Are Machine Learning Artificial Intelligence
Machine learning and artificial intelligence (AI) are often used interchangeably, but are they really the same thing? While they are related concepts, there are important distinctions between the two. Understanding these differences can help us better grasp the capabilities and limitations of these technologies.
Key Takeaways:
- Machine learning and artificial intelligence are related concepts but have distinct differences.
- Machine learning refers to the ability of a system to learn and improve from experience.
- Artificial intelligence encompasses a broader scope, including machines that can mimic human intelligence.
**Machine learning** is a form of AI that enables computers to learn and make predictions or decisions without being explicitly programmed. It relies on algorithms and statistical models to analyze vast amounts of data and find patterns or correlations. *This allows machines to improve their performance over time as they gather more data.*
**Artificial intelligence**, on the other hand, goes beyond the realm of machine learning. It refers to machines or systems that exhibit **human-like intelligence**. This includes capabilities such as natural language processing, computer vision, problem-solving, and decision-making. *These machines can simulate human cognitive abilities and perform tasks that traditionally require human intelligence.*
Machine Learning vs. Artificial Intelligence
While **machine learning** is a subset of artificial intelligence, it is important to understand the distinctions between the two:
- Approach: Machine learning focuses on the development of algorithms and statistical models to enable machines to learn and improve from data. Artificial intelligence, on the other hand, aims to create systems that can simulate human intelligence and perform tasks that typically require human capabilities.
- Capabilities: Machine learning enables machines to automatically learn patterns and make predictions or decisions based on data. AI, on the other hand, encompasses a broader range of capabilities such as natural language processing, computer vision, and complex problem-solving.
- Data Dependency: Machine learning heavily relies on large volumes of labeled data to train models and make predictions. AI systems can operate with or without large datasets, as they are designed to mimic human intelligence rather than relying solely on data.
The Relationship Between Machine Learning and Artificial Intelligence
Machine learning is an integral part of many AI systems, as it enables machines to learn from experience and adapt their behavior accordingly. **Artificial intelligence** systems often leverage machine learning algorithms to process and analyze data, which then guides their decision-making and problem-solving abilities. *This symbiotic relationship between machine learning and artificial intelligence enables the development of intelligent systems that continually improve their performance.*
Applications of Machine Learning and Artificial Intelligence
Both machine learning and artificial intelligence have numerous applications across various industries. Here are a few examples:
- Healthcare: Machine learning algorithms can analyze medical data to detect patterns and diagnose diseases. Artificial intelligence systems can assist in surgical procedures or provide personalized treatment recommendations.
- Finance: Machine learning is used in fraud detection systems and credit scoring models. AI-powered chatbots can provide customer support and personalized financial advice.
- Transportation: Machine learning is employed in route optimization and predictive maintenance for vehicles. Autonomous cars and drones rely on AI for navigation and decision-making.
Machine Learning and Artificial Intelligence in Numbers
Statistic | Value |
---|---|
Global AI market size in 2020 | $62.4 billion |
Applications of machine learning |
---|
Healthcare |
Finance |
Transportation |
Key AI technologies | Market Growth Rate |
---|---|
Natural Language Processing | 20.7% |
Computer Vision | 17.3% |
Machine Learning | 43.5% |
The Future of Machine Learning and Artificial Intelligence
The potential of machine learning and artificial intelligence is vast and their future prospects are promising. As these technologies continue to evolve, we can expect to see advancements in various fields such as healthcare, finance, and robotics. With ongoing research and development, **the boundaries of what machines can achieve will continue to be pushed**, bringing us closer to a world where intelligent machines are integrated into our everyday lives.
