How Deep Learning Can Change the World
Deep learning, a subset of artificial intelligence (AI), has gained significant attention and is revolutionizing various industries. This advanced technology is enabling computers to learn and make decisions just like humans do, opening up incredible possibilities for a wide range of applications.
Key Takeaways:
- Deep learning is a subset of AI that mimics human intelligence and is transforming multiple industries.
- It allows computers to learn from data and make complex decisions on their own.
- Deep learning has the potential to revolutionize healthcare, transportation, finance, and other sectors.
- Its applications include image recognition, natural language processing, and predictive analytics.
**Deep learning** utilizes artificial neural networks to analyze vast amounts of data and extract meaningful patterns. These networks are inspired by the structure and function of the human brain, comprising interconnected layers of nodes that process and transmit information. One of the remarkable qualities of deep learning is its ability to automatically identify relevant features from raw data, eliminating the need for explicit programming.
*Deep learning has gained prominence in recent years due to its exceptional performance in various tasks.* It has surpassed traditional machine learning algorithms by achieving state-of-the-art results in fields such as image recognition, speech recognition, and natural language processing. Its **unsupervised learning** capabilities allow it to discover patterns and relationships in data without the need for explicit labels, making it an incredibly versatile technology.
Revolutionizing Industries with Deep Learning
Deep learning has the potential to disrupt and transform numerous industries, revolutionizing the way we live and work. Let’s explore how it can shape the future in key sectors:
1. Healthcare
In the healthcare industry, deep learning is being used to improve diagnosis and treatment outcomes. By analyzing medical images, deep learning algorithms can detect diseases at an early stage, potentially saving lives. *With deep learning, medical professionals can tap into a vast array of data-driven insights to make faster and more accurate decisions.*
2. Transportation
*Self-driving cars are a prime example of deep learning’s impact on the transportation industry.* Deep learning algorithms help autonomous vehicles perceive and interpret their surroundings by analyzing sensor data, enabling them to navigate and make intelligent decisions on the road. This innovation has the potential to reduce accidents and congestion while increasing transportation efficiency.
3. Finance
Financial institutions are leveraging deep learning to enhance fraud detection, risk assessment, and trading strategies. Deep learning models can analyze large volumes of financial data in real-time, identifying patterns indicative of fraudulent activities. *This automation enables quicker fraud detection, protecting individuals and organizations from financial losses.*
The Power of Deep Learning in Applications
Deep learning finds its application in various areas, bringing significant advancements and transformative potential:
1. Image Recognition
*Deep learning has revolutionized image recognition by achieving near-human performance.* It can accurately identify objects, faces, and gestures, opening up possibilities in security systems, autonomous vehicles, and medical diagnostics.
2. Natural Language Processing
*Natural language processing, a subset of deep learning, enables computers to understand, interpret, and generate human language.* It allows for virtual assistants, chatbots, and automated language translation services, enhancing communication and accessibility.
3. Predictive Analytics
Deep learning excels in predictive analytics by predicting outcomes and trends based on historical data. It can assist businesses in making data-driven decisions, optimizing processes, and improving customer experiences.
Data on Deep Learning’s Impact
Industry | Data Points |
---|---|
Healthcare | Deep learning has achieved high accuracy rates in diagnosing diseases from medical images, reducing the need for invasive procedures. |
Transportation | Self-driving cars using deep learning algorithms have shown promising results in reducing accidents and enhancing road safety. |
Finance | Deep learning models have significantly improved fraud detection rates in the financial industry, safeguarding individuals and institutions. |
The Future of Deep Learning
As deep learning continues to evolve and advance, we can expect even more significant breakthroughs and applications in the future. With its ability to process vast amounts of data swiftly and accurately, deep learning has the potential to shape the world we live in, enhancing various industries and improving our daily lives.
