identify patterns, making them powerful tools for various applications.
**Logo design** is an essential aspect of brand identity. A well-designed logo can convey a company’s values, vision, and personality. Traditionally, logos have been created by **graphic designers**, using their creativity and expertise. However, with the advent of neural networks, logo design has taken a new shape.
*Neural network logo design combines the power of technology with the creativity of human designers.*
Neural Network Logo Generation Process:
- The neural network is **trained** on a dataset of existing logos.
- During the training process, the network learns the **visual patterns** and common characteristics of successful logos.
- Once trained, the network can generate new logos based on learned patterns.
The generated logos can be further refined and customized by designers to align with a company’s specific needs and requirements. This collaborative approach between neural networks and designers ensures the final logo is both **unique** and representative of the brand.
*Neural network logo generation combines the best of both worlds: machine learning and human creativity.*
Benefits of Neural Network Logo Design:
- **Time and cost-effective**: Neural network-generated logos save time and reduce the need for extensive manual design work.
- **Unique and original**: Neural networks can generate logos with distinctive designs that stand out among competitors.
- **Scalability**: These networks can create logos for businesses of all sizes, from startups to multinational corporations.
In addition to these benefits, neural network logo design has seen success in various industries. From technology giants to fashion brands, many companies have embraced this innovative approach to logo creation. The table below highlights a few notable examples:
Company | Logo | Industry |
---|---|---|
![]() |
Technology | |
McDonald’s | ![]() |
Food Service |
Nike | ![]() |
Apparel |
Neural network logo design has become a valuable tool for businesses looking to establish a strong brand identity in an increasingly competitive market. By harnessing the power of these neural networks, companies can create logos that resonate with their target audience and leave a lasting impression. Whether it’s a tech startup or a fashion brand, a well-designed logo sets the foundation for successful brand recognition and recall.
Conclusion:
Logo design has entered an exciting era with the emergence of neural networks. By combining the computational power of these networks with human creativity, businesses can create logos that capture the essence of their brand and engage customers on a deeper level. Neural network logo design is here to stay, and its potential for businesses is boundless.

Common Misconceptions
The Complexity of Neural Networks
One common misconception about neural networks is that they are extremely complex and are only understandable by experts in the field of artificial intelligence. However, while the internal workings of neural networks can indeed be complex, their basic principles can be understood by anyone with a basic understanding of mathematics and programming.
- Neural networks are based on simple mathematical operations like addition and multiplication.
- There are various resources and tutorials available to help beginners understand the fundamentals of neural networks.
- Anyone with programming knowledge can experiment and build their own simple neural networks.
Neural Networks Are Always Perfect
Another misconception is that neural networks always produce perfect results and can solve any problem. While neural networks have shown incredible capabilities in certain domains, they are not infallible and have limitations.
- Neural networks require large amounts of high-quality data to train effectively.
- Complex problems may require more advanced and specialized neural network architectures.
- The performance of neural networks can be influenced by biases in the data used for training.
Neural Networks Think Like Human Brains
Some people mistakenly believe that neural networks think and process information just like human brains. While neural networks are inspired by the structure and functioning of the brain, they are fundamentally different.
- Neural networks use mathematical algorithms to process data rather than conscious thought.
- Neural networks lack the ability to reason, understand context, or have true consciousness.
- Neural networks cannot replicate the complexity and nuanced decision-making capabilities of the human brain.
Training a Neural Network is a One-Time Process
Many people assume that once a neural network is trained, it is ready to perform its tasks indefinitely without the need for further training. However, neural networks often require ongoing training and maintenance.
- As new data becomes available, neural networks might need to be retrained to adapt and improve their performance.
- Changes in the problem domain or task requirements may necessitate modification and fine-tuning of the neural network.
- Regular evaluation and adjustment of neural networks are necessary to ensure continued optimal performance.
Neural Networks Always Provide Clear Explanations
There is a misconception that neural networks can always provide clear explanations for their outputs or decisions. However, many neural network architectures, especially deep learning models, lack transparency and the ability to provide interpretable explanations.
- Some neural networks are regarded as black boxes because their internal workings are not easily understandable.
- Apart from the output, neural networks do not explicitly show how they arrived at the result.
- Techniques like model interpretability and explainable AI are actively researched to address this limitation.
