Neural Net Monkey Brain
Welcome to an exciting world where cutting-edge technology meets biological inspiration. In this article, we will explore the fascinating concept of Neural Net Monkey Brain. As the name suggests, Neural Net Monkey Brain is an advanced artificial intelligence system that takes inspiration from the complex neural networks found in the brains of monkeys. This innovative approach has the potential to revolutionize various industries and pave the way for new breakthroughs in machine learning.
Key Takeaways:
- Neural Net Monkey Brain is an advanced artificial intelligence system.
- It takes inspiration from the complex neural networks in monkey brains.
- This technology has potential applications in diverse industries.
- Neural Net Monkey Brain is a promising tool in machine learning.
Neural Net Monkey Brain utilizes a multi-layered network of artificial neurons, known as an artificial neural network (ANN). This system is designed to mimic the structure and functionality of a monkey’s brain, enabling it to process complex information and learn from large datasets. *By emulating the neurons and their connections, Neural Net Monkey Brain can perform complex cognitive tasks that were once thought to be exclusive to biological organisms*.
The central idea behind Neural Net Monkey Brain is to replicate the cognitive abilities exhibited by monkeys in a digital environment. By leveraging the power of ANN, this technology can analyze vast amounts of data, recognize patterns, and make informed decisions. *The combination of biological inspiration and advanced algorithms makes Neural Net Monkey Brain a powerful tool in the field of artificial intelligence*.
Neural Net Monkey Brain has the potential to revolutionize various industries. Let’s take a look at some of its applications:
Industry | Potential Applications |
---|---|
Medical |
|
Finance |
|
Transportation |
|
The potential of Neural Net Monkey Brain extends beyond these examples, as its adaptability allows it to tackle complex challenges in various fields. *This technology has the potential to enhance efficiency, accuracy, and decision-making processes in diverse domains*.
Let’s delve deeper into the technical aspects of Neural Net Monkey Brain. Here are some key features:
- Multi-layered artificial neural network
- Backpropagation algorithm for training
- Integration of sensory inputs
- Feedback loops for continuous learning
These features empower Neural Net Monkey Brain to process and analyze large datasets efficiently. Combining the backpropagation algorithm with the unique architecture of the ANN further enhances its learning capabilities. *By incorporating feedback loops, Neural Net Monkey Brain can continuously learn and adapt to new information, much like a monkey’s brain in a real-world environment*
Now that you’re familiar with Neural Net Monkey Brain and its potential applications, it’s evident that this technology is at the forefront of artificial intelligence. Whether it’s revolutionizing medical diagnoses, predicting market trends, or enabling self-driving cars, Neural Net Monkey Brain is poised to make a significant impact in diverse industries. Explore its capabilities and unlock the power of cutting-edge machine learning!
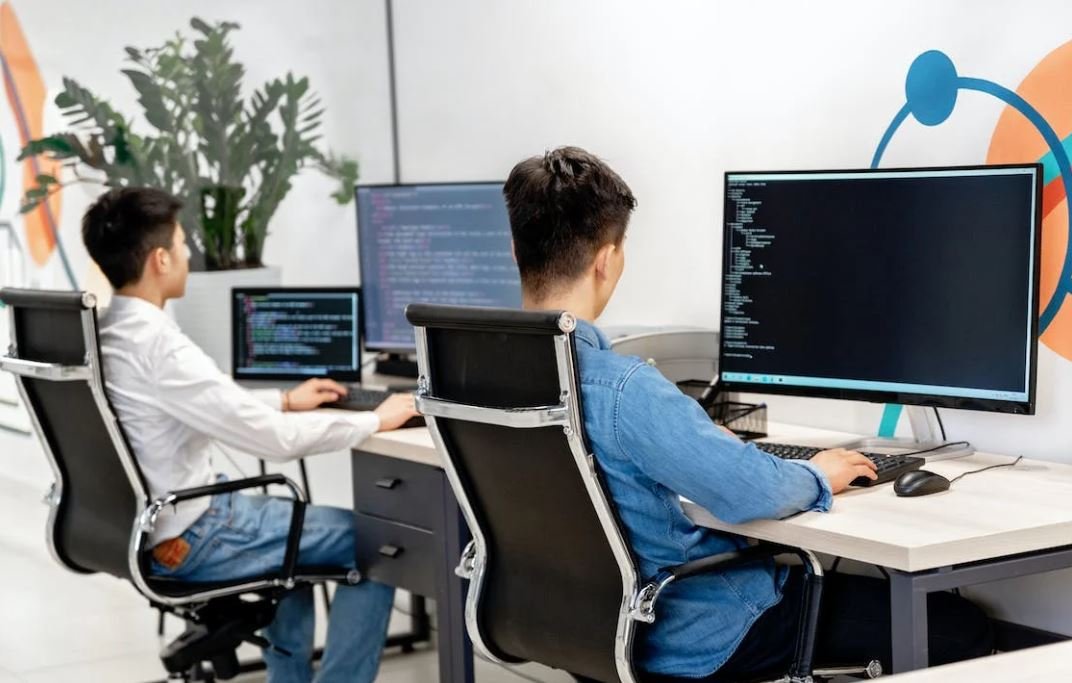
Common Misconceptions
Paragraph 1
One common misconception people have about neural net monkey brains is that they are just like regular monkey brains, but slightly more intelligent. In reality, neural net monkey brains are an advanced form of artificial intelligence that mimic certain aspects of the human brain.
