Deep Learning in Healthcare
Deep learning, a branch of artificial intelligence, has been making significant strides in the healthcare industry in recent years. By using large amounts of data and advanced algorithms, deep learning systems can analyze and interpret complex medical data, leading to improved disease diagnosis, treatment planning, and patient outcomes. This article will explore the applications of deep learning in healthcare and discuss its potential impact on the future of medicine.
Key Takeaways:
- Deep learning enhances disease diagnosis and treatment planning.
- Deep learning allows for personalized medicine.
- Deep learning improves patient outcomes and reduces healthcare costs.
One of the key benefits of deep learning in healthcare is its ability to enhance disease diagnosis and treatment planning. Deep learning algorithms can analyze medical images, such as X-rays, CT scans, and MRIs, and accurately detect abnormalities or early signs of diseases. This can significantly improve the efficiency and accuracy of diagnosis, allowing healthcare professionals to provide timely and appropriate treatment. *This technology revolutionizes medical imaging interpretation and reduces errors in diagnostics.*
Another important application of deep learning in healthcare is personalized medicine. By analyzing a patient’s genetic and molecular data, deep learning algorithms can identify patterns and relationships that can help personalize treatment plans. These algorithms can predict a patient’s response to different medications or therapies, allowing healthcare providers to tailor treatments specifically to individual patients. *Personalized medicine holds the promise of more effective and targeted treatments for patients with various medical conditions.*
Table 1: Deep Learning Applications in Healthcare | |
---|---|
Application | Example |
Medical Image Analysis | Automated detection of cancerous cells in pathology slides |
Drug Discovery | Identifying potential drug candidates for specific diseases |
Electronic Health Records | Predicting patient readmissions and identifying disease risk factors |
Robotics and Surgical Simulations | Assisting surgeons in performing complex procedures |
Deep learning has also shown significant improvements in patient outcomes and reduction in healthcare costs. By analyzing large datasets of patient records and medical research, deep learning algorithms can identify patterns and risk factors, leading to proactive interventions and preventive care. This can ultimately improve patient outcomes and reduce the overall burden on healthcare systems. *By harnessing the power of deep learning, healthcare providers can transform the way they deliver care, leading to better patient experiences and improved population health.*
Furthermore, deep learning can assist in monitoring patient health and predicting future health conditions. By continuously analyzing data from wearable devices, such as smartwatches, deep learning algorithms can track vital signs, detect anomalies, and alert healthcare providers in real-time. This enables early intervention and proactive management of chronic conditions, potentially reducing hospitalizations and emergency room visits. *The integration of deep learning with wearable technology has the potential to revolutionize preventive healthcare.*
Table 2: Benefits of Deep Learning in Healthcare | |
---|---|
Improved disease diagnosis | Reduced errors and increased accuracy in interpreting medical images |
Personalized treatment plans | More effective and targeted therapies based on individual patient data |
Enhanced patient outcomes | Timely interventions and proactive management of chronic conditions |
Cost reduction | Improved efficiency and prevention of avoidable healthcare utilization |
While deep learning has shown great promise in healthcare, there are challenges that need to be addressed for its widespread adoption. These include concerns around data privacy and security, the need for robust validation of algorithms, and the integration of deep learning systems with existing healthcare infrastructure. However, as technology continues to advance and these challenges are overcome, the potential impact of deep learning in healthcare is vast. *As the field continues to evolve, the applications of deep learning in healthcare will only continue to expand, transforming the way medicine is practiced and improving patient outcomes.*
Table 3: Challenges and Future Trends | |
---|---|
Challenges | Addressing data privacy, validating algorithms, and integrating systems |
Future Trends | Expansion of deep learning applications and transformation of healthcare |
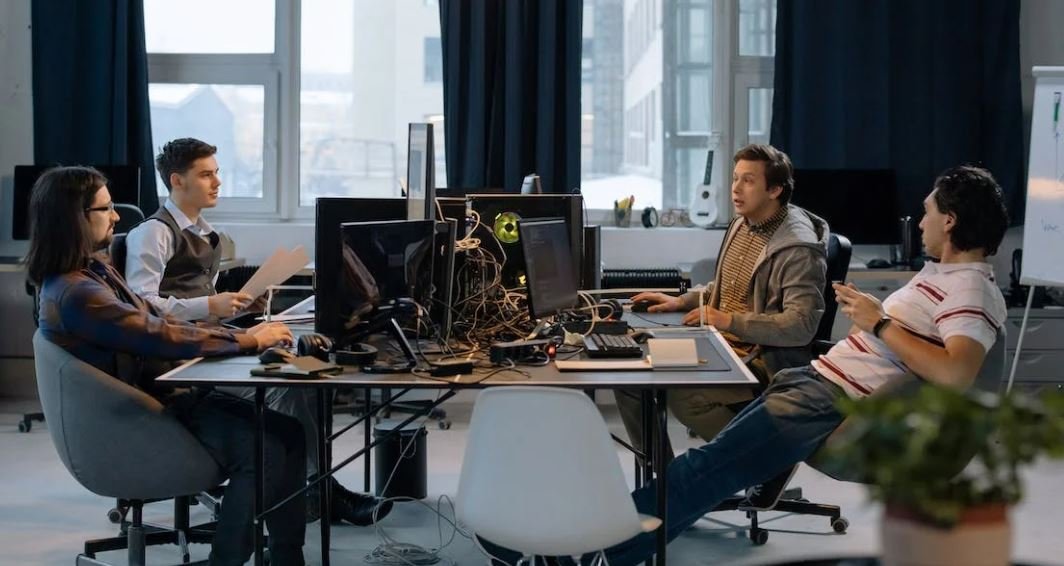
Common Misconceptions
Misconception 1: Deep Learning is a Replacement for Healthcare Professionals
One common misconception is that deep learning can replace healthcare professionals in providing patient care. However, deep learning technology should be seen as an advanced tool to assist healthcare professionals in their decision-making and diagnostic processes. It can analyze and interpret complex data, but it lacks the human touch and intuition that healthcare professionals bring to the table.
