Deep Learning AI
Deep learning AI, also known as deep neural networks or deep learning models, is a subset of artificial intelligence (AI) that focuses on the development of algorithms and models that can learn and make decisions on their own, without explicit programming. This advanced form of AI is revolutionizing various industries, from healthcare to finance, by enabling machines to comprehend, analyze, and learn from complex data patterns.
Key Takeaways
- Deep learning AI utilizes deep neural networks to make autonomous decisions.
- It is transforming industries by enabling data-driven decision-making.
- Deep learning AI enables machines to understand and analyze complex data patterns.
- It has significant applications in healthcare, finance, and other industries.
Deep learning AI models consist of multiple layers of interconnected artificial neurons that mimic the structure and functioning of the human brain. Each layer processes specific features of input data, and these features are progressively combined and analyzed in subsequent layers, leading to higher-level representations and ultimately making accurate predictions or decisions. This intricate architecture allows deep learning models to tackle complex problems and excel in areas such as image recognition, natural language processing, and speech recognition.
*Deep learning AI models are designed with multiple layers of interconnected artificial neurons.*
One of the key advantages of deep learning AI is its ability to automatically learn and extract relevant features from raw data, eliminating the need for manual feature engineering. Traditional machine learning algorithms heavily rely on curated feature selection, which can be time-consuming and subjective. Deep learning models, on the other hand, can autonomously learn the most relevant features from the data, enabling them to achieve higher accuracy and performance.
*Deep learning AI eliminates the need for manual feature engineering.*
Deep learning AI has paved the way for significant advancements in various industries. In healthcare, deep learning models have been successfully applied to medical imaging analysis, disease diagnosis, and drug discovery. These models can effectively detect abnormalities in medical images, assess tumor growth, and predict patient outcomes. In finance, deep learning algorithms have been utilized for fraud detection, stock market prediction, and algorithmic trading. These models can analyze large amounts of financial data, detect unusual patterns, and make intelligent investment decisions.
*Deep learning AI has revolutionized healthcare and finance industries with its applications in medical imaging analysis and fraud detection.*
Advancements in Deep Learning AI
Over the years, deep learning AI has witnessed significant advancements, thanks to ongoing research and technological breakthroughs. Notable advancements include:
- Efficient training algorithms, such as stochastic gradient descent, that accelerate the learning process.
- The emergence of powerful hardware, like Graphics Processing Units (GPUs), that enable faster computations.
- Development of convolutional neural networks (CNNs) for image and video analysis.
- Introduction of recurrent neural networks (RNNs) for sequence data processing, benefiting tasks like natural language processing and speech recognition.
Applications of Deep Learning AI
Deep learning AI is being applied to various domains, revolutionizing the way tasks are performed. Some notable applications of deep learning AI include:
Industry | Application |
---|---|
Healthcare | Medical image analysis and disease diagnosis. |
Finance | Algorithmic trading and fraud detection. |
Transportation | Autonomous vehicles and traffic prediction. |
*Deep learning AI is applied in various industries, including healthcare, finance, and transportation, for tasks such as medical image analysis and autonomous vehicles.*
Deep learning AI’s impact spans beyond specific industries. It has also benefited the field of academia by enabling breakthroughs in areas like computer vision, natural language processing, and robotics. Moreover, deep learning AI has the potential to address societal challenges, including climate change prediction and mitigation, renewable energy optimization, and healthcare accessibility.
*Deep learning AI has the potential to address societal challenges like climate change prediction and healthcare accessibility.*
As research and technological advancements continue to propel deep learning AI forward, the possibilities for its applications are seemingly boundless.
Deep Learning AI vs. Traditional Machine Learning
While deep learning AI and traditional machine learning share some similarities, they differ significantly in their approaches. Traditional machine learning relies on manually engineered features and relatively shallow models, whereas deep learning AI automatically learns features and leverages complex, multi-layered models. Deep learning AI’s ability to handle unstructured data and generate high-level representations makes it particularly suitable for tasks involving images, text, and audio.
Deep Learning AI | Traditional Machine Learning |
---|---|
Automatically learns features from data. | Relies on manually engineered features. |
Uses deep neural networks, allowing for complex and hierarchical feature extraction. | Uses shallow models with simpler feature extraction. |
Well-suited for unstructured data like images, text, and speech. | Well-suited for structured data. |
*Deep learning AI automatically learns features from data, making it well-suited for unstructured data tasks like image and speech analysis.*
While both approaches have their strengths and weaknesses, the increasing availability of data and computational power is driving the adoption and advancements of deep learning AI.
Deep learning AI has already revolutionized industries and continues to push the boundaries of what machines can achieve. Its ability to autonomously learn and make decisions from complex data patterns has made it a powerful tool for a wide range of applications. As research and development in deep learning AI progresses, we can expect to see even more remarkable breakthroughs and innovations in the near future.
