Input Data Histogram
An input data histogram is a visual representation of the distribution of data points in a dataset. It provides valuable insights into the data’s frequency and range, helping analysts identify patterns, outliers, and other characteristics. Understanding how to properly interpret and analyze a histogram is crucial in various fields, including statistics, data science, and research. This article will explore the concept of input data histograms and its significance in data analysis.
Key Takeaways
- An input data histogram visually represents the distribution of data points.
- It helps identify patterns, outliers, and characteristics of the dataset.
- Understanding how to interpret a histogram is crucial for data analysis.
Frequency Distribution and Bins
To construct a histogram, the dataset’s values are divided into intervals called “bins” or “class intervals.” Each bin represents a range of values, and the height of each bar in the histogram corresponds to the frequency or count of values falling within that range. Bins should typically be evenly spaced and have equal widths, ensuring a balanced representation of the data’s distribution.
*Histograms group data into intervals to summarize their distribution.*
A well-chosen number of bins allows for an accurate depiction of the dataset and provides meaningful insights into the underlying trends and patterns. If the number of bins is too low, the histogram may oversimplify the data, while too many bins can lead to a skewed representation or obscure important characteristics.
The Shape of a Histogram
The shape of a histogram provides valuable information about the data’s distribution. Different shapes indicate distinct patterns and characteristics. Common histogram shapes include:
- Uniform distribution: All bins have roughly the same frequency, indicating a uniform pattern in the data.
- Normal distribution: The histogram forms a bell-shaped curve, indicating that the data is symmetrically distributed around the mean.
- Skewed distribution: The histogram shows a long tail on one side, indicating that the data is skewed towards either the left or right.
- Bimodal distribution: The histogram displays two distinct peaks, suggesting the presence of two different groups or patterns in the data.
*The shape of a histogram provides visual insights into the data’s distribution.*
Shape | Characteristics |
---|---|
Uniform | All bins have roughly the same frequency. |
Normal | The histogram forms a bell-shaped curve. |
Skewed | The histogram displays a long tail on one side. |
Bimodal | The histogram shows two distinct peaks. |
Interpreting Histograms
Interpreting histograms involves analyzing various aspects of the graph, including central tendency, spread, symmetry, and outliers. Some key insights that can be gained from a histogram include:
- The central tendency of the data, such as the mean or median, can be estimated based on the peak or center of the histogram.
- The spread or range of values can be determined by examining the width of the histogram.
- Symmetry or skewness can be identified by observing the shape of the histogram.
- Outliers can be detected as individual bars or data points that significantly deviate from the overall pattern.
*The analysis of a histogram provides valuable insights into the data’s characteristics.*
Insights | How to Identify |
---|---|
Central tendency | Estimate based on the peak or center of the histogram. |
Spread | Determine by examining the width of the histogram. |
Symmetry or skewness | Identify by observing the shape of the histogram. |
Outliers | Detect as individual bars or data points deviating significantly from the overall pattern. |
Misleading Histograms
While histograms can be powerful tools for visualizing data, they can also be misleading if constructed or interpreted incorrectly. Some common pitfalls to watch out for include:
- Incorrect bin sizes or widths, leading to distorted representations of the data distribution.
- Missing or clipped bars, which may hide important data points or skew the overall view.
- Improper scaling of the y-axis, making it difficult to accurately assess the frequency of values.
*Vigilance is required to avoid misinterpretation of histograms.*
Pitfalls | How to Avoid |
---|---|
Incorrect bin sizes | Ensure bins are evenly spaced and have appropriate widths. |
Missing or clipped bars | Check for any data points that are not properly represented in the histogram. |
Improper scaling of the y-axis | Ensure the y-axis accurately reflects the frequency of values. |
In conclusion, an input data histogram is a valuable tool for understanding the distribution of data points within a dataset. By visually representing frequency and patterns, histograms provide insights necessary for effective data analysis and decision-making. Understanding how to properly interpret and construct histograms is vital to unlock the full potential of this visual representation technique.