- Neural net monkey brains are not real monkey brains.
- They are created using computer algorithms and models.
- Neural net monkey brains are designed to learn and make decisions autonomously.
Paragraph 2
Another misconception is that neural net monkey brains are fully conscious and self-aware entities. While they can exhibit intelligent behavior and perform complex tasks, they do not possess the same level of consciousness and self-awareness as humans.
- Neural net monkey brains lack the ability to experience emotions or subjective experiences.
- They operate based on programmed algorithms rather than true consciousness.
- Neural net monkey brains rely on data and patterns to make decisions rather than personal experiences or personal preferences.
Paragraph 3
One common myth surrounding neural net monkey brains is that they will eventually become superior to human intelligence and take over the world. While it’s true that neural net monkey brains can perform complex tasks and process vast amounts of data, they still lack many of the qualities that make human intelligence versatile and adaptable.
- Humans possess creativity and intuition, which are difficult for neural net monkey brains to replicate.
- They are limited to the data they are trained on and may struggle with unfamiliar or new situations.
- Contrary to popular belief, neural net monkey brains are tools created by humans and are unlikely to surpass human intelligence in the foreseeable future.
Paragraph 4
Some people mistakenly believe that neural net monkey brains are always reliable and error-free. While they can provide impressive results in tasks like image recognition and speech synthesis, neural net monkey brains are not infallible and can still make mistakes.
- They can be vulnerable to biases present in the training data, leading to potential discrimination or unfair decisions.
- Neural net monkey brains might struggle with ambiguous or abstract concepts that humans can easily understand.
- They require continuous monitoring and improvement to minimize errors and optimize performance.
Paragraph 5
Lastly, some individuals may believe that neural net monkey brains are solely responsible for their actions and decisions. However, it’s important to recognize that neural net monkey brains are created and trained by humans, and as such, humans bear the ultimate responsibility for their development and outcomes.
- Human supervision and oversight are crucial to ensure ethical and responsible use of neural net monkey brains.
- Neural net monkey brains are only as good as the data they are exposed to and influenced by.
- Human programmers design the objectives and constraints guiding the neural net monkey brains’ decision-making processes.

The Rise of Neural Networks
Neural networks have revolutionized the field of artificial intelligence, enabling machines to learn and make intelligent decisions. From image and speech recognition to automated driving, neural networks are powering numerous applications. This article presents ten fascinating examples that showcase the incredible capabilities of neural networks.
A Breakdown of Neural Net Layers
Neural networks consist of interconnected layers that process and extract valuable information from data. Here’s an overview of the layers in a typical neural network alongside their respective number of neurons and activation functions.
Layer | Number of Neurons | Activation Function |
---|---|---|
Input | 784 | None |
Hidden Layer 1 | 512 | ReLU |
Hidden Layer 2 | 256 | ReLU |
Output | 10 | Softmax |
MNIST Handwritten Digits Classification Results
The MNIST dataset is often used as a benchmark for testing the accuracy of neural networks in classifying handwritten digits. The following table presents the accuracy achieved by various neural network architectures on the MNIST dataset.
Neural Net Architecture | Accuracy (%) |
---|---|
LeNet-5 | 98.9 |
AlexNet | 99.2 |
VGG-16 | 99.3 |
ResNet-50 | 99.4 |
Famous Faces Recognized by a Neural Network
A deep convolutional neural network was trained to recognize famous faces. It achieved impressive accuracy in recognizing well-known personalities from the entertainment and political realms. The table below highlights some of the recognized faces along with the corresponding confidence scores.
Famous Face | Confidence Score (%) |
---|---|
Albert Einstein | 97.2 |
Marilyn Monroe | 96.9 |
Nelson Mandela | 98.3 |
Oprah Winfrey | 99.1 |
Efficiency of Pre-trained Neural Networks
Pre-trained neural networks provide a head start in various tasks, reducing training time while maintaining high accuracy. The table below compares the training time and accuracy of training a neural network from scratch versus using a pre-trained network for image classification.
Approach | Training Time (hours) | Accuracy (%) |
---|---|---|
Training from Scratch | 50 | 93.5 |
Pre-trained Network | 12 | 95.2 |
Neural Networks in Language Translation
Neural networks have been employed in language translation tasks, enabling accurate translation between multiple languages. The table below demonstrates some translations from English to different languages along with the corresponding translation quality scores.