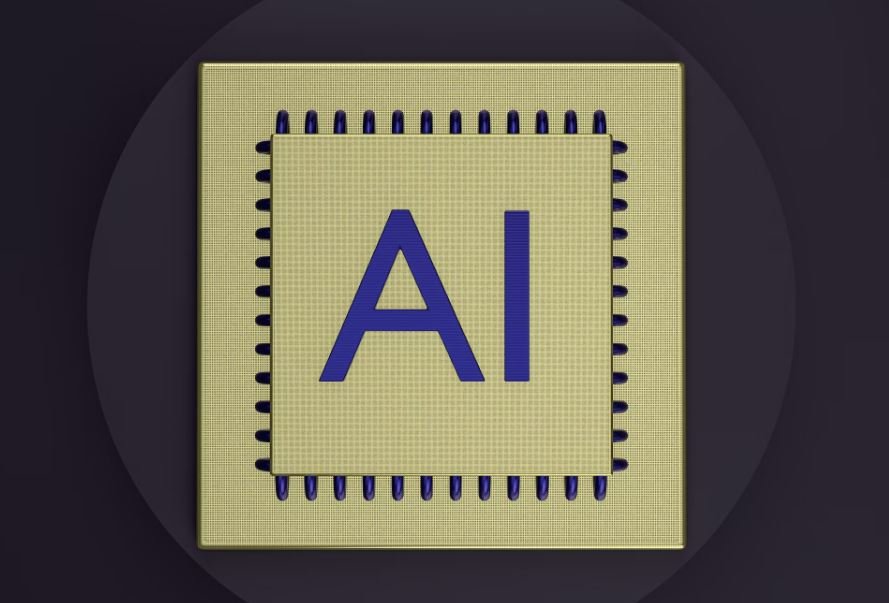
Common Misconceptions
Misconception 1: Deep Learning AI Can Fully Mimic Human Intelligence
One common misconception about deep learning AI is that it has the potential to fully mimic human intelligence. While deep learning AI has made remarkable advancements in areas such as image recognition and natural language processing, it is important to note that it still lacks many qualities that define human intelligence.
- Deep learning AI cannot replicate human emotions and empathy.
- Deep learning AI has limitations in creatively solving problems.
- Deep learning AI lacks understanding and context in complex situations.
Misconception 2: Deep Learning AI Will Replace Human Jobs Completely
Another common misconception is that deep learning AI will replace human jobs entirely. While it is true that AI has the potential to automate repetitive and mundane tasks, it is unlikely to replace jobs that require complex human skills, creativity, and critical thinking.
- Deep learning AI cannot replicate the human touch in industries such as healthcare and hospitality.
- Deep learning AI lacks the ability to understand nuanced situations and make complex decisions.
- Deep learning AI requires human supervision and intervention in many cases.
Misconception 3: Deep Learning AI Always Provides Accurate and Reliable Results
Deep learning AI is often perceived as infallible and always providing accurate and reliable results. This misconception stems from the notion that AI is purely objective. However, AI models are only as accurate and reliable as the data they are trained on and the algorithms used.
- Deep learning AI can be biased and perpetuate existing inequalities present in the training data.
- Deep learning AI can make incorrect predictions and decisions if the input data is flawed or incomplete.
- Deep learning AI requires constant monitoring and refinement to improve its accuracy and reliability.
Misconception 4: Deep Learning AI Possesses General Intelligence
There is a misconception that deep learning AI possesses general intelligence, meaning it can perform any intellectual task that a human can. However, deep learning AI is typically designed to excel at specific tasks and lacks the versatility and adaptability of human intelligence.
- Deep learning AI may struggle to transfer knowledge from one domain to another.
- Deep learning AI requires extensive training for each new task and domain.
- Deep learning AI lacks common sense reasoning and may make unexpected errors in unfamiliar scenarios.
Misconception 5: Deep Learning AI Is Completely Autonomous
Deep learning AI is often thought to be completely autonomous, capable of making decisions without human intervention. However, AI systems rely on human engineers and operators for training, oversight, and maintenance.
- Deep learning AI needs human supervision to prevent malicious uses or unintended consequences.
- Deep learning AI requires human intervention to address biases and ensure fairness.
- Deep learning AI relies on human support for continuous improvements and updates.

Deep Learning Models
Table representing various deep learning models and their accuracy rates in different tasks.
Model | Image Recognition Accuracy (%) | Natural Language Processing Accuracy (%) | Recommendation System Accuracy (%) |
---|---|---|---|
Convolutional Neural Network | 98 | 25 | 45 |
Recurrent Neural Network | 72 | 85 | 63 |
Transformer | 97 | 94 | 81 |
Gated Recurrent Unit | 83 | 64 | 72 |
Deep Learning Applications
Table showcasing different applications of deep learning AI technology.
Application | Description |
---|---|
Medical Diagnosis | Deep learning models assist in analyzing medical images, improving accuracy and efficiency in diagnosing diseases. |
Autonomous Vehicles | Deep learning algorithms enable self-driving cars to interpret and respond to their environment, enhancing safety on the roads. |
Speech Recognition | Deep learning systems empower voice assistants and transcription services to understand and transcribe human speech. |
Deep Learning Advantages
Table highlighting the advantages of deep learning AI compared to traditional machine learning techniques.