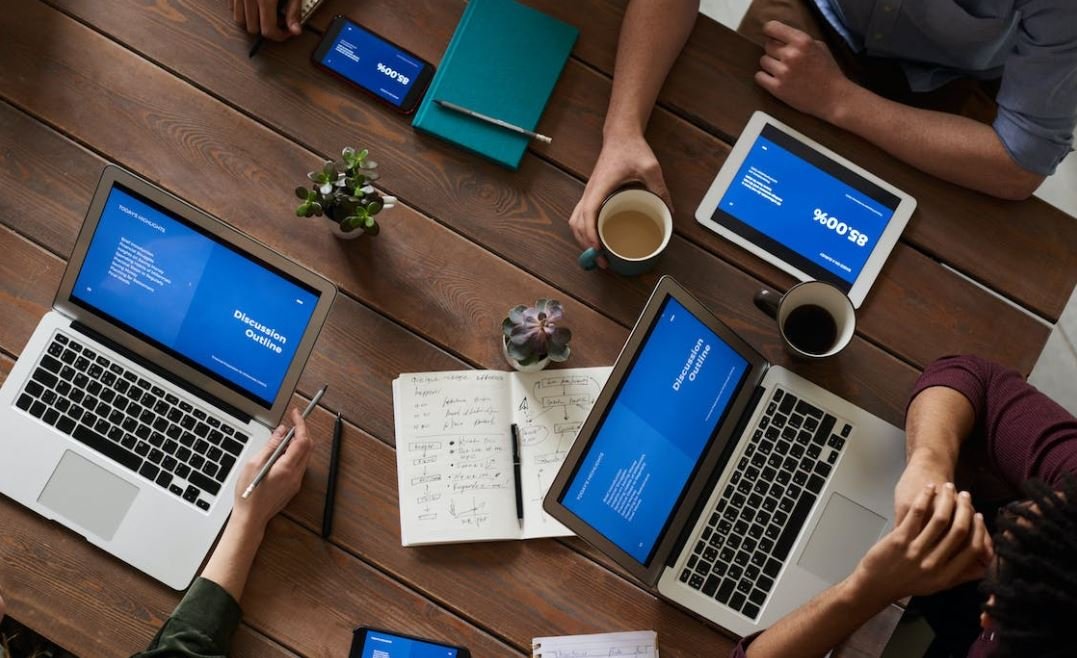
Common Misconceptions
Misconception 1: Deep learning is the same as artificial intelligence (AI)
One common misconception is that deep learning and artificial intelligence (AI) are interchangeable terms. While deep learning is a subfield of AI, it is important to note that AI encompasses a broader range of techniques and technologies. Deep learning specifically refers to a type of machine learning algorithm that uses artificial neural networks to learn and make predictions.
- Deep learning is a subset of AI
- Deep learning focuses on neural networks
- AI includes various other techniques and technologies
Misconception 2: Deep learning can replace human judgment and decision-making
Another misconception is that deep learning can completely replace human judgment and decision-making. While deep learning algorithms can perform complex tasks and automate certain processes, they are limited to the patterns and data they have been trained on. Humans possess cognitive abilities that enable them to understand context, make ethical decisions, and exercise empathy, which cannot be fully replicated by AI technologies like deep learning.
- Deep learning can automate certain tasks
- Humans possess cognitive abilities not replicated in deep learning
- Deep learning is limited to patterns and data it has been trained on
Misconception 3: Deep learning is infallible and error-free
Some people believe that deep learning algorithms are infallible and error-free. However, like any technology, deep learning algorithms are prone to errors and face limitations. These algorithms heavily rely on the quality and quantity of training data, and can produce inaccurate results or biased outcomes if the training data is flawed or insufficient. Ongoing human supervision and continuous improvement are crucial to ensure the accuracy and reliability of deep learning models.
- Deep learning algorithms can produce errors
- Quality and quantity of training data affects outcomes
- Ongoing human supervision is essential
Misconception 4: Deep learning has no ethical implications
Many people assume that deep learning has no ethical implications. However, the use of deep learning algorithms in various domains raises significant ethical concerns. AI systems can inadvertently perpetuate existing biases present in the training data, leading to discriminatory outcomes. Additionally, the use of deep learning in sensitive areas such as healthcare and criminal justice requires careful consideration of privacy, security, and accountability to ensure fair and ethical outcomes.
- Deep learning can perpetuate biases
- Ethical implications in sensitive domains like healthcare and criminal justice
- Privacy, security, and accountability considerations are necessary
Misconception 5: Deep learning will lead to widespread job loss
One of the most prevalent misconceptions is that deep learning will result in widespread job loss and unemployment. While some repetitive and mundane tasks can be automated through deep learning, this technology also creates new job opportunities. Deep learning requires skilled professionals who can design, implement, and maintain complex neural networks. It also enables the development of innovative applications and new business models that can drive economic growth and create employment opportunities.