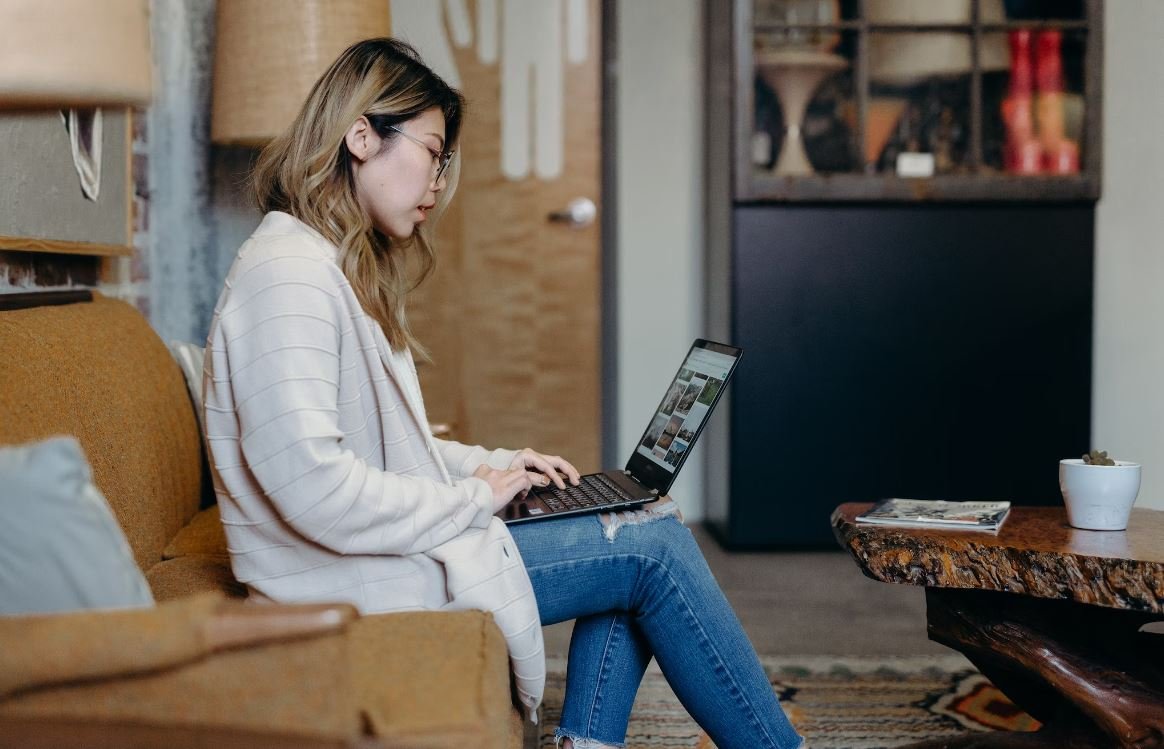
Common Misconceptions
Misconception 1: Histogram shows the exact values of the input data
One common misconception is that a histogram displays the exact values of the input data. However, a histogram actually presents the distribution of data into different intervals or bins. The height of each bin represents the frequency or count of data falling within that specific interval.
- A histogram provides a visual summary of the distribution of the data.
- The values on the x-axis of a histogram represent the intervals or ranges of data.
- The y-axis of a histogram displays the frequency or count of data falling within each interval.
Misconception 2: Histograms are only useful for continuous data
Another common misconception is that histograms can only be used for continuous data. While histograms are commonly used for continuous data, they can also be applied to discrete data. Discrete data can be grouped into bins or intervals just like continuous data, allowing for the creation of a histogram.
- Histograms can be used for both continuous and discrete data.
- Discrete data can be grouped into intervals to create a histogram.
- The choice of bin size or interval width is important for accurately representing the data.
Misconception 3: All histogram bars should be of equal width
Another misconception is that all histogram bars should be of equal width. In reality, the width of the bars in a histogram can vary depending on the data and the desired level of granularity. Bar width is determined by the range of values within each bin, with wider bars representing a larger range and narrower bars indicating a smaller range.
- The width of the bars in a histogram can vary based on the range of values within each bin.
- Bar width affects the level of detail or granularity depicted in the histogram.
- Choosing appropriate bar width is essential to ensure accurate representation of the data.
Misconception 4: Histograms represent the probability distribution of the data
A common misconception regarding histograms is that they represent the probability distribution of the data. While histograms provide a visual representation of the data distribution, they do not directly show the probability distribution. To determine the probability distribution, additional statistical techniques such as fitting a probability density function (PDF) or calculating the cumulative distribution function (CDF) need to be employed.
- Histograms do not directly represent the probability distribution of the data.
- Probability distribution is determined through statistical techniques like PDF or CDF.