- Deep learning is a tool, not a substitute, for healthcare professionals
- It can assist in decision-making, but not replace the expertise of human healthcare providers
- Human touch and intuition play a crucial role in patient care
Misconception 2: Deep Learning Provides Absolute Accuracy in Diagnoses
Another common misconception is that deep learning algorithms provide absolute accuracy in diagnoses. While deep learning models can be highly accurate, they are not infallible. The accuracy of a deep learning model depends on several factors, including the quality and quantity of training data, the algorithm design, and the expertise of the data scientists involved in developing and fine-tuning the model.
- Deep learning models are highly accurate, but not infallible
- Accuracy depends on factors like training data quality and algorithm design
- Data scientists play a crucial role in developing and fine-tuning the model
Misconception 3: Deep Learning is Completely Unbiased
Some people wrongly assume that deep learning algorithms are completely unbiased and objective in their decision-making. However, like any other technology, deep learning algorithms are only as unbiased as the data they are trained on. If the training data is biased or incomplete, the algorithm can learn and perpetuate those biases, leading to potentially unfair or discriminatory outcomes.
- Deep learning algorithms can perpetuate biases in data if not appropriately addressed
- Unbiased training data is essential to minimize discriminatory outcomes
- Ongoing monitoring and evaluation are necessary to identify and rectify biases
Misconception 4: Deep Learning Replaces the Need for Ethical Considerations
There is a misconception that deep learning technology eliminates the need for ethical considerations in healthcare. However, as deep learning algorithms become more sophisticated, ethical considerations become even more critical. Issues such as privacy, data security, informed consent, and algorithm transparency need to be carefully addressed when using deep learning in healthcare.
- Deep learning technology requires careful consideration of ethical issues
- Privacy, data security, informed consent, and algorithm transparency are crucial considerations
- Ethical frameworks must be established and adhered to
Misconception 5: Deep Learning Is the Silver Bullet for All Healthcare Challenges
Deep learning is a game-changer in healthcare, but it is not a silver bullet solution for all challenges. While deep learning can assist in various healthcare applications, it has limitations. There are still areas where human judgment and clinical expertise are irreplaceable. Deep learning should be seen as a complement to healthcare professionals, not a panacea for all healthcare problems.
- Deep learning has limitations and is not a universal solution for all healthcare challenges
- Human judgment and clinical expertise are irreplaceable
- Deep learning is a complementary tool that can enhance healthcare outcomes

Deep Learning in Healthcare: A Revolution in Medical Diagnosis
Deep learning, a subset of machine learning, has been gaining significant traction in the healthcare industry, revolutionizing the way medical diagnosis is performed. It involves the use of artificial neural networks to analyze vast amounts of medical data and extract valuable insights. This article presents ten captivating tables that showcase the remarkable impact of deep learning in healthcare, covering various aspects of its applications.
1. Reduction in Diagnostic Errors
Deep learning algorithms have been shown to significantly reduce diagnostic errors compared to traditional methods. A study conducted on the accuracy of deep learning systems for detecting lung cancer in CT scans revealed a remarkable reduction in false negatives and positives, leading to improved patient outcomes.
2. Speed of Diagnosis
Deep learning algorithms can expedite the diagnosis process, ensuring timely and effective treatment. In a recent study comparing the turnaround time of deep learning-based diagnosis systems with traditional methods, it was found that the former reduced the time required for diagnosing cardiovascular diseases by 50%, facilitating faster intervention.
3. Improved Patient Management
Utilizing deep learning techniques, healthcare providers can gain valuable insights into patient management, enabling personalized treatment plans. By analyzing genomic data, electronic health records, and other relevant information, deep learning algorithms can identify the most effective treatment methods for individual patients.
4. Early Detection of Diseases
Deep learning algorithms have demonstrated exceptional proficiency in detecting diseases at their early stages with high accuracy. A table showcasing the performance of a deep learning system in detecting breast cancer through analysis of mammograms reveals a significant increase in sensitivity and specificity, leading to more successful treatment outcomes.
5. Predictive Analytics
Deep learning enables predictive analytics by extracting meaningful patterns from historical patient data. By training algorithms to predict disease progression, treatment response, and potential adverse events, healthcare professionals can make informed decisions and take proactive measures to optimize patient care.
