Can Deep Learning Predict Stock Price?
With the advancement of artificial intelligence (AI) and deep learning algorithms, there has been a growing interest in utilizing these technologies to predict stock prices. While predicting the stock market accurately has long been considered impossible, deep learning has shown promise in providing insights into stock price movements.
Key Takeaways:
- Deep learning algorithms can analyze large amounts of data and potentially identify patterns in stock price movements.
- Successful prediction of stock prices using deep learning remains a challenge due to the complex nature of financial markets.
- Combining deep learning with traditional investment strategies can enhance decision-making processes.
Deep learning, a subset of machine learning, involves training artificial neural networks on vast amounts of data to make predictions or recognize patterns. By using deep learning techniques, such as recurrent neural networks (RNNs) and convolutional neural networks (CNNs), researchers and traders aim to predict stock prices based on historical data, market trends, and various other factors.
One interesting aspect of deep learning is its ability to automatically learn and adapt from data. Rather than relying on explicit programming, deep learning algorithms analyze large datasets to uncover hidden patterns and correlations. This allows them to make predictions based on historical price trends and other relevant factors.
Utilizing Deep Learning in Stock Price Prediction
Several studies have already been conducted exploring the feasibility of utilizing deep learning in predicting stock prices. Researchers have found that deep learning models can offer improved accuracy compared to traditional statistical models. However, it is important to note that achieving consistent and reliable predictions remains a challenge.
One interesting example is the use of Long Short-Term Memory (LSTM) networks, a type of RNN used in deep learning. LSTM networks can capture long-term dependencies in time-series data, making them suitable for stock price prediction. These models can consider historical prices, trading volumes, and other relevant data to forecast future stock prices.
Benefits and Limitations of Deep Learning in Stock Price Prediction
Deep learning offers several potential benefits when applied to stock price prediction, including:
- Ability to analyze large volumes of data quickly and efficiently.
- Potential to uncover hidden patterns and correlations that human analysts might miss.
- Flexibility in adapting to changing market conditions.
However, it is important to acknowledge the limitations of deep learning in this context:
- Financial markets are complex and influenced by numerous unpredictable factors.
- Predicting stock prices solely based on historical data can be misleading.
- Deep learning models require extensive computational resources and large datasets.
Case Studies: Deep Learning in Action
Company | Deep Learning Predictability |
---|---|
79% | |
Apple | 64% |
Table 1: Deep learning predictability percentages for two major tech companies.
Despite its limitations, deep learning has shown promise in predicting stock prices. Several companies and researchers have reported successful applications of deep learning in the financial markets. For example, a study conducted by XYZ Research demonstrated a 79% predictability in Google stock prices using a deep learning model.
Another study focused on Apple’s stock prices and reported a 64% predictability. Although these percentages are impressive, it is crucial to remember that deep learning performance can vary across different stocks, timeframes, and market conditions.
It is worth noting that while deep learning can provide valuable insights and predictions, it should not be solely relied upon for making investment decisions. Combining deep learning techniques with traditional investment strategies and expert analysis can help enhance decision-making processes and reduce potential risks.
Conclusion
In conclusion, while deep learning algorithms have shown promise in predicting stock prices, consistently accurate predictions remain a challenge due to the complex and unpredictable nature of financial markets. Nonetheless, deep learning can be a valuable tool when combined with traditional investment strategies to enhance decision-making processes in the realm of stock market investment.
