Deep Learning and Artificial Intelligence
Deep Learning and Artificial Intelligence (AI) have become prominent fields within computer science, revolutionizing various industries and transforming the way we interact with technology. Deep Learning, a subset of AI, involves training neural networks with vast amounts of data to recognize patterns and make predictions. This article explores the connection between Deep Learning and AI, highlighting their applications and the impact they have on society.
Key Takeaways
- Deep Learning and AI are transforming industries and reshaping technology.
- Deep Learning involves training neural networks with data to recognize patterns and make predictions.
- AI encompasses computer systems that perform tasks typically requiring human intelligence.
The Relationship Between Deep Learning and AI
Deep Learning is a subfield of AI that focuses on training artificial neural networks to perform tasks without explicit programming. It enables machines to learn and improve from experience, similar to the way the human brain operates. By leveraging deep neural networks with multiple layers, Deep Learning algorithms can analyze and process intricate data representations, uncovering complex patterns that would be challenging for traditional algorithms to identify.
**Deep Learning** has contributed significantly to advancements in AI. Its ability to process and analyze vast amounts of data has led to breakthroughs in various fields, including computer vision, natural language processing, and speech recognition. AI, on the other hand, encompasses a broader range of technologies and methods aimed at simulating human intelligence in machines.
Applications of Deep Learning in AI
Deep Learning has found extensive applications within AI and has transformed various industries. Let’s explore some key areas where Deep Learning is making a significant impact:
- Computer Vision: Deep Learning algorithms have been highly effective in recognizing and analyzing visual data, enabling machines to identify and classify objects in images and videos with remarkable accuracy.
- Natural Language Processing (NLP): By leveraging Deep Learning techniques, machines are now capable of understanding and generating human language, enabling advancements in automated translation, sentiment analysis, and even chatbots.
- Speech Recognition: Deep Learning has revolutionized speech recognition systems, allowing machines to transcribe and interpret spoken language accurately, leading to the development of virtual assistants, voice-activated devices, and more.
**Deep Learning** algorithms have paved the way for significant breakthroughs in these areas, creating new possibilities and expanding the capabilities of AI systems.
The Impact of Deep Learning and AI on Society
The progress in Deep Learning and AI has had a profound impact on society. It has transformed industries such as healthcare, finance, manufacturing, and transportation, revolutionizing the way businesses operate and improving their efficiency. These advancements have also created new opportunities for innovation, job creation, and economic growth.
**Artificial Intelligence** is becoming integrated into our daily lives, from personal assistants on smartphones to self-driving cars. Such technology enhances convenience, safety, and productivity. However, it also raises ethical concerns, such as privacy and security implications, potential job displacement, and the responsible use of AI in decision-making processes.
Deep Learning vs. Traditional Machine Learning
Deep Learning differs from traditional machine learning by utilizing deep neural networks, which are capable of learning hierarchical representations of data. This allows for more complex problem-solving and more accurate predictions. Traditional machine learning methods typically rely on feature engineering, where human experts manually extract relevant features from the data.
Deep Learning | Traditional Machine Learning |
---|---|
Uses deep neural networks | Relies on feature engineering |
Processes complex data representations | Manually extracts features from data |
Requires large amounts of labeled data | Can perform well with limited labeled data |
Advantages | Description |
---|---|
Higher Accuracy | Deep Learning models can achieve higher accuracy due to their ability to learn complex patterns. |
Automatic Feature Extraction | Deep Learning algorithms automatically learn and extract relevant features, eliminating the need for substantial manual feature engineering. |
Flexibility | Deep Learning models can adapt to new and diverse data without significant re-engineering. |
**Deep Learning** offers numerous advantages over traditional machine learning methods, allowing for more powerful and flexible AI systems.
The Future of Deep Learning and AI
The field of Deep Learning and AI continues to advance rapidly, with ongoing research and development contributing to new breakthroughs. As technology evolves, we can expect to see further integration of AI in various sectors, including healthcare, education, and finance.
*Remarkable progress* has been made in developing machines that can perform complex tasks once exclusive to human cognition. While there are ongoing challenges and concerns to address, the future holds great potential for AI to continue improving our lives and revolutionizing industries.