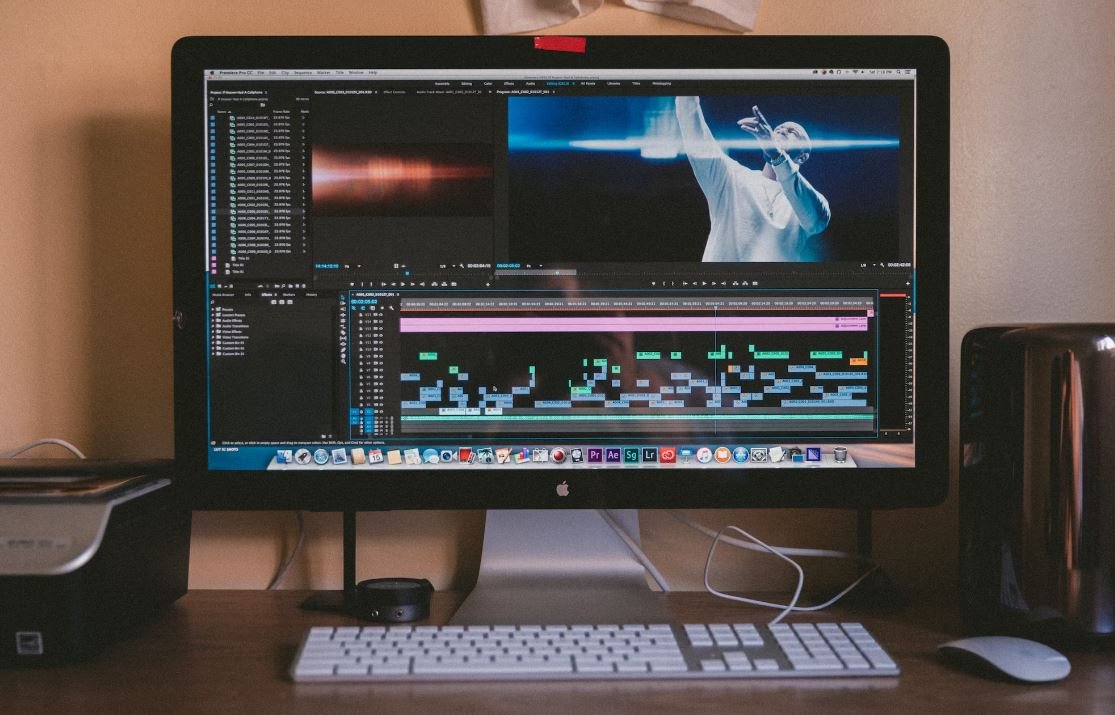
Common Misconceptions
Paragraph 1: Machine Learning and Artificial Intelligence
One common misconception is that machine learning and artificial intelligence are the same thing. While they are related, they are not interchangeable terms.
- Machine learning is a subset of artificial intelligence.
- Artificial intelligence refers to any system or program that can perform tasks that would typically require human intelligence.
- Machine learning focuses on the development of algorithms and statistical models that allow the system to improve its performance on a specific task through experience.
Paragraph 2: Machine Learning Replacing Humans
Another misconception is that machine learning will completely replace human workers in various industries. While machine learning has the potential to automate certain tasks, it is unlikely to replace humans entirely.
- Machine learning can assist and enhance human capabilities.
- Humans are still necessary for critical thinking, creativity, and decision-making in complex situations.
- Machine learning can help humans by performing repetitive and mundane tasks, freeing up time for more value-added activities.
Paragraph 3: Machine Learning as a Magic Solution
Many people perceive machine learning as a magical solution that can solve any problem. However, it has its limitations and cannot solve everything.
- Machine learning requires quality input data to produce accurate results.
- Models can be biased and perpetuate any existing biases in the input data.
- Machine learning cannot replace the need for domain expertise and understanding of the problem being addressed.
Paragraph 4: Machine Learning Always Predicts the Future
There is a misconception that machine learning algorithms can always predict the future with absolute certainty. In reality, machine learning predicts patterns based on historical data and is subject to uncertainties.
- Machine learning predictions are probabilistic, not deterministic.
- Models can be influenced by outliers, noisy data, or unseen patterns.
- Uncertainties in predictions are inherent and should be considered when making decisions based on machine learning algorithms.
Paragraph 5: Machine Learning Implementation is Easy
Some people believe that implementing machine learning is a straightforward task. However, it requires careful planning, data preparation, and expertise.
- Machine learning implementation involves understanding the problem, choosing appropriate algorithms, and data preprocessing.
- Data collection, cleaning, and feature engineering are crucial steps in preparing the data for machine learning models.
- Machine learning requires regular monitoring and updating to adapt to changing environments and data patterns.