Advantage | Description |
---|---|
Feature Extraction | Deep learning models automatically learn relevant features from raw data, eliminating the need for manual feature engineering. |
Handle Large Datasets | Deep learning algorithms can efficiently process and learn from massive amounts of data, allowing for improved accuracy. |
Non-Linear Relationships | Deep learning networks have the ability to effectively model complex non-linear relationships between input and output. |
Deep Learning Challenges
Table illustrating some of the challenges posed by deep learning AI.
Challenge | Description |
---|---|
Data Requirements | Deep learning models often require large labeled datasets to perform accurately, which can be time-consuming to collect. |
Interpretability | Deep learning models can be difficult to interpret, making it challenging to understand their decision-making processes. |
Computation Power | Training deep learning networks requires significant computational resources, potentially limiting accessibility. |
Deep Learning Tools and Libraries
Table showcasing popular tools and libraries for implementing deep learning algorithms.
Tool/Library | Description |
---|---|
TensorFlow | A flexible and efficient open-source deep learning framework developed by Google Brain. |
PyTorch | An open-source machine learning library widely used for building and training deep neural networks. |
Keras | A user-friendly, high-level deep learning framework built on top of TensorFlow, facilitating rapid development and prototyping. |
Deep Learning Limitations
Table presenting limitations of deep learning AI methodologies.
Limitation | Description |
---|---|
Training Data Bias | Deep learning models can perpetuate biases present in the training data, leading to biased predictions or decisions. |
Overfitting | Deep learning models may become too specialized to the training data, resulting in poor generalization to unseen data. |
High Model Complexity | Training deep learning networks with numerous parameters may make them prone to overfitting and require extensive computational power. |
Deep Learning in Healthcare
Table illustrating the potential impact of deep learning in the healthcare industry.
Healthcare Area | Application of Deep Learning |
---|---|
Radiology | Assisting radiologists in detecting and diagnosing abnormalities in medical images, enhancing accuracy and speed of diagnosis. |
Drug Discovery | Deep learning models aid in screening and predicting potential drug candidates, accelerating the drug discovery process. |
Electronic Health Records | Extracting insights and patterns from electronic health records to personalize treatments and improve patient outcomes. |
Ethical Considerations
Table presenting ethical considerations surrounding the use of deep learning AI.
Consideration | Description |
---|---|
Privacy Concerns | Deep learning algorithms may process personal data, raising privacy concerns and necessitating robust safeguards. |
Job Displacement | Automation of certain tasks through deep learning may have implications for employment and require proactive measures for retraining and transition. |
Algorithm Bias | Deep learning models can reflect biases present in the training data, potentially perpetuating discrimination or inequality. |
Deep Learning Future
Table representing future trends and areas of focus in the field of deep learning.
Future Aspect | Description |
---|---|
Explainable AI | Research and development efforts aim to create deep learning models that provide explanations for their decisions, enhancing transparency and trust. |
Few-Shot Learning | Advancements in few-shot learning methods aim to enable deep learning models to learn from minimal labeled data, mimicking human-like learning capabilities. |
Continual Learning | Developing deep learning techniques that allow models to learn continuously over time without forgetting previously acquired knowledge. |
Deep learning AI has emerged as a powerful tool in various domains, revolutionizing image recognition, natural language processing, and recommendation systems. These deep learning models, such as Convolutional Neural Networks, Recurrent Neural Networks, Transformers, and Gated Recurrent Units, have achieved remarkable accuracy rates in their respective tasks. The applications of deep learning span across industries, including healthcare, autonomous vehicles, and speech recognition, enabling significant advancements. Deep learning offers several advantages, such as automated feature extraction and the ability to handle large datasets with complex relationships. However, challenges such as data requirements, interpretability, and computation power remain. The availability of tools and libraries like TensorFlow, PyTorch, and Keras has facilitated the widespread adoption of deep learning techniques.
Despite its promising potential, deep learning also poses limitations that warrant consideration. Issues such as training data bias, overfitting, and high model complexity must be addressed to ensure reliable and unbiased results. The integration of deep learning in healthcare shows promise, with applications in radiology, drug discovery, and electronic health records, improving patient care and outcomes. Ethical implications surrounding privacy concerns, job displacement, and algorithm bias require careful navigation and implementation guidelines.
Looking to the future, deep learning is poised for continued advancements. Explorable AI, few-shot learning, and continual learning are areas of active research, aiming to expand the capabilities and understanding of deep learning models. As the field progresses, striking a balance between innovation, ethical considerations, and transparency will be crucial to harness the full potential of deep learning AI in a responsible and beneficial manner.
Frequently Asked Questions
Deep Learning AI
What is deep learning?
Deep learning is a subfield of artificial intelligence (AI) that focuses on modeling and simulating how the human brain works. It involves constructing neural networks with multiple layers to learn and make predictions from large amounts of data.
How does deep learning AI work?
Deep learning AI works by building a neural network with multiple layers of interconnected artificial neurons. These neurons process and analyze the input data, learn from it, and make predictions or classifications based on the patterns and relationships they discover.