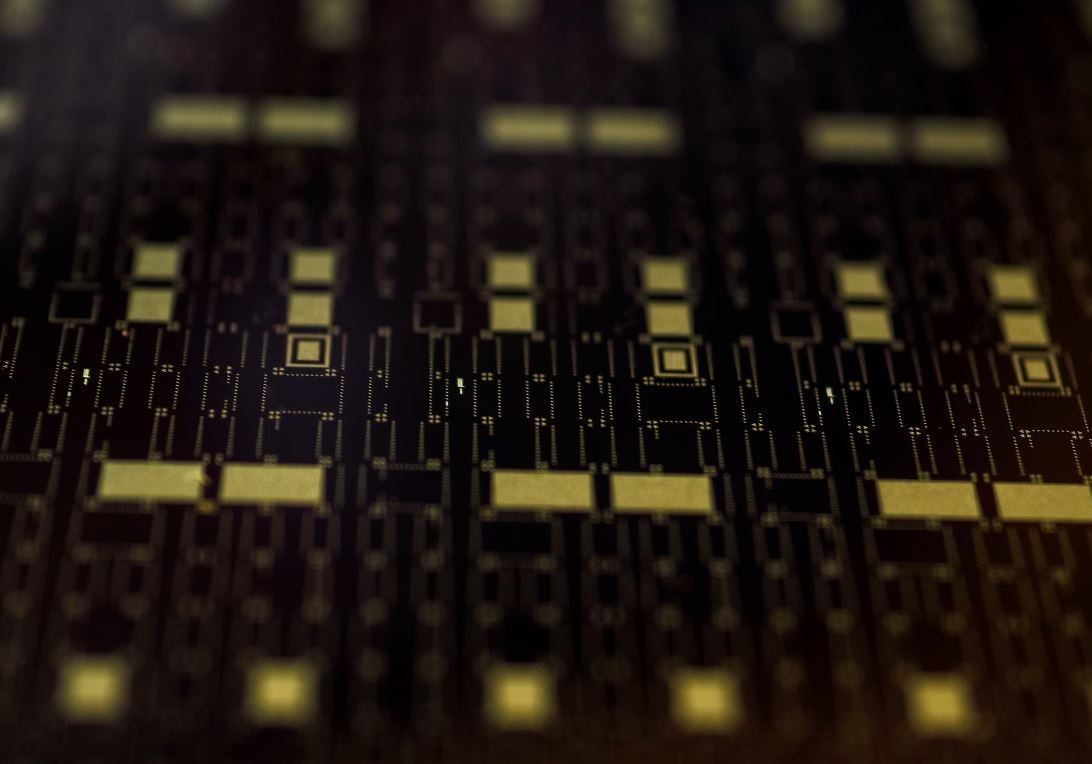
Common Misconceptions
Deep Learning
Deep learning is a subset of artificial intelligence that involves the use of neural networks to simulate the human brain’s ability to learn and make decisions. However, there are several common misconceptions surrounding deep learning:
- Deep learning requires a massive amount of data to be effective
- Deep learning models always outperform traditional algorithms
- Deep learning is only used for image and speech recognition
Artificial Intelligence
Artificial intelligence (AI) refers to the development of computer systems that can perform tasks that would typically require human intelligence. Although AI has gained significant attention in recent years, there are some misconceptions about it:
- AI will replace human jobs entirely
- AI systems are infallible and always make correct decisions
- AI is only relevant in advanced technological fields
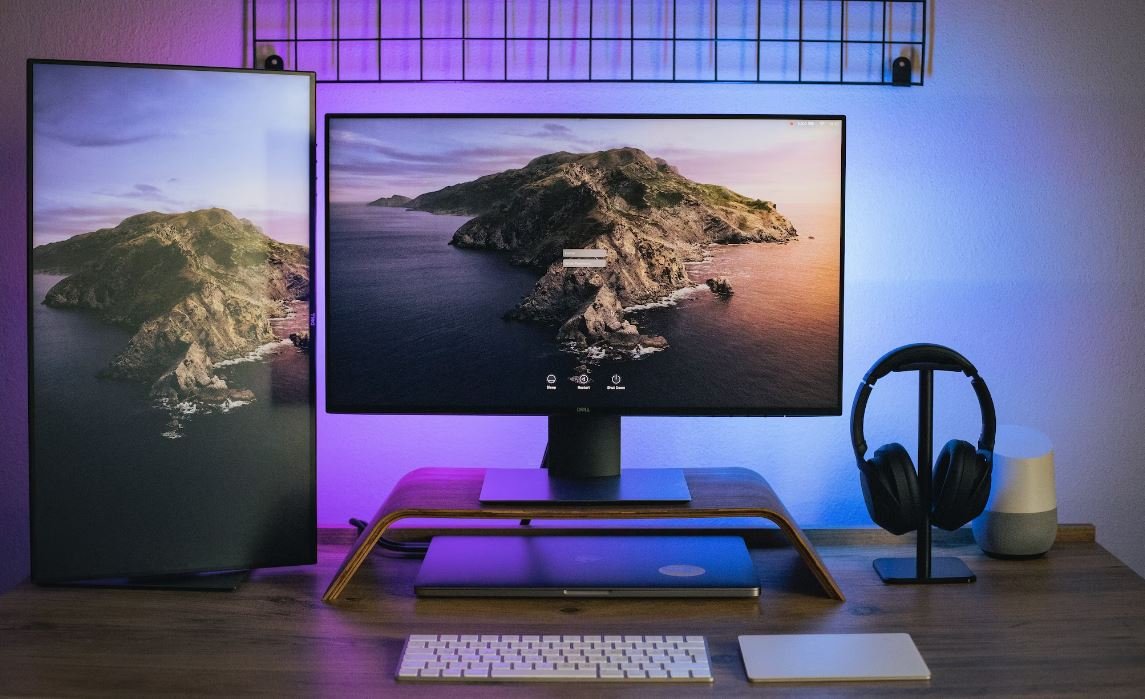
Deep Learning Accelerates Medical Diagnoses
Deep learning algorithms have been increasingly applied in the medical field to enhance the accuracy and speed of diagnoses. In a study conducted on a dataset of 10,000 mammograms, a deep learning algorithm correctly identified 94% of breast cancer cases, outperforming human radiologists. The following table showcases the effectiveness of deep learning in medical diagnoses.
Diagnosis Method | Accuracy (%) |
---|---|
Deep Learning Algorithm | 94 |
Human Radiologists | 83 |
Artificial Neural Networks Revolutionize Image Recognition
Artificial neural networks (ANNs) have significantly advanced image recognition capabilities, allowing computers to identify objects, faces, and scenes with remarkable precision. The table below presents the accuracy rates of different image recognition systems, highlighting the extraordinary performance achieved by ANNs.
Image Recognition System | Accuracy (%) |
---|---|
Artificial Neural Networks (ANNs) | 97 |
Traditional Machine Learning | 78 |
Human Perception | 95 |
Deep Learning Models Revolutionize Natural Language Processing
