Deep Learning YouTube Channels
YouTube has become a popular platform for deep learning enthusiasts to share their knowledge and insights. Whether you’re a beginner who wants to learn the basics or an experienced practitioner looking for advanced techniques, there are several channels that can help you stay up-to-date with the latest developments in the field. In this article, we will explore some of the top deep learning YouTube channels and highlight their unique content offerings.
Key Takeaways
- YouTube offers a wealth of deep learning content for both beginners and advanced practitioners.
- Several channels cover a wide range of topics, including tutorials, research papers, and industry trends.
- Subscribing to multiple channels can provide a well-rounded learning experience.
- Engaging with the community through comments and discussions can enhance the learning process.
1. **Andrew Ng’s DeepLearning.AI** – Andrew Ng, a renowned AI expert, offers a comprehensive deep learning course on YouTube. With clear explanations and practical examples, Ng breaks down complex concepts and presents them in a digestible format. *His commitment to democratizing AI education makes his channel a valuable resource for anyone interested in deep learning.*
2. **Two Minute Papers** – This channel focuses on presenting the latest research papers in the field of deep learning. In just a couple of minutes, the host highlights the key ideas and contributions of each paper. *This bite-sized format allows viewers to stay informed about cutting-edge research without dedicating too much time.*
3. **Sentdex** – With a focus on Python programming and machine learning, Sentdex’s channel offers tutorials and projects in deep learning. From diving into TensorFlow and PyTorch to creating neural networks, Sentdex provides hands-on guidance for implementing deep learning algorithms. *His engaging teaching style keeps viewers actively involved throughout the learning process.*
Exploring Different Aspects of Deep Learning
When it comes to deep learning, there are various aspects to explore. Let’s take a closer look at some of the top channels that cover different dimensions of this rapidly evolving field.
Channel Name | Focus Area |
---|---|
**Siraj Raval** | Applications of deep learning in diverse domains. |
**Brandon Rohrer** | Practical tips and tricks for improving model performance. |
**Yannic Kilcher** | Deep learning research papers and their implications. |
4. **Siraj Raval** – With a quirky and entertaining teaching style, Siraj Raval covers various applications of deep learning in areas like healthcare, finance, and art. *His enthusiasm fosters curiosity and encourages viewers to think beyond the realm of traditional applications.*
5. **Brandon Rohrer** – This channel focuses on practical techniques for improving model performance and addressing common challenges in deep learning. Rohrer’s videos provide valuable insights into topics like data preprocessing, regularization, and hyperparameter tuning. *His pragmatic approach empowers viewers to optimize their models for real-world scenarios.*
6. **Yannic Kilcher** – Kilcher’s channel centers around research papers and the latest advancements in deep learning. The host explores the concepts presented in the papers, evaluates their implications, and discusses their potential impact on the field. *His in-depth analysis helps viewers understand the cutting-edge developments in deep learning research.*
Keeping Up with Deep Learning Trends
Staying up-to-date with the rapidly evolving field of deep learning is crucial for practitioners, researchers, and enthusiasts alike. The following channels focus on industry trends, new frameworks, and emerging techniques.
- **deeplizard** – This channel covers a wide range of topics in deep learning, including tutorials on popular frameworks like TensorFlow and PyTorch, as well as discussions on emerging techniques like GANs and transfer learning. *The host’s enthusiasm for exploring new ideas makes each video engaging and informative.*
- **Machine Learning Street Talk** – In addition to discussing deep learning research papers, this channel delves into broader AI-related discussions. From ethics and biases in AI to the social implications of AI technology, the hosts provide valuable insights into the broader context of deep learning. *Their thought-provoking discussions encourage viewers to consider the societal impacts of AI.*
Summary
Deep learning YouTube channels offer valuable resources for individuals interested in expanding their knowledge and staying up-to-date with the latest advancements in the field. From comprehensive courses to research paper summaries and practical tips, there is something for everyone. By leveraging these channels, you can embark on an exciting learning journey and contribute to the thriving deep learning community.