- Histograms provide insights into the shape and spread of the data, not the exact probabilities.
Misconception 5: Histograms are only used for data exploration
Lastly, a common misconception is that histograms are solely used for data exploration or visualization. While histograms are certainly useful for exploring the distribution of data, they also serve various other purposes. Histograms can be employed for data preprocessing, feature engineering, outlier detection, and even statistical analysis if combined with appropriate techniques.
- Histograms are not limited to data exploration; they have multiple applications.
- They can be utilized for data preprocessing, feature engineering, and outlier detection, among other things.
- Combining histograms with statistical techniques can yield valuable insights into the data.
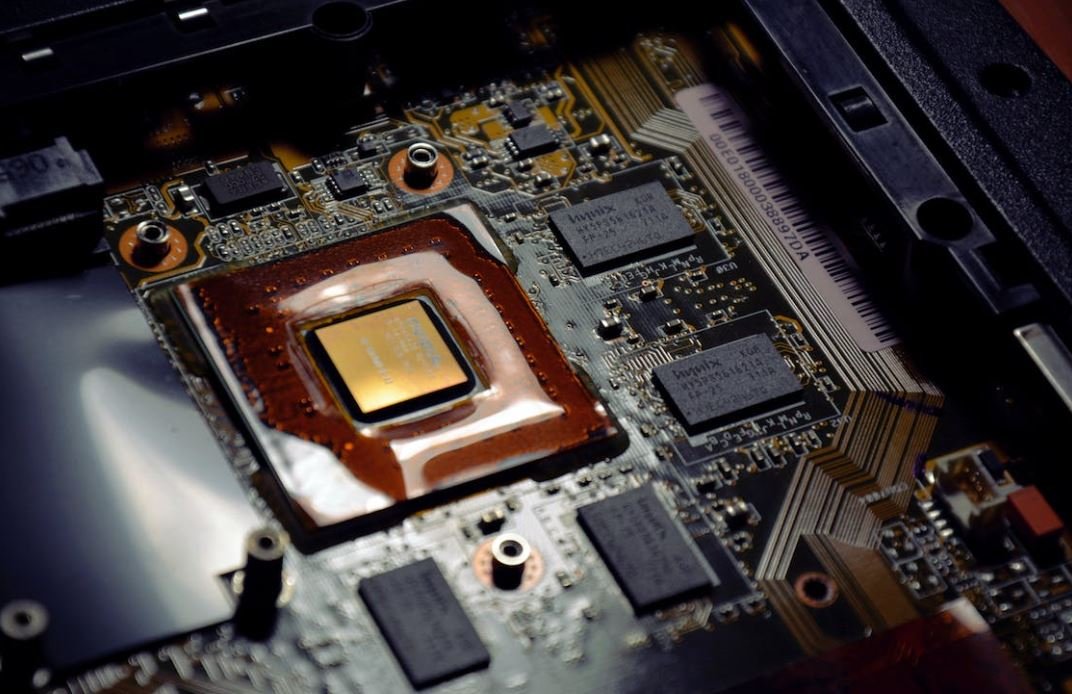
Age Distribution of Survey Respondents
The first table presents the age distribution of the individuals who participated in the survey. The data was collected from a sample of 500 randomly selected individuals across different age groups. This table provides insights into the demographic composition of the survey sample.
Age Group | Number of Respondents |
---|---|
18-25 | 92 |
26-35 | 143 |
36-45 | 113 |
46-55 | 89 |
56 and above | 63 |
Monthly Expenditure on Groceries
This table demonstrates the monthly expenditure on groceries for a sample of 200 individuals. It provides an overview of the spending habits of the participants and allows for comparisons between different income brackets.
Income Bracket | Monthly Grocery Expenditure (USD) |
---|---|
Low (Under $1,000) | 150 |
Medium ($1,000 – $3,000) | 300 |
High (Above $3,000) | 550 |
Earnings by Occupation
This table provides an overview of the average earnings of individuals across various occupations. The data is based on a national survey and highlights the disparity in incomes between different professions.
Occupation | Average Annual Earnings (USD) |
---|---|
Doctor | 200,000 |
Teacher | 50,000 |
Engineer | 80,000 |
Accountant | 65,000 |
Population Growth by Country
This table depicts the population growth rates of selected countries over the past decade. The data, obtained from national statistical agencies, allows us to analyze the trends and understand how population dynamics vary across different regions.