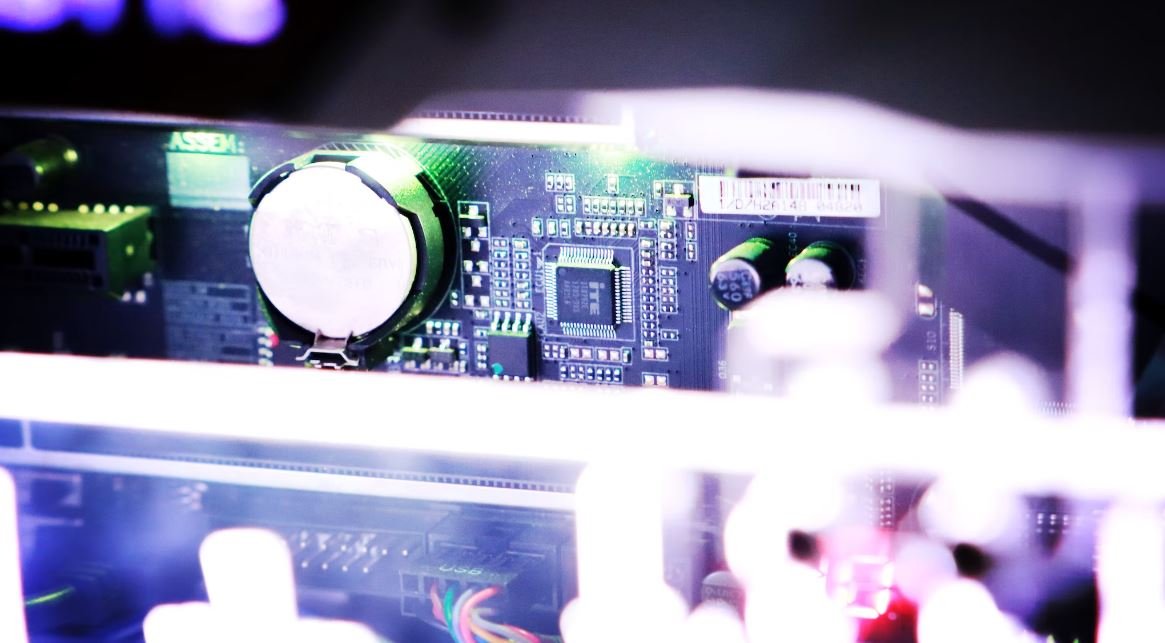
Machine Learning Applications in Everyday Life
Machine learning and artificial intelligence have become integral parts of our everyday lives. From recommendations on streaming platforms to voice recognition on our smartphones, machine learning algorithms are constantly at work. This article explores 10 fascinating applications of machine learning in various fields.
1. Predictive Analytics in Finance
Machine learning models are used in finance to analyze historical data, identify patterns, and predict future market trends. By considering factors such as market fluctuations, economic indicators, and investor sentiment, these models help in making informed investment decisions.
Application | Data Inputs | Benefits |
---|---|---|
Stock Market Prediction | Historical stock prices, news sentiment | Improved portfolio management |
Credit Risk Assessment | Borrower’s financial history, credit records | Enhanced decision-making for loans |
2. Image Classification in Healthcare
Machine learning algorithms are employed in healthcare to assist in the interpretation of medical images, aiding in the detection and diagnosis of diseases. This table showcases some notable applications of image classification in healthcare.
Application | Image Inputs | Advantages |
---|---|---|
Cancer Detection | Mammography, histopathological images | Early detection and higher accuracy |
Retinal Disease Diagnosis | Retinal scans | Timely identification of eye diseases |
3. Natural Language Processing in Chatbots
Chatbots powered by natural language processing (NLP) enable effective interaction between humans and computers. Whether it’s customer support or voice assistants, NLP plays a vital role in improving user experience.
Application | Features | Benefits |
---|---|---|
Virtual Customer Support | Language understanding, sentiment analysis | 24/7 availability, improved query resolution |
Voice-controlled Assistants | Speech recognition, language generation | Hands-free task management |
4. Fraud Detection in Banking
Machine learning models are utilized in the banking sector for fraud detection, helping prevent financial losses and secure customer accounts. This table highlights some instances of machine learning usage in banking fraud detection.
Application | Data Features | Advantages |
---|---|---|
Credit Card Fraud | Transaction history, anomaly detection | Early detection and reduced financial losses |
Identity Theft | User behavior patterns, biometric data | Improved customer protection |
5. Recommendation Systems in E-commerce
Machine learning-based recommendation systems personalize online shopping experiences by suggesting products based on user behavior and past purchases. This table explores different types of recommendation systems in e-commerce.
System | Data Inputs | Benefits |
---|---|---|
Collaborative Filtering | User preferences, ratings | Increased customer satisfaction |
Content-Based Filtering | Item attributes, user preferences | Improved discovery of new products |
6. Autonomous Vehicles and Traffic Management
Self-driving cars and advanced traffic management systems utilize machine learning to improve road safety and optimize traffic flow. The table below depicts examples of machine learning applications in autonomous vehicles.
Application | Data Inputs | Advantages |
---|---|---|
Object Recognition | Camera feeds, sensor data | Enhanced road safety |
Route Planning | Traffic data, historical patterns | Efficient navigation and reduced congestion |
7. Personalized Healthcare Recommendations
Machine learning algorithms can analyze personal health data to provide tailored recommendations and guidance for disease prevention and management. The following table outlines examples of personalized healthcare recommendations.
Recommendation Type | Data Inputs | Benefits |
---|---|---|
Dietary Suggestions | Individual dietary habits, medical records | Improved nutrition and healthier lifestyle |
Activity Planning | