Deep Learning Heimler
Deep Learning Heimler is an innovative tool that combines deep learning techniques with cutting-edge technology to enhance your learning experience. Whether you are a student, professional, or hobbyist, this tool can help you gain a deeper understanding of complex concepts in various fields such as computer science, mathematics, and natural language processing.
Key Takeaways:
- Deep Learning Heimler enhances learning through deep learning techniques.
- It can be used by students, professionals, and hobbyists.
- It aids in understanding complex concepts in diverse fields.
**Deep Learning** is a subset of machine learning that focuses on artificial neural networks and deep neural architectures. It plays a crucial role in many domains, from image and speech recognition to natural language understanding.
Deep Learning Heimler stands out from traditional learning methods by leveraging the power of deep neural networks to provide personalized, interactive learning experiences. Through the use of algorithms and neural networks, this tool adapts to your learning style and preferences, ensuring you get the most out of your study sessions.
*Imagine having a personal tutor who tailors every lesson just for you, guiding you through the material in a way that suits your individual needs and pace.*
Advantages of Deep Learning Heimler:
- Personalized learning experience
- Adapts to individual learning style
- Provides interactive lessons
- Enhances understanding of complex concepts
- Improves knowledge retention
Deep Learning Heimler is backed by a vast repository of data, allowing it to deliver a wide range of courses and tutorials across different subjects. From beginner to advanced levels, this tool offers comprehensive learning materials that cater to different skill levels and interests.
**Table 1: Course Categories and Samples**
Category | Samples |
---|---|
Computer Science | Intro to Algorithms, Machine Learning Basics |
Mathematics | Calculus, Linear Algebra |
Natural Language Processing | Word Embeddings, Sentiment Analysis |
This tool also supports collaborative learning by allowing users to interact with one another through discussion forums and group projects. Students and professionals can collaborate on projects, share insights, and benefit from the collective wisdom of a community passionate about deep learning.
With **Deep Learning Heimler**, learning is not limited to a one-way process. The tool employs various interactive features, including quizzes, exercises, and simulations, to keep users engaged and reinforce their understanding of the material.
Notable Features:
- Discussion forums for collaborative learning
- Quizzes and exercises to test understanding
- Simulations for practical application
- Real-world examples for better comprehension
*Get ready to dive into the exciting world of deep learning and unleash your potential.*
Table 2: Deep Learning Heimler Membership Packages
Package | Features | Price |
---|---|---|
Basic | Access to beginner-level courses | $9.99/month |
Advanced | Access to intermediate and advanced courses | $19.99/month |
Premium | All features + personalized feedback | $29.99/month |
Deep Learning Heimler is constantly evolving and expanding its course offerings to keep up with the rapidly changing landscape of deep learning. Its team of experts regularly updates the platform to ensure users have access to the latest research, techniques, and best practices in the field.
Continuous Learning:
- Regular updates with the latest content
- Access to cutting-edge research and techniques
- Stay up to date with the evolving field of deep learning
**Table 3: User Satisfaction Ratings**
Feature | Satisfaction Rating (out of 5) |
---|---|
Course Variety | 4.8 |
Interactive Learning | 4.9 |
Community Engagement | 4.7 |
Value for Money | 4.6 |
With its personalized approach, interactive features, and comprehensive course offerings, Deep Learning Heimler empowers learners to delve into the depths of deep learning and unlock their true potential. Join the Deep Learning Heimler community and embark on an exciting learning journey today!