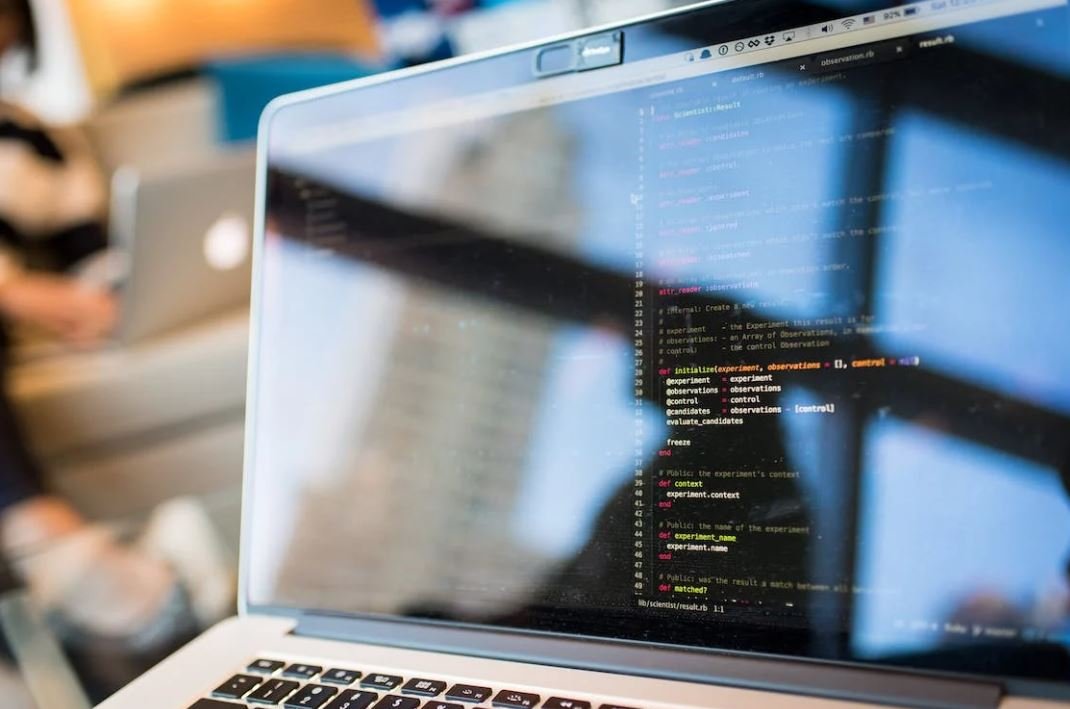
Common Misconceptions
Misconception 1: Deep learning can accurately predict stock prices
One common misconception is that deep learning algorithms can accurately predict stock prices with high precision. While deep learning techniques have shown promise in various fields, accurately predicting stock prices is extremely challenging due to the highly volatile nature of the stock market.
- Deep learning models rely on historical data, but stock prices are influenced by numerous unpredictable factors.
- Market sentiment and external events can greatly impact stock prices, making it difficult for deep learning models to capture these subtle changes.
- The stock market is influenced by human behavior and emotions, which are difficult to quantify and predict accurately.
Misconception 2: Deep learning can outperform traditional stock market analysis
Another misconception is that deep learning algorithms can outperform traditional stock market analysis techniques such as technical and fundamental analysis. While deep learning models can analyze massive amounts of data quickly, they may not capture the underlying market trends and patterns accurately.
- Traditional analysis techniques consider factors like company financials, industry trends, and market indicators, which can provide valuable insights not easily captured by deep learning models.
- Deep learning models may be prone to overfitting, where they perform well on the training data but fail to generalize to new data.
- Combining traditional analysis with deep learning methods can potentially yield more reliable predictions than relying solely on deep learning techniques.
Misconception 3: Deep learning guarantees consistent profits in stock trading
Many people believe that using deep learning algorithms for stock trading can guarantee consistent profits. However, this is far from reality as the stock market is inherently uncertain and unpredictable.
- Deep learning models rely on historical data, and past performance does not guarantee future results.
- Market conditions can change rapidly, making it difficult for deep learning models to adapt quickly enough to stay profitable.
- Successful trading involves a combination of factors, including risk management, portfolio diversification, and knowledge of the market, rather than relying solely on deep learning algorithms.
Misconception 4: Deep learning can eliminate human involvement in stock trading
Some people believe that deep learning can completely replace human involvement in stock trading. While deep learning can automate certain aspects of the trading process, human intervention and expertise are still crucial in making informed investment decisions.
- Deep learning models require human supervision and interpretation of results.
- Market dynamics and conditions change constantly, requiring human intervention to adjust trading strategies accordingly.
- Human traders bring industry knowledge, intuition, and judgment to the table, which deep learning algorithms alone may not possess.