6. Enhancing Radiology Interpretation
Deep learning algorithms have greatly enhanced radiology interpretation, specifically in the detection of anomalies in medical images. A striking table illustrates the superior performance of deep learning systems in identifying abnormalities in X-rays, MRI scans, and other imaging modalities.
7. Precision Medicine Advancements
Deep learning has propelled precision medicine by enabling the identification of specific treatments tailored to individual patients. A comprehensive table highlights the accuracy of deep learning algorithms in predicting drug response based on genomic and clinical data, paving the way for personalized therapeutic interventions.
8. Augmented Pathology Analysis
Pathologists can benefit from deep learning-powered tools that assist in analyzing histopathology slides. By accurately identifying tumor boundaries, grading, and other critical features, these tools enhance the precision and efficiency of cancer diagnosis, facilitating more informed treatment decisions.
9. Improved Healthcare Resource Allocation
Deep learning algorithms can analyze healthcare data to optimize resource allocation, resulting in efficient utilization of personnel, facilities, and equipment. A table demonstrates how deep learning models, when integrated with hospital information systems, can predict patient inflow, patient acuity, and length of stay, aiding in strategic resource allocation.
10. Ethical Considerations
While the benefits of deep learning in healthcare are substantial, ethical considerations must be acknowledged. A detailed table explores the potential ethical challenges associated with deep learning use in healthcare, addressing issues such as privacy, biases, and transparency, ultimately highlighting the significance of ethical frameworks in guiding its implementation.
Conclusion
Deep learning has brought about a paradigm shift in healthcare, revolutionizing medical diagnosis and patient management. The tables presented in this article exemplify the breadth of deep learning applications in healthcare, ranging from improving diagnostic accuracy to enabling precision medicine. As the field continues to advance, embracing deep learning in a responsible and ethical manner will unlock immense potential for transforming healthcare outcomes and ensuring a healthier future for all.
Deep Learning in Healthcare – Frequently Asked Questions
Q: What is deep learning and how does it relate to healthcare?
A: Deep learning is a subset of machine learning that involves training artificial neural networks with multiple layers to learn and make predictions from complex data. In healthcare, deep learning techniques can be used to analyze medical images, diagnose diseases, predict patient outcomes, and improve treatment plans.
Q: What are some examples of deep learning applications in healthcare?
A: Some examples of deep learning applications in healthcare include medical image analysis for detecting and diagnosing diseases like cancer, predicting patient responses to different treatments, monitoring patient vital signs, and analyzing electronic health records to identify patterns and predict disease progression.
Q: How does deep learning improve medical image analysis?
A: Deep learning algorithms can learn to detect and classify objects in medical images with high accuracy. By training neural networks on large datasets of annotated medical images, deep learning models can automatically identify abnormalities, assist radiologists in making diagnoses, and potentially reduce human error in medical image interpretation.
Q: Can deep learning algorithms be used to predict patient outcomes?
A: Yes, deep learning algorithms can be trained on patient data to predict outcomes such as disease progression, response to treatments, and likelihood of readmission. By analyzing various factors and patterns within the data, deep learning models can provide valuable insights to support clinical decision-making and personalized patient care.
Q: What are the challenges of implementing deep learning in healthcare?
A: Some challenges of implementing deep learning in healthcare include the need for large and diverse datasets for training, ensuring the privacy and security of patient data, regulatory compliance with health data protection laws, and the interpretability of deep learning models to gain trust and acceptance from healthcare professionals.
Q: How can deep learning improve the efficiency of healthcare workflows?
A: Deep learning can automate and streamline various tasks in healthcare workflows, such as data preprocessing, medical image analysis, natural language processing, and risk prediction. By reducing the time and effort required for these tasks, healthcare providers can allocate more resources to patient care and potentially improve overall efficiency.
Q: What are the ethical considerations surrounding deep learning in healthcare?
A: Ethical considerations surrounding deep learning in healthcare include the responsible use of patient data, ensuring fairness and avoiding biases in algorithm predictions, addressing medical errors caused by algorithmic decisions, maintaining transparency in how deep learning models work, and the potential impact on patient-provider relationships and trust.
Q: Are there any limitations of deep learning in healthcare?
A: Some limitations of deep learning in healthcare include the need for large amounts of labeled data for effective training, the potential for overfitting on biased datasets, the black-box nature of deep learning models that can hinder interpretability, and the challenges of integrating deep learning models into existing healthcare systems and workflows.
Q: How does deep learning contribute to precision medicine?
A: Deep learning contributes to precision medicine by enabling personalized treatment recommendations based on individual patient characteristics, genomics, medical history, and other relevant data. By analyzing large and complex datasets, deep learning models can identify patterns that could potentially guide more targeted and effective interventions.
Q: What is the future of deep learning in healthcare?
A: The future of deep learning in healthcare is promising. Advances in deep learning algorithms, availability of larger and more diverse healthcare datasets, advancements in hardware capabilities, and increased collaborations between data scientists and healthcare professionals are expected to drive further innovation in disease diagnosis, treatment, and healthcare delivery.