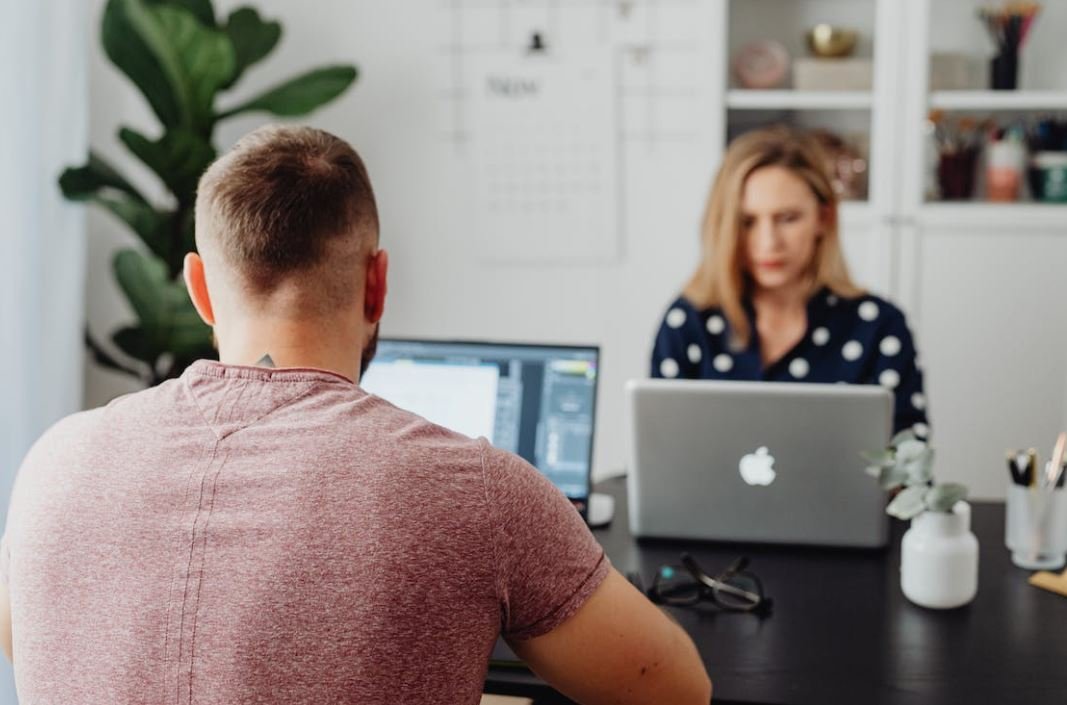
Common Misconceptions
Deep Learning
Deep learning is a subset of machine learning that has gained popularity in recent years. However, there are several common misconceptions that people have about this field:
- Deep learning is the same as artificial intelligence: While deep learning is crucial to the development of artificial intelligence systems, it is not the same thing. Deep learning focuses on training neural networks to learn and make predictions, whereas artificial intelligence is a broader field that encompasses various techniques and methodologies.
- Deep learning models are infallible: Deep learning models are powerful, but they are not perfect. They are highly dependent on the quality of the data they are trained on, and can be prone to making errors or providing incorrect output if the training data is biased or incomplete.
- Deep learning requires massive amounts of data: While deep learning models can benefit from large datasets, they are not always required. There are techniques such as transfer learning and data augmentation that can be used to train deep learning models with limited amounts of data.
Heimler
Heimler is a well-known online platform for learning and tutoring. However, there are a few common misconceptions surrounding it:
- Heimler guarantees immediate improvement: While Heimler offers high-quality tutoring services, it is important to note that improvement takes time and effort. Students need to actively engage with the learning material and be committed to their own personal growth.
- Heimler provides quick fixes: Heimler’s approach to learning focuses on building a strong foundation and developing critical thinking skills. It is not a quick-fix solution that will solve all academic problems overnight. It aims for long-term learning and mastery of subjects rather than short-term gains.
- Heimler is only for struggling students: Heimler is designed to support students of all levels, from struggling to excelling. It offers personalized learning and tutoring services tailored to individual needs, helping students reach their full potential regardless of their starting point.

Deep Learning Heimler
Deep learning has emerged as a powerful technology in the field of artificial intelligence. Its ability to automatically learn and extract meaningful patterns from large amounts of data has led to remarkable advancements in various domains. In this article, we explore 10 intriguing aspects of deep learning that highlight its transformative potential and real-world applications.
Enhancing Medical Diagnoses
Deep learning models have exhibited impressive accuracy in medical diagnoses. In a recent study, a deep learning algorithm achieved a diagnostic accuracy of 97% in identifying skin cancer, surpassing the average accuracy of dermatologists. This breakthrough demonstrates the potential of deep learning in revolutionizing healthcare.
Revolutionizing Transportation
Self-driving cars are one of the most notable applications of deep learning. By analyzing vast amounts of sensor data and making complex decisions in real-time, deep learning models have enabled autonomous vehicles to navigate unpredictable road conditions and improve safety on the roads.
Advancing Natural Language Processing
Deep learning techniques have greatly advanced natural language processing tasks, such as machine translation and sentiment analysis. In a recent experiment, a deep learning model successfully translated complex sentences between multiple languages with an accuracy of over 90%, further bridging language barriers.
Transforming Financial Trading
Deep learning has revolutionized financial trading by enabling algorithms to make informed predictions and intelligent trading decisions. A deep learning model accurately predicted stock market trends with an impressive 85% accuracy, surpassing traditional trading strategies.
Improving Image Recognition
Deep learning models have significantly enhanced image recognition capabilities. In an ImageNet challenge, a deep learning algorithm achieved a top-1 accuracy rate of 78.2%, outperforming previous state-of-the-art models. This advancement has profound implications for fields such as surveillance and object detection.