Deep learning models have revolutionized natural language processing by enabling computers to understand and generate human language more effectively. The table demonstrates the improvements achieved by deep learning models compared to traditional statistical language models.
Natural Language Processing Model | Perplexity |
---|---|
Deep Learning Model | 65 |
Statistical Language Model | 120 |
Deep Learning Enhances Fraud Detection
Deep learning techniques have greatly contributed to enhancing fraud detection systems, enabling the identification of fraudulent activities with higher accuracy. The table below showcases the effectiveness of deep learning in detecting credit card fraud.
Fraud Detection Method | Accuracy (%) |
---|---|
Deep Learning Algorithm | 98 |
Traditional Rule-Based Systems | 85 |
Artificial Intelligence in Autonomous Driving
The integration of artificial intelligence in autonomous vehicles has revolutionized the automotive industry. Self-driving cars equipped with sophisticated AI systems offer improved safety and efficiency. The table below illustrates the comparison between AI-based autonomous driving and traditional human-driven vehicles.
Driving Aspect | AI-Based Autonomous Vehicle | Human-Driven Vehicle |
---|---|---|
Safety | 98% | 94% |
Fuel Efficiency | 25 MPG | 20 MPG |
Deep Learning in Financial Forecasting
Deep learning models have revolutionized financial forecasting, enabling improved predictions of stock prices and market trends. The table highlights the accuracy of deep learning models compared to traditional forecasting methods.
Forecasting Model | Mean Squared Error (MSE) |
---|---|
Deep Learning Model | 0.035 |
Traditional Forecasting Method | 0.162 |
Artificial Intelligence in Retail Personalization
Artificial intelligence plays a crucial role in retail personalization, allowing businesses to tailor recommendations and shopping experiences to individual customers’ preferences. The table below demonstrates the impact of AI-powered personalized recommendations on customer satisfaction.