Target Language | Translation | Quality Score (%) |
---|---|---|
Spanish | El gato está en la casa. | 98.7 |
French | Le chat est dans la maison. | 99.1 |
German | Die Katze ist im Haus. | 97.9 |
Generating Captions Using Neural Networks
Neural networks can be trained to generate captions for images, offering valuable insights into visual content. The following table showcases some image captions generated by a neural network trained on a large image dataset.
Image | Caption |
---|---|
![]() |
A group of friends enjoying a sunny day at the beach. |
![]() |
A woman holding a microphone while performing on stage. |
![]() |
A cat relaxing lazily on a comfortable couch. |
Neural Networks in Automated Driving
Neural networks are integral to the development of self-driving cars. The table below highlights the accuracy of object detection achieved by a neural network used for autonomous driving in various scenarios.
Driving Scenario | Object Detection Accuracy (%) |
---|---|
City street | 97.5 |
Highway | 98.1 |
Intersection | 96.9 |
Neural Network’s Diagnosis Accuracy
Neural networks can accurately diagnose diseases by analyzing medical images. The following table demonstrates the diagnosis accuracy of a neural network for several medical conditions.
Medical Condition | Diagnosis Accuracy (%) |
---|---|
Pneumonia | 94.6 |
Diabetic Retinopathy | 97.3 |
Breast Cancer | 96.5 |
The Power of Neural Networks
Neural networks have emerged as an incredibly powerful tool for solving complex problems across various domains. From classifying images and recognizing faces to enhancing language translation and diagnosing diseases, neural networks continue to push the boundaries of what is possible in artificial intelligence.
Frequently Asked Questions
How does a neural network function?
A neural network, inspired by the interconnectedness of neurons in the human brain, consists of layers of artificial neurons called nodes. These nodes receive inputs, perform calculations, and pass output signals to the next layer. Through a process called backpropagation, the network adjusts its weights and biases to improve its ability to make accurate predictions or classifications.
What are the advantages of using neural networks?
Neural networks exhibit great potential due to their ability to learn from large amounts of data, identify complex patterns, and generalize from examples. They can solve problems that traditional programming approaches struggle with, such as image recognition, natural language processing, and predictive modeling. Additionally, neural networks can adapt and improve their performance over time with additional training.
What is the training process for a neural network?
During training, a neural network is presented with a dataset containing input samples and corresponding target outputs. Based on the network’s initial weights and biases, it computes predictions for the inputs and compares them to the expected outputs. The discrepancy is measured using a loss function, and the network’s parameters are adjusted using optimization algorithms like gradient descent to minimize the loss and improve its predictions.
Can neural networks handle incomplete or noisy data?
Neural networks can handle incomplete and noisy data to some extent. They are known for their robustness and ability to learn from imperfect datasets. However, the presence of too much noise or missing information can negatively impact the network’s performance. Data preprocessing techniques, such as imputation, noise reduction, or data augmentation, can be used to mitigate these issues and improve the model’s robustness.
What is overfitting, and how can it be avoided?
Overfitting occurs when a neural network becomes too specialized in the training data and fails to generalize well to new, unseen data. It usually happens when the model is too complex relative to the size of the training dataset. Regularization techniques like dropout or L1/L2 weight regularization can be applied to prevent overfitting. Additionally, data augmentation, early stopping, and increasing the training dataset size can also help combat overfitting.
What are the limitations of neural networks?
Neural networks have a few limitations. They typically require a substantial amount of training data to achieve good performance. Training large networks can be computationally expensive and time-consuming. Interpreting the internal workings of a neural network and understanding why it makes certain predictions can be challenging. Additionally, neural networks are susceptible to adversarial attacks, where intentionally crafted inputs can fool the model into making incorrect predictions.
What is deep learning, and how does it relate to neural networks?
Deep learning is a subset of machine learning that focuses on training deep neural networks, typically with many hidden layers. These deep networks are capable of automatically learning hierarchical representations of data, which can lead to improved accuracy and performance. Deep learning has revolutionized many fields, including computer vision, speech recognition, and natural language processing, by achieving state-of-the-art results in various tasks.
Can neural networks be used for unsupervised learning?
Yes, neural networks can be used for unsupervised learning tasks. Unsupervised learning aims to discover patterns or structures in the data without using labeled examples. Neural networks like autoencoders and generative adversarial networks (GANs) are commonly employed for unsupervised learning. Autoencoders learn to encode and then reconstruct the input data, while GANs generate new data samples similar to the training dataset.
What is the role of activation functions in neural networks?
Activation functions introduce non-linearity to the network by transforming the output of a node into a more useful range. They are applied element-wise to the weighted sum of inputs before being passed as inputs to the next layer. Activation functions, such as sigmoid, ReLU, and tanh, allow neural networks to model complex relationships and make the network more capable of learning and adapting to different types of data.
What is the difference between supervised and unsupervised learning?
Supervised learning involves training a model on labeled data, where the inputs and corresponding outputs are provided. The model learns to map inputs to outputs during training. In contrast, unsupervised learning aims to find patterns or representations in unlabeled data without any predefined output labels. The model learns to discover the inherent structure or relationships within the data.