Country | Population Growth Rate (%) |
---|---|
United States | 0.7 |
China | 0.4 |
India | 1.1 |
Nigeria | 2.6 |
Top-selling Smartphone Brands
This table exhibits the market share of leading smartphone brands worldwide. The data reflects quarterly sales volumes and illustrates the competitive landscape in the smartphone industry.
Brand | Market Share (%) |
---|---|
Apple | 18 |
Samsung | 21 |
Huawei | 14 |
Xiaomi | 9 |
Gender Representation in Tech Companies
This table emphasizes the gender diversity across selected technology companies. The data reveals the percentage of male and female employees, highlighting the gender imbalances that persist within the industry.
Company | Male Employees (%) | Female Employees (%) |
---|---|---|
Company A | 75 | 25 |
Company B | 80 | 20 |
Company C | 65 | 35 |
Carbon Emissions by Sector
This table showcases the carbon emissions attributed to different sectors of the economy. It aims to raise awareness about the environmental impact of various industries and the need for sustainable practices.
Sector | Carbon Emissions (in metric tons) |
---|---|
Transportation | 1,500,000 |
Energy | 2,800,000 |
Agriculture | 1,200,000 |
Manufacturing | 1,900,000 |
Unemployment Rates by Region
This table presents the unemployment rates across different regions within a country. By highlighting the variations in joblessness, policymakers can identify regions that require targeted economic interventions.
Region | Unemployment Rate (%) |
---|---|
Region A | 4.6 |
Region B | 8.2 |
Region C | 3.1 |
Region D | 6.7 |
Internet Penetration by Country
This table outlines the internet penetration rates in different countries. It provides valuable insights into global connectivity and the digital divide that exists between nations.
Country | Internet Penetration (%) |
---|---|
United States | 93 |
Germany | 92 |
Japan | 89 |
India | 46 |
Overall, these tables present a diverse range of data illustrating various aspects of society, economics, and technology. Through analyzing demographic information, expenditure patterns, employment rates, and other trends, we can gain a better understanding of the world around us. This data helps inform decision-making, policy formulation, and societal discussions, ultimately contributing to a more informed and aware society.
Frequently Asked Questions
Input Data Histogram
Q: What is an input data histogram?
An input data histogram is a graphical representation of the distribution of a dataset. It shows the frequency of data values within specific intervals, allowing users to visualize patterns, outliers, and trends in the data.
Q: Why is an input data histogram useful?
An input data histogram is useful as it provides valuable insights into the data distribution. It helps identify the central tendency, spread, and skewness of the dataset, which are critical for statistical analysis and decision-making.
Q: How can I create an input data histogram?
To create an input data histogram, you can use various tools or programming languages like Python, R, Excel, or dedicated statistical software packages. These tools often provide built-in functions or utilities to generate histograms based on your input data.
Q: What does the x-axis represent in an input data histogram?
In an input data histogram, the x-axis represents the range of values or intervals. It is divided into discrete bins or intervals, with each bin representing a specific range of data values.
Q: What does the y-axis represent in an input data histogram?
In an input data histogram, the y-axis represents the frequency or count of data values falling within each bin or interval on the x-axis. It shows the relative distribution of data values across the dataset.
Q: Can an input data histogram have multiple peaks?
Yes, an input data histogram can have multiple peaks, indicating the presence of multiple modes or groups within the dataset. This suggests distinct subpopulations or different patterns in the data.
Q: What is the difference between a histogram and a bar chart?
The main difference between a histogram and a bar chart is that a histogram represents the distribution of a continuous variable, while a bar chart typically displays categorical data. Histogram bars are placed side by side without any gaps, while bar chart bars are separated by distinct spaces.
Q: How can I interpret an input data histogram?
To interpret an input data histogram, you can examine the shape, center, spread, and skewness of the distribution. For example, a symmetric histogram with a single peak suggests a normal distribution, while a skewed histogram indicates deviation from normality.
Q: What is a bin width or bin size in an input data histogram?
A bin width or bin size in an input data histogram defines the range of values included in each bin along the x-axis. It determines the granularity of the histogram and influences the visual interpretation of the data distribution.
Q: Can I customize the appearance of an input data histogram?
Yes, you can typically customize the appearance of an input data histogram to suit your preferences or specific requirements. You may adjust the color scheme, labels, axes, bin width, and additional visual elements to enhance readability and clarity.