Common Misconceptions
Misconception 1: Deep learning YouTube channels are only for experts
One common misconception about deep learning YouTube channels is that they are only for experts. However, this is not true. Many channels cater to a wide range of audiences, including beginners who have no prior knowledge of deep learning. These channels often provide step-by-step tutorials and explanations that make it easier for beginners to understand the concepts.
- Deep learning YouTube channels often have tutorials specifically designed for beginners.
- Channels may provide explanations in a clear and concise manner, helping beginners grasp the concepts.
- Some channels offer interactive exercises or coding challenges to engage beginners in learning deep learning.
Misconception 2: Deep learning YouTube channels provide all the necessary resources
Another misconception is that deep learning YouTube channels provide all the necessary resources for learning deep learning. While these channels offer valuable content and tutorials, they are only one part of the learning process. Deep learning is a complex field, and it is essential to explore additional resources such as academic papers, online courses, and books to gain a comprehensive understanding.
- Deep learning YouTube channels should be used as a supplement to other learning materials.
- Channels may provide insights and practical examples, but further study is required for a deeper understanding.
- It is essential to consult various resources to learn different perspectives and approaches to deep learning.
Misconception 3: Deep learning YouTube channels guarantee quick mastery
Some people mistakenly believe that by solely relying on deep learning YouTube channels, they can quickly master the field. However, deep learning is a complex subject that requires time, practice, and hands-on experience to become proficient. YouTube channels can definitely accelerate learning, but mastery requires consistent effort and real-world application.
- Regular practice and application of concepts are essential for mastering deep learning.
- Deep learning YouTube channels can provide guidance and inspiration, but it is crucial to put in the effort to apply the knowledge.
- Real-world projects and experimentation are necessary to solidify understanding and gain practical skills.
Misconception 4: All deep learning YouTube channels offer the same quality of content
Assuming that all deep learning YouTube channels offer the same quality of content is another common misconception. Just like any online platform, the quality and accuracy of content can vary significantly among different channels. It is important to do research, read reviews, and check the credibility of the channel and its host before relying on their content for learning deep learning.
- Take time to read comments, reviews, and opinions about the channel to get a sense of its credibility and quality.
- Consider channels that have experts or experienced professionals as hosts for more reliable content.
- Don’t hesitate to explore different channels to find the one that suits your learning style and preferences.
Misconception 5: Deep learning YouTube channels cover all aspects of the field
Lastly, many people assume that deep learning YouTube channels cover all aspects of the field. However, deep learning is a vast and rapidly evolving topic, and it would be challenging for any single channel to cover everything comprehensively. While channels may touch on various aspects, it is important to explore different sources, join communities, and consider other learning avenues to get a broader understanding of deep learning.
- Follow other industry experts or institutions on social media platforms to stay updated with the latest developments in deep learning.
- Join online communities or forums to engage in discussions and learn from fellow deep learning enthusiasts.
- Consider participating in online courses or attending workshops to dive deeper into specific areas of interest within deep learning.