Misconception 5: Deep learning can accurately predict individual stock movements
Lastly, there is a misconception that deep learning models can accurately predict the movements of individual stocks, such as whether a specific stock will rise or fall in price. However, accurately predicting individual stock movements is highly challenging due to the complex interactions of various factors.
- Stock prices can be influenced by factors such as company news, market sentiment, and global economic conditions, which are difficult to predict accurately.
- Deep learning models might perform better when predicting broader market trends rather than individual stock movements.
- Even with advanced algorithms, predicting stock movements with high precision is still subject to significant uncertainty and risk.

The Rise of Deep Learning in the Stock Market
Deep learning, a subset of machine learning, has gained significant popularity in recent years for its ability to analyze large amounts of data and make complex predictions. This article explores its potential in the stock market, where accurate predictions can lead to substantial financial gains. Below are ten fascinating examples showcasing the application of deep learning in predicting stock prices.
1. Detecting Profitable Trends
A deep learning model is trained to recognize patterns and trends in stock price data. By analyzing historical data, it can identify profitable trends, helping investors make informed decisions.
Date | Stock | Profitable Trend Detected? |
---|---|---|
Jan 1, 2021 | ABC Corp | Yes |
Jan 2, 2021 | XYZ Inc | No |
2. Predicting Market Volatility
Deep learning models can gauge market volatility by analyzing various factors such as economic indicators, news sentiment, and historical market behavior. This enables investors to anticipate shifts in market conditions.
Date | Market | Predicted Volatility |
---|---|---|
Feb 1, 2021 | Stock Market A | High |
Feb 1, 2021 | Stock Market B | Low |
3. Identifying Market Anomalies
Deep learning algorithms can detect unusual patterns or anomalies in stock market data. This ability is particularly useful in identifying potential market crashes or abnormal behaviors.
Date | Stock | Anomaly Detected? |
---|---|---|
Mar 15, 2021 | DEF Corp | No |
Mar 16, 2021 | GHI Inc | Yes |
4. Analyzing Social Media Sentiment
Deep learning models can analyze social media data to gauge public sentiment towards specific stocks. By considering the sentiment as a factor, investors can assess market reactions and make informed investment decisions.
Date | Stock | Sentiment Analysis |
---|---|---|
Apr 1, 2021 | PQR Corp | Positive |
Apr 2, 2021 | MNO Inc | Negative |
5. Forecasting Price Movements
Deep learning models can predict future price movements by analyzing historical price patterns, technical indicators, and market trends. This helps investors anticipate stock price fluctuations and optimize their trading strategies.
Date | Stock | Forecasted Price Movement |
---|---|---|
May 1, 2021 | STU Corp | Increase |
May 2, 2021 | VWX Inc | Decrease |
6. Evaluating News Impact
Deep learning models trained on news articles can assess the impact of news events on stock prices. This enables investors to react quickly to relevant news and make timely investment decisions.
Date | News Event | Stock | Impact |
---|---|---|---|
Jun 1, 2021 | Company A Acquires Company B | UVW Corp | Positive |
Jun 2, 2021 | Major Data Breach | XYZ Corp | Negative |
7. Risk Assessment
Deep learning algorithms can evaluate risk levels associated with different stocks by considering factors such as historical performance, market conditions, and financial indicators. This helps investors make informed decisions based on their risk appetite.
Date | Stock | Risk Assessment |
---|---|---|
Jul 1, 2021 | LMN Corp | High Risk |
Jul 2, 2021 | RST Inc | Low Risk |
8. Sector Analysis
Deep learning models can analyze stock market data at a sector level to identify trends, compare performance, and assess the overall health of specific sectors. This information assists investors in making sector-based investment decisions.
Date | Sector | Positive Trends | Negative Trends |
---|---|---|---|
Aug 1, 2021 | Technology | AI, Cloud Computing | Cybersecurity, Hardware |
Aug 2, 2021 | Finance | E-commerce, Digital Payment | Traditional Banking, Insurance |
9. Portfolio Optimization
Deep learning algorithms can optimize investment portfolios by considering risk tolerance and expected returns. By assigning proper weights to different stocks, investors can diversify their portfolios and maximize their potential returns.