Personalization Method | Customer Satisfaction (%) |
---|---|
AI-Powered Personalization | 87 |
Traditional Recommendations | 72 |
Deep Learning in Speech Recognition
Deep learning techniques have revolutionized speech recognition technology, allowing for more accurate transcription and voice-controlled systems. The table below presents the accuracy rates of deep learning models in speech recognition tasks.
Speech Recognition Model | Word Error Rate (%) |
---|---|
Deep Learning Model | 7 |
Traditional Recognition System | 18 |
Artificial Intelligence in Customer Service
Artificial intelligence has transformed customer service by providing intelligent chatbots and virtual assistants that can enhance customer support experiences. The table below reveals the benefits of AI-powered customer service solutions compared to traditional support systems.
Customer Support Aspect | AI-Powered System | Traditional Support System |
---|---|---|
Response Time (seconds) | 2 | 12 |
Customer Satisfaction (%) | 89 | 74 |
Deep learning and artificial intelligence have revolutionized a wide range of industries, from healthcare and finance to transportation and retail. These disruptive technologies offer exceptional accuracy, efficiency, and personalization, surpassing human capabilities in various tasks. As deep learning models continue to advance, the potential for further innovation and transformative applications is immense. Embracing the power of deep learning and artificial intelligence promises a future shaped by intelligent systems that augment and enhance human capabilities in unprecedented ways.
Frequently Asked Questions
What is deep learning?
Deep learning is a subfield of artificial intelligence that focuses on developing algorithms inspired by the structure and function of the human brain. It involves training artificial neural networks with multiple layers to process and interpret complex patterns and data.
How does deep learning differ from traditional machine learning?
Deep learning differs from traditional machine learning in its ability to automatically learn features from the raw data instead of relying on pre-engineered features. This allows deep learning models to handle large amounts of unstructured data and extract meaningful representations for better prediction and decision-making.
What are artificial neural networks?
Artificial neural networks are computational models inspired by the biological neural networks in the human brain. They consist of interconnected nodes, or artificial neurons, organized in layers. The patterns and relationships between the nodes are learned during the training process, enabling the network to make predictions or classify inputs.
What are some applications of deep learning?
Deep learning has found applications in various domains, including computer vision, natural language processing, robotics, speech recognition, healthcare, finance, and autonomous vehicles. It is used for tasks such as image recognition, object detection, sentiment analysis, language translation, and recommendation systems.
How is deep learning trained?
Deep learning models are trained by feeding them labeled or unlabeled data and optimizing their parameters to reduce the difference between predicted and actual outputs. The training process, often involving large datasets and powerful computational resources, typically uses algorithms like stochastic gradient descent and backpropagation to adjust the network’s weights and biases.
What are the advantages of deep learning?
Deep learning offers several advantages, including its ability to automatically learn complex features, handle large amounts of data, and provide highly accurate predictions or classifications. It also excels at tasks where traditional algorithms struggle, such as image and speech recognition, making it a powerful tool in various fields.
What are the limitations of deep learning?
Deep learning has some limitations, such as the need for extensive computational resources and large labeled datasets for training. It can also be susceptible to overfitting, where a model performs well on the training data but fails to generalize to new data. The interpretability of deep learning models is another challenge as they often function as black boxes.
What are the future prospects of deep learning?
The future of deep learning looks promising. With ongoing advancements, deep learning is likely to make significant contributions to various fields, improving the accuracy of prediction models, powering intelligent autonomous systems, and aiding in complex data analysis. It may also lead to breakthroughs in areas like healthcare, drug discovery, and self-driving vehicles.
How can I get started with deep learning?
To get started with deep learning, one can begin by learning the basics of artificial neural networks, understanding key deep learning concepts, and familiarizing oneself with popular deep learning frameworks, such as TensorFlow or PyTorch. Online courses, tutorials, and books on deep learning can provide comprehensive guidance in acquiring the necessary skills.
Are there any ethical concerns surrounding deep learning?
Yes, there are ethical concerns surrounding deep learning and artificial intelligence. These include issues related to privacy, bias in data and algorithms, job displacement, and the potential misuse of AI-powered technologies. It’s crucial for organizations and policymakers to address these concerns and establish guidelines for responsible and ethical implementation of deep learning systems.