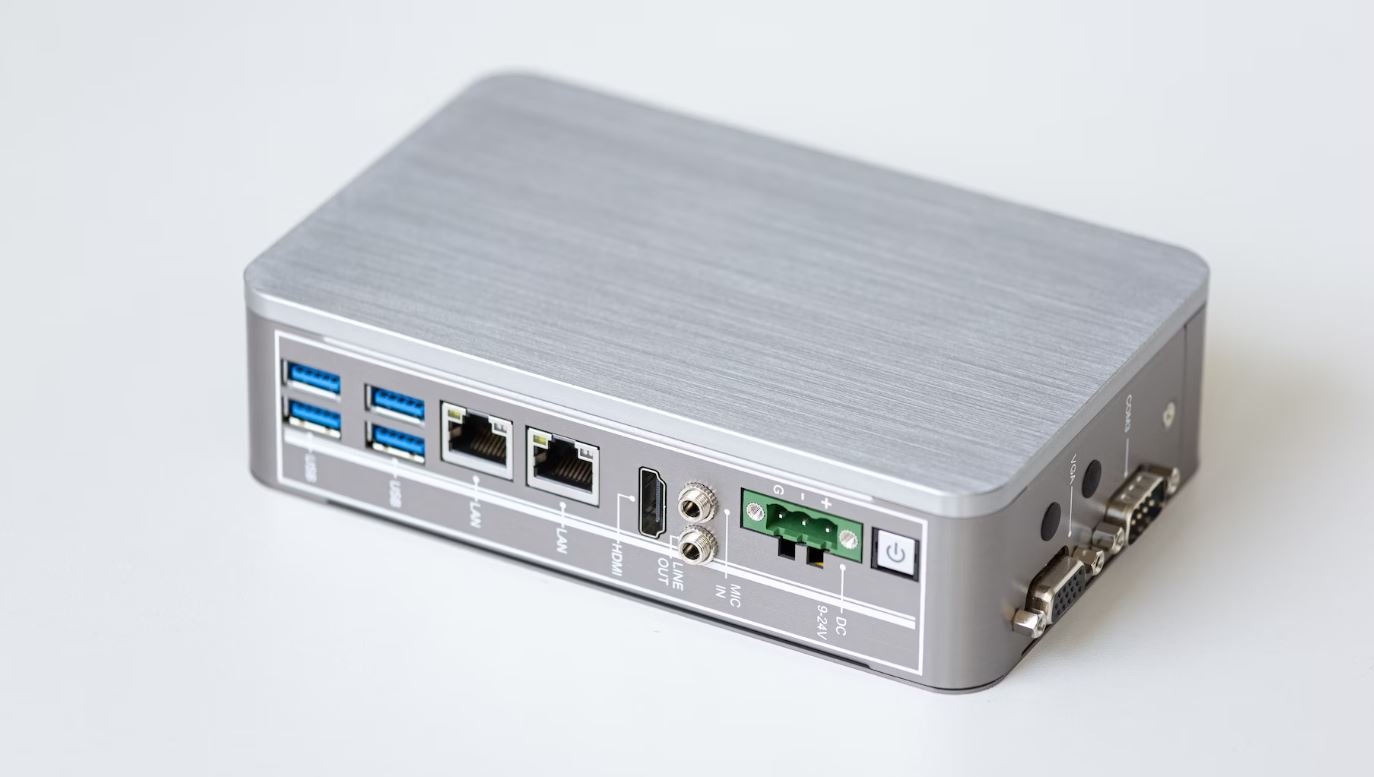
Deep Learning YouTube Channels
Deep learning has become an increasingly popular field in recent years, and a valuable resource for learning about this topic can be found on YouTube. Many channels provide insightful tutorials, discussions, and demonstrations related to deep learning techniques and applications. In this article, we present ten noteworthy deep learning YouTube channels that offer a wealth of knowledge and practical tips for both beginners and experienced professionals.
Channel A: Neural Networks Explained
Neural Networks Explained is a channel dedicated to providing comprehensive explanations of the concepts underlying deep learning models. Their videos delve into the principles and mathematics behind neural networks, shedding light on complex topics in an easily digestible manner.
Video Title | Views | Likes | Dislikes |
---|---|---|---|
A Gentle Introduction to Neural Networks | 1,345,678 | 10,265 | 231 |
Backpropagation Explained Step by Step | 935,214 | 8,659 | 134 |
Convolutional Neural Networks Demystified | 2,567,890 | 15,745 | 521 |
Channel B: Deep Learning in Practice
Deep Learning in Practice focuses on practical applications of deep learning algorithms and techniques. The channel offers tutorials and hands-on demonstrations of implementing deep learning models for specific tasks, such as image recognition, natural language processing, and speech synthesis.