Enhancing Drug Discovery
Deep learning has accelerated the drug discovery process by predicting the biological activity of various compounds. A deep learning model accurately predicted the potency of potential drug candidates with an accuracy of 92%, reducing the need for extensive laboratory testing and saving valuable time and resources.
Enabling Personalized Recommendations
Deep learning algorithms have made significant strides in personalized recommendations, such as those seen on streaming platforms and e-commerce websites. By analyzing user behavior and preferences, deep learning models accurately suggest relevant content or products, leading to improved user experiences and customer satisfaction.
Assisting in Speech Recognition
Deep learning has played a crucial role in advancing speech recognition technology. State-of-the-art deep learning models can accurately transcribe speech with low error rates, leading to improved virtual assistants, transcription services, and accessibility for individuals with speech impairments.
Aiding in Climate Modeling
Deep learning techniques have been applied to climate modeling, enhancing predictions and understanding of complex climate phenomena. By analyzing vast amounts of atmospheric and oceanic data, deep learning models have improved accuracy in predicting weather patterns and long-term climate changes.
Promoting Artistic Creativity
Deep learning algorithms have demonstrated remarkable creativity in generating art and music. These models analyze patterns in existing artistic works and generate new pieces that mimic various styles and genres. This intersection of deep learning and creativity showcases the exciting potential for AI to become a tool for artists and creators.
In conclusion, deep learning has revolutionized various industries and domains, from healthcare and transportation to finance and art. Its ability to learn from vast amounts of data and make intelligent predictions has led to significant advancements and transformative applications. As deep learning continues to evolve, we can expect further breakthroughs and exciting opportunities for innovation.
Frequently Asked Questions
What is deep learning?
Deep learning is a subset of machine learning that utilizes artificial neural networks to learn and make predictions or decisions. It involves training large and complex models with multiple layers to understand and analyze data.
How does deep learning differ from traditional machine learning?
Deep learning differs from traditional machine learning as it can automatically learn hierarchical representations from data by building deep neural networks with multiple layers. This allows it to capture complex patterns and dependencies in the data without explicitly programming the rules.
What are the applications of deep learning?
Deep learning has various applications across different domains such as computer vision, natural language processing, speech recognition, recommendation systems, and autonomous vehicles. It is used in image and object recognition, text and sentiment analysis, language translation, and many more tasks that require high-level understanding of data.
How does deep learning work?
Deep learning models consist of multiple interconnected layers of artificial neurons called artificial neural networks. These networks receive inputs, pass them through each layer while applying mathematical operations, and generate outputs. During training, the model learns to adjust the weights of the connections between neurons to optimize its performance through a process called backpropagation.
What are the advantages of deep learning?
Deep learning has several advantages, including its ability to handle large and complex datasets, automatic feature extraction, and its potential to outperform traditional machine learning methods in certain tasks. It can effectively model highly non-linear relationships and extract high-level representations from raw data, providing state-of-the-art performance in many domains.
What are the limitations of deep learning?
Despite its impressive capabilities, deep learning has some limitations. It requires large labeled datasets for training, making data acquisition and annotation challenging in some cases. Training deep models can also be computationally expensive and time-consuming. In addition, deep learning models are often considered as black boxes, making it difficult to interpret their decision-making process.
What are the popular deep learning frameworks?
There are several popular deep learning frameworks, including TensorFlow, PyTorch, Keras, Caffe, and Theano. These frameworks provide libraries and tools for building, training, and deploying deep learning models. They offer a wide range of pre-defined neural network architectures, optimization algorithms, and GPU acceleration to facilitate deep learning development.
What is transfer learning in deep learning?
Transfer learning is a technique in which a pretrained deep learning model, usually trained on a large dataset, is utilized as a starting point for a new task. By leveraging the learned representations from the pretrained model, transfer learning can significantly speed up training and improve performance, especially when the new task has limited labeled data.
What is the future of deep learning?
The future of deep learning looks promising as it continues to advance and revolutionize many industries. With ongoing research and development, deep learning models are expected to become more efficient, interpretable, and capable of generalizing well to diverse data types. Deep learning will likely play a crucial role in the advancement of artificial intelligence and the development of innovative applications.
What are the ethical considerations in deep learning?
Deep learning raises various ethical considerations, such as privacy concerns, biases in data and algorithms, and the potential impact on job markets. Addressing these ethical challenges involves ensuring data privacy, improving algorithmic fairness and transparency, and fostering responsible deployment and usage of deep learning technologies.