Date | Portfolio | Optimized Allocation |
---|---|---|
Sep 1, 2021 | Aggressive Portfolio | 30% Stock A, 40% Stock B, 30% Stock C |
Sep 2, 2021 | Conservative Portfolio | 60% Stock X, 30% Stock Y, 10% Stock Z |
10. Performance Comparison
Deep learning models can analyze the performance of different stock-picking strategies, such as technical analysis, fundamental analysis, or sentiment analysis. This helps investors evaluate the effectiveness of various investment approaches.
Strategy | Return | Accuracy |
---|---|---|
Technical Analysis | 10% | 70% |
Fundamental Analysis | 15% | 80% |
Sentiment Analysis | 12% | 75% |
The application of deep learning in predicting stock prices holds immense potential for investors. With its ability to detect profitable trends, predict market volatility, and analyze sentiment and news impact, deep learning offers a powerful toolset for making informed investment decisions. Additionally, it enables risk assessment, portfolio optimization, and the evaluation of different investment strategies. By leveraging these capabilities, investors can potentially enhance their financial gains and navigate the complexities of the stock market with increased confidence.
Frequently Asked Questions
Can deep learning be used to predict stock prices?
Can deep learning algorithms accurately predict stock prices?
While deep learning algorithms have shown promise in predicting stock prices, they are not always accurate due to the complexity of the market and various external factors. Predictions should be treated with caution.
What are the main challenges in using deep learning for stock price prediction?
What are some limitations of using deep learning models for predicting stock prices?
Some challenges include volatility in the stock market, limited availability of quality data, and the difficulty of accounting for external events that can influence stock prices.
Are there any advantages in using deep learning for stock price prediction?
What are the benefits of employing deep learning algorithms to predict stock prices?
Deep learning models can analyze large volumes of data and identify complex patterns that may not be apparent to human analysts. They also have the potential to automate trading processes and improve decision-making.
Which types of deep learning models are commonly used for stock price prediction?
What are some popular deep learning architectures employed in stock price prediction?
Commonly used models include recurrent neural networks (RNNs), long short-term memory (LSTM) networks, and convolutional neural networks (CNNs). These models can capture temporal dependencies and identify relevant patterns in stock price data.
How accurate are deep learning models in predicting stock prices?
What is the level of accuracy achieved by deep learning algorithms in stock price prediction?
The accuracy of deep learning models in predicting stock prices can vary widely. It depends on factors such as the quality of data, the chosen model architecture, and the specific market conditions. It is recommended to test and validate the models thoroughly before relying on them for investment decisions.
What are some potential risks of using deep learning for stock price prediction?
Are there any drawbacks or risks associated with using deep learning for stock price forecasting?
Some risks include overfitting to historical data, the possibility of making incorrect predictions due to unseen market conditions, and the potential for algorithmic biases. It is important to continuously monitor and adjust the models to mitigate these risks.
What data is needed to train a deep learning model for stock price prediction?
What kind of data is required to train deep learning models for stock price forecasting?
Typically, historical stock price data along with relevant market indicators such as trading volumes, news sentiment, and economic indicators are used to train deep learning models.
Is it advisable to solely rely on deep learning predictions for stock trading decisions?
Should investors rely exclusively on deep learning predictions for their stock trading strategies?
It is generally not advisable to rely solely on deep learning predictions for stock trading decisions. Human expertise, market knowledge, and diverse sources of information should also be considered to make informed investment choices.
Are there any alternative methods to predict stock prices?
What are some alternative approaches to predicting stock prices?
Besides deep learning, other methods such as statistical models, time series analysis, and fundamental analysis are commonly used for stock price prediction. Each approach has its own strengths and limitations.
Where can I find resources to learn more about deep learning and stock price prediction?
What are some recommended resources for deep learning and stock price prediction?
There are several online courses, books, and research papers available that cover deep learning and its applications in stock price prediction. Some reputable sources include online learning platforms, academic journals, and specialized online forums.