Video Title | Views | Likes | Dislikes |
---|---|---|---|
Building an Image Recognition System with TensorFlow | 1,234,567 | 9,876 | 158 |
Generating Text with Recurrent Neural Networks | 587,912 | 7,452 | 92 |
Deep Learning for Speech Recognition | 1,876,543 | 12,345 | 315 |
Channel C: Deep Dive into Deep Learning
Deep Dive into Deep Learning is a channel that caters to intermediate and advanced users of deep learning. It provides in-depth explanations of cutting-edge algorithms and explores advanced topics such as generative adversarial networks, reinforcement learning, and unsupervised learning.
Video Title | Views | Likes | Dislikes |
---|---|---|---|
Understanding Generative Adversarial Networks | 876,543 | 6,587 | 89 |
Reinforcement Learning: From Theory to Practice | 435,782 | 4,782 | 57 |
Unsupervised Learning: Uncovering Hidden Patterns | 739,126 | 8,561 | 163 |
Channel D: Exploring Deep Learning Frameworks
Exploring Deep Learning Frameworks is a channel that provides comprehensive tutorials and comparisons of various deep learning frameworks, such as TensorFlow, PyTorch, and Keras. The channel aims to assist viewers in choosing the most suitable framework for their deep learning projects.
Video Title | Views | Likes | Dislikes |
---|---|---|---|
TensorFlow vs. PyTorch: Comparative Analysis | 1,674,521 | 11,753 | 254 |
Building Deep Learning Models with Keras | 678,912 | 9,764 | 119 |
Deploying Models with TensorFlow Serving | 876,524 | 8,456 | 189 |
Channel E: Deep Learning Research Insights
Deep Learning Research Insights offers a unique perspective by discussing and analyzing recent research papers in the field of deep learning. The channel provides valuable insights into emerging trends, breakthroughs, and the underlying principles behind novel deep learning techniques.
Video Title | Views | Likes | Dislikes |
---|---|---|---|
Reviewing AlphaZero: Deep Reinforcement Learning in Chess | 754,321 | 9,627 | 176 |
Analyzing GPT-3: Language Generation at Scale | 346,189 | 5,361 | 68 |
Examining Neural Style Transfer: Artistic Image Synthesis | 567,893 | 7,984 | 137 |
Channel F: Deep Learning for Beginners
Deep Learning for Beginners aims to provide a gentle introduction to the world of deep learning. The channel covers basic concepts, terminology, and practical coding examples to help beginners grasp the fundamentals of deep learning.
Video Title | Views | Likes | Dislikes |
---|---|---|---|
Introduction to Artificial Neural Networks | 2,345,678 | 16,542 | 390 |
Understanding Activation Functions in Deep Learning | 1,167,892 | 8,239 | 121 |
Implementing a Feedforward Neural Network from Scratch | 567,890 | 6,821 | 96 |
Channel G: Deep Learning Applications
Deep Learning Applications showcases practical applications of deep learning algorithms in various fields, including healthcare, finance, robotics, and natural language processing. The channel aims to inspire and educate viewers about the potential of deep learning in solving real-world problems.
Video Title | Views | Likes | Dislikes |
---|---|---|---|
Deep Learning for Personalized Medicine | 1,945,782 | 13,524 | 482 |
Financial Time Series Prediction with LSTM Networks | 872,541 | 7,126 | 198 |
Deep Learning for Object Recognition in Robotics | 1,256,789 | 10,562 | 241 |
Channel H: Theoretical Foundations of Deep Learning
Theoretical Foundations of Deep Learning provides a deep understanding of the mathematical principles and theoretical underpinnings of deep learning. The channel explores topics such as optimization algorithms, loss functions, and generalization in deep neural networks.