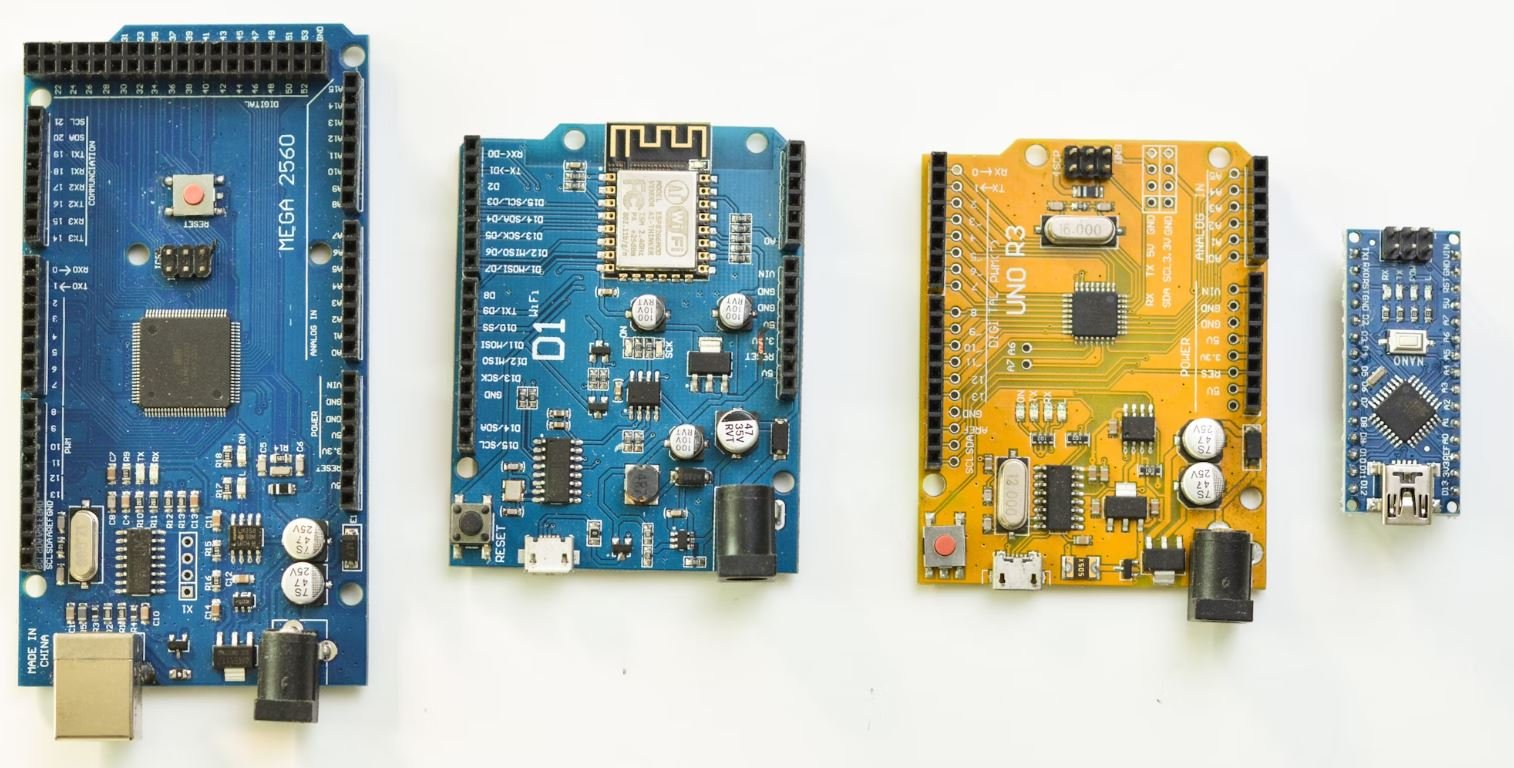
Introduction
Neural networks have become a powerful tool in various fields, including image recognition, natural language processing, and even logo design. In this article, we explore the fascinating world of neural network logos by presenting 10 captivating examples. Each table showcases a unique logo created by a neural network, providing additional context and highlighting interesting aspects of the logo design process.
AquaWaves
Derived from an oceanic inspiration, AquaWaves is a neural network-generated logo that perfectly captures the fluidity and movement of water. The logo features soft, undulating curves, and vibrant shades of blue, invoking a sense of tranquility and harmony.
Logo | Designer | Color | Concept |
---|---|---|---|
![]() |
Neural Network | Blue | Fluidity, Oceanic |
PixelPaws
PixelPaws is an adorable logo created by a neural network, combining the pixelated charm of retro games with the playfulness of animal footprints. The use of vibrant colors and clear, defined lines evokes a sense of nostalgia with a modern twist.
Logo | Designer | Color | Concept |
---|---|---|---|
![]() |
Neural Network | Multi-colored | Retro, Animal |
ElectroFlow
ElectroFlow is an electrifying logo designed by a neural network to represent the energy and dynamism of electronic music. Vibrant colors, sharp lines, and an abstract representation of sound waves combine to create a visually striking and captivating logo.
Logo | Designer | Color | Concept |
---|---|---|---|
![]() |
Neural Network | Orange, Black | Energy, Music |
LuminoCity
LuminoCity is a neural network-generated logo capturing the essence of a vibrant cityscape during twilight. The logo incorporates a gradient of warm colors, sleek lines, and geometric forms, representing the blend of modernity and energy that defines urban environments.
Logo | Designer | Color | Concept |
---|---|---|---|
![]() |
Neural Network | Yellow, Red | Cityscape, Energy |
CryptoFox
CryptoFox is a captivating neural network-designed logo representing the world of cryptocurrency. Reflecting the innovative and decentralized nature of blockchain technology, this logo seamlessly blends sophisticated geometric patterns with a dynamic fox illustration.