- Deep learning automates repetitive tasks but also creates new jobs
- Skilled professionals are needed to work with deep learning
- Deep learning drives innovation and job creation
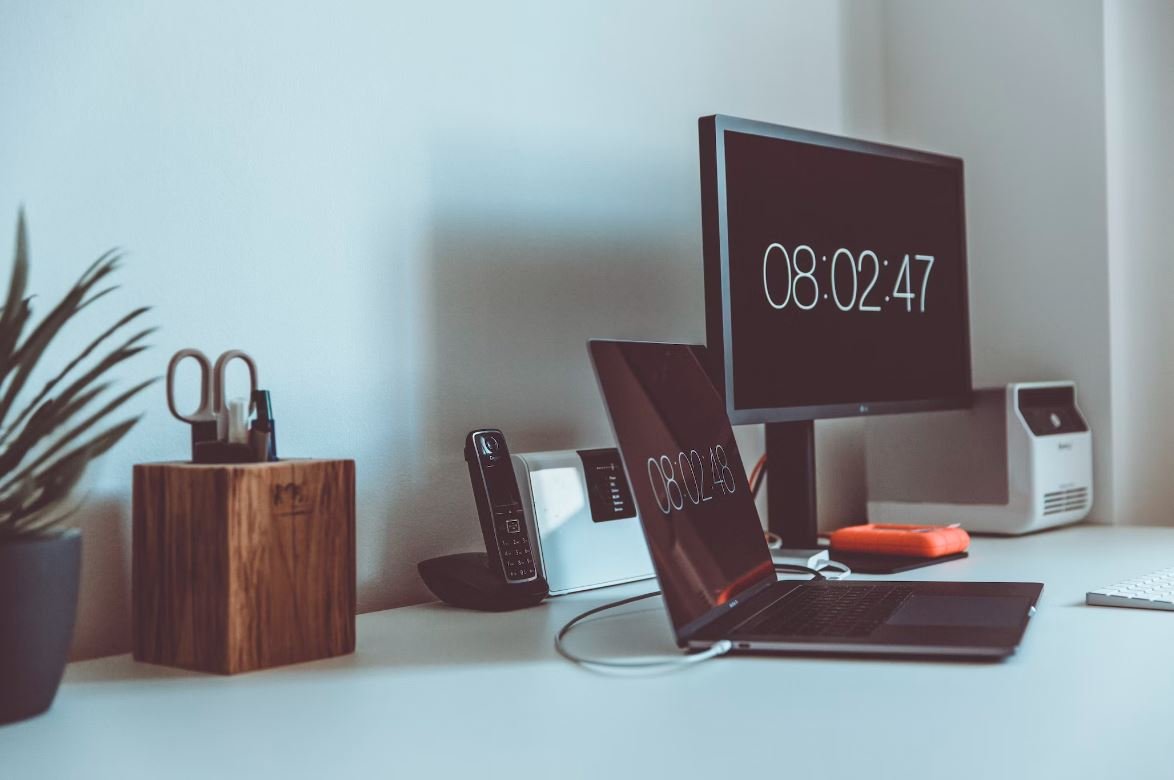
Artificial Intelligence Statistics in 2021
The following table presents some key statistics related to artificial intelligence and deep learning in 2021. These numbers demonstrate the rapid growth and potential impact of deep learning on various industries.
Statistic | Value |
---|---|
Global AI market size | $62.35 billion |
Number of AI startups | 8,705 |
AI-related job postings | 1.2 million |
Percentage of enterprises using AI | 37% |
Annual growth in AI investment | 44.3% |
Applications of Deep Learning in Healthcare
This table highlights several applications of deep learning in the field of healthcare. These technologies have the potential to revolutionize medical diagnostics and treatment.
Application | Example |
---|---|
Medical imaging analysis | AI-assisted diagnosis of lung cancer |
Drug discovery | AI-based identification of novel drug candidates |
Disease prediction | Early detection of Alzheimer’s disease |
Remote patient monitoring | Real-time analysis of vital signs |
Robot-assisted surgeries | Precision robotic surgical systems |
Deep Learning in Autonomous Vehicles
This table explores the integration of deep learning techniques in autonomous vehicles, enabling them to make intelligent decisions based on real-time data.
Technology | Description |
---|---|
Computer vision | Object detection, lane tracking, and traffic sign recognition |
Natural language processing | Understanding voice commands and conversational interactions |
Machine learning algorithms | Optimizing driving behavior based on road conditions |
Sensor fusion | Combining data from cameras, lidar, radar, and ultrasonic sensors |
Deep reinforcement learning | Continuous learning and decision-making in dynamic environments |
Deep Learning in Finance
This table elucidates the utilization of deep learning methods in the finance industry, providing advanced data analysis and decision-making capabilities.
Application | Advantage |
---|---|
Fraud detection | Identification of suspicious patterns and anomalies in transactions |
Algorithmic trading | Automated trading strategies based on large-scale data analysis |
Credit risk assessment | Improved accuracy in predicting creditworthiness |
Portfolio management | Optimization of investment portfolios based on market trends |
Natural language processing for news analysis | Extraction of sentiment and market-impacting information from news |
Ethical Considerations in Deep Learning
This table sheds light on some ethical considerations that must be taken into account when developing and deploying deep learning systems.
Concern | Explanation |
---|---|
Data bias | Ensuring fairness and preventing discrimination in data-driven decisions |
Privacy | Safeguarding personal information from unauthorized access |
Job displacement | Addressing the potential impact of automation on employment |
Transparency | Making deep learning processes and decisions interpretable |
Accountability | Defining responsibility and liability for AI system errors or accidents |
Deep Learning in Image Recognition
This table showcases the remarkable advancements in deep learning for image recognition tasks, demonstrating the ability to accurately classify and detect objects within images.
Application | Accuracy |
---|---|
Image classification | Top-1 error rate reduced to 4.9% |
Object detection | Multiscale detection with an average precision of 81.5% |
Facial recognition | Recognition rate surpassing human-level performance |
Scene understanding | Accurate identification of complex scenes and objects within |
Image generation | Creating realistic and novel images based on learned patterns |
Deep Learning in Natural Language Processing
This table demonstrates the significant progress made in natural language processing tasks utilizing deep learning models, enabling better understanding and generation of human language.
Task | Advancement |
---|---|
Machine translation | Reducing translation errors across multiple languages |
Sentiment analysis | Accurate classification of textual sentiment and emotions |
Speech recognition | Improved accuracy in transcribing spoken language |
Question answering | Efficient retrieval of answers from large knowledge bases |
Text summarization | Generating concise summaries from lengthy articles |
Deep Learning in E-commerce
This table exemplifies the various ways deep learning is advancing the e-commerce industry, enhancing user experience, and improving customer satisfaction.
Application | Benefit |
---|---|
Product recommendation | Personalized recommendations leading to increased sales |
User behavior analysis | Understanding and predicting customer preferences and actions |
Visual search | Searching for similar products based on images |
Dynamic pricing | Optimizing prices based on demand and market trends |
Chatbots | Efficient customer service and support |
Deep Learning Limitations
This table sheds light on some limitations and challenges faced by deep learning algorithms, highlighting areas that require further research and development.
Challenge | Description |
---|---|
Data requirements | Large amounts of labeled data needed for training |
Interpretability | Understanding the reasoning behind decisions made by models |
Adversarial attacks | Vulnerability to manipulated inputs leading to incorrect outputs |
Generalization | Ensuring models perform well in diverse real-world scenarios |
Computational resources | High computational power required for training and inference |
Deep learning, a subset of artificial intelligence, has the potential to revolutionize numerous industries. The tables presented above illustrate the wide-ranging applications and advancements of deep learning in healthcare, autonomous vehicles, finance, image recognition, natural language processing, e-commerce, while also addressing ethical considerations and limitations. These technologies have already made significant progress, showcasing remarkable accuracy and potential to transform our lives. However, further research, collaboration, and addressing challenges will be vital to fully unlock the potential of deep learning and shape a future where it positively influences various aspects of our world.
Frequently Asked Questions
How Deep Learning Can Change the World
Question 1
What is deep learning?
Question 2
How does deep learning work?
Question 3
What are the applications of deep learning?
Question 4
How can deep learning change the world?
Question 5
What are the challenges of deep learning?
Question 6
Is deep learning the same as artificial intelligence?
Question 7
What data is required for deep learning?
Question 8
What skills are needed to work in deep learning?
Question 9
Are there any ethical considerations with deep learning?
Question 10
Can deep learning be used for cybersecurity?