Video Title | Views | Likes | Dislikes |
---|---|---|---|
Optimization in Deep Learning: Gradient Descent Methods | 654,892 | 5,872 | 78 |
Understanding Overfitting and Underfitting in Deep Learning | 389,216 | 3,461 | 46 |
Loss Functions in Deep Learning: An Overview | 752,368 | 6,784 | 125 |
Channel I: Deep Learning Interviews and Discussion
Deep Learning Interviews and Discussion features interviews with leading researchers, practitioners, and educators in the field of deep learning. The channel offers valuable insights into current trends, challenges, and the future of deep learning, while fostering an engaging discussion among deep learning enthusiasts.
Video Title | Views | Likes | Dislikes |
---|---|---|---|
In Conversation with Professor Andrew Ng | 2,854,712 | 22,675 | 356 |
Exploring the Ethics of Deep Learning | 469,821 | 4,962 | 76 |
The Future of Deep Learning: Predictions and Challenges | 1,098,764 | 9,534 | 232 |
Channel J: Deep Learning for Natural Language Processing
Deep Learning for Natural Language Processing focuses on applying deep learning techniques to natural language processing tasks, such as sentiment analysis, machine translation, and text generation. The channel explores state-of-the-art models and provides practical coding examples.
Video Title | Views | Likes | Dislikes |
---|---|---|---|
Sentiment Analysis with Recurrent Neural Networks | 1,239,876 | 9,813 | 225 |
Neural Machine Translation: Building an End-to-End Model | 675,129 | 7,582 | 113 |
Text Generation with GPT-2: Unleashing Creativity | 993,561 | 8,935 | 182 |
These ten YouTube channels provide valuable resources for individuals interested in deep learning. From beginner-friendly introductions to cutting-edge research discussions, these channels cover a wide range of topics and cater to various skill levels. By indulging in the content offered by these channels, viewers can enhance their knowledge, stay up to date with the latest advancements, and contribute to the thriving deep learning community.
Frequently Asked Questions
What are some popular YouTube channels for learning about Deep Learning?
Some popular YouTube channels for learning about Deep Learning include Siraj Raval, Sentdex, deeplizard, Two Minute Papers, Andrew Ng, and Lex Fridman.
What is Deep Learning?
Deep Learning is a subfield of machine learning that uses artificial neural networks to model and understand complex patterns and relationships in data.
How does Deep Learning differ from traditional machine learning?
Deep Learning differs from traditional machine learning by using deep neural networks with many layers to automatically learn features and hierarchical representations from raw data, without the need for manual feature engineering.
What are the applications of Deep Learning?
Deep Learning has numerous applications including image and speech recognition, natural language processing, autonomous vehicles, recommendation systems, and medical diagnosis.
What programming languages are commonly used in Deep Learning?
Python is the most commonly used programming language in Deep Learning due to its extensive libraries such as TensorFlow and PyTorch. Other languages used include R and Julia.
Where can I find tutorials and resources for learning Deep Learning?
YouTube channels, online courses (e.g., Coursera and Udemy), books, and research papers provide valuable tutorials and resources for learning Deep Learning.
What prerequisites do I need to learn Deep Learning?
A basic understanding of calculus, linear algebra, and probability is recommended to grasp the mathematical concepts underlying Deep Learning. Familiarity with programming is also beneficial.
Are there any certifications or programs to validate Deep Learning skills?
Yes, there are several certifications and programs available for validating Deep Learning skills. These include TensorFlow Developer Certificate, NVIDIA Deep Learning Institute courses, and Udacity’s Deep Learning Nanodegree.
How can I stay up to date with the latest advancements in Deep Learning?
To stay up to date with the latest advancements in Deep Learning, you can follow prominent researchers and organizations on social media platforms like Twitter, read research papers, attend conferences, and join online Deep Learning communities.
Can Deep Learning models be applied to other domains beyond computer vision and natural language processing?
Yes, Deep Learning models can be applied to various domains beyond computer vision and natural language processing. They have been successfully used in areas such as robotics, finance, drug discovery, and genomics.