Logo | Designer | Color | Concept |
---|---|---|---|
![]() |
Neural Network | Green, Blue | Cryptocurrency, Innovation |
ZenNest
ZenNest is a tranquil neural network-generated logo that symbolizes a peaceful retreat nestled within nature. The use of soft pastel colors, organic shapes, and a delicate bird illustration creates a sense of serenity and balance.
Logo | Designer | Color | Concept |
---|---|---|---|
![]() |
Neural Network | Pastel | Tranquility, Nature |
RoboSmart
RoboSmart is a futuristic neural network-designed logo that embodies the precision and technological advancements of robotics. The logo features sleek lines, metallic colors, and an abstract representation of a robotic head, inspiring thoughts of innovation and artificial intelligence.
Logo | Designer | Color | Concept |
---|---|---|---|
![]() |
Neural Network | Silver, Blue | Robotics, Technology |
NatureFusion
NatureFusion is a remarkable logo created by a neural network, blending natural elements with a modern design. The logo showcases a tree merging seamlessly with geometric shapes, symbolizing the harmonious coexistence of nature and human ingenuity.
Logo | Designer | Color | Concept |
---|---|---|---|
![]() |
Neural Network | Green, Grey | Nature, Modernity |
NeoRetro
NeoRetro is a captivating neural network-generated logo combining futuristic aesthetics with a retro twist. With its vibrant neon colors, bold geometric shapes, and a depiction of an old cassette tape, this logo transports us to a bygone era while embracing modern design sensibilities.
Logo | Designer | Color | Concept |
---|---|---|---|
![]() |
Neural Network | Neon | Futuristic, Retro |
SolarSpheres
SolarSpheres is a neural network-generated logo inspired by the captivating beauty of the cosmos. This logo features illuminated spheres, graceful curves, and a cosmic color palette, representing the limitless possibilities and vastness of space.
Logo | Designer | Color | Concept |
---|---|---|---|
![]() |
Neural Network | Purple, Blue | Cosmos, Exploration |
Conclusion
Neural networks have unlocked a new realm of creativity in logo design, producing captivating and visually striking logos that evoke a range of emotions and concepts. From the tranquil ZenNest logo to the electrifying ElectroFlow logo, each neural network-generated logo showcases unique characteristics that captivate and engage viewers. With the advancements in neural network technology, the possibilities for logo design are seemingly limitless, opening doors to new and exciting visual experiences.
Frequently Asked Questions
1. What is a neural network?
A neural network is a computational model inspired by the biological neural networks in the human brain. It is used to recognize complex patterns and make predictions or decisions based on input data.
2. How does a neural network work?
A neural network consists of interconnected artificial neurons, also known as nodes or units. These nodes receive input data, perform calculations using weights and activation functions, and generate output signals. The network learns by adjusting the weights based on the observed errors in its predictions.
3. What is the training process of a neural network?
The training process involves feeding the neural network with a labeled dataset, where the correct outputs are known. The network makes predictions, compares them with the desired outputs, and adjusts its weights to minimize the prediction errors. This iterative process continues until the network achieves satisfactory accuracy.
4. What is the role of activation functions in a neural network?
Activation functions introduce non-linearity to the network, allowing it to learn complex relationships between inputs and outputs. They determine the output of a node based on the weighted sum of its inputs and introduce non-linear transformations that help the network to model and generalize data more effectively.
5. What are the different types of neural networks?
There are various types of neural networks, including feedforward neural networks, recurrent neural networks, convolutional neural networks, and long short-term memory networks. Each type is designed to tackle specific types of problems, such as image recognition, natural language processing, and time-series analysis.
6. Can neural networks handle big data?
Yes, neural networks can handle big data. They have the capability to learn from large datasets with thousands or even millions of samples. However, training neural networks on big data requires substantial computational resources and efficient algorithms to avoid overfitting or underfitting the data.
7. What are the advantages of using neural networks?
Some advantages of using neural networks include their ability to learn from large datasets, handle complex patterns and relationships, make accurate predictions, and adapt to changing conditions. They can also handle noisy or incomplete data and perform well in tasks such as image recognition, speech recognition, and natural language processing.
8. Are there any limitations to neural networks?
Neural networks have some limitations. They require a significant amount of labeled training data to achieve good performance. They can also be computationally expensive, especially when dealing with complex architectures or massive datasets. Additionally, their predictions may lack interpretability, making it challenging to understand the underlying decision-making process.
9. What are some real-world applications of neural networks?
Neural networks find applications in various fields, including but not limited to image and speech recognition, natural language processing, recommendation systems, financial forecasting, autonomous vehicles, medical diagnosis, and drug discovery. They are also used in many AI-powered technologies and systems.
10. What is deep learning, and how is it related to neural networks?
Deep learning is a subfield of machine learning that focuses on training deep neural networks with multiple hidden layers. These deep architectures enable the network to learn hierarchical representations of data, resulting in more accurate and robust predictions. Neural networks form the foundation for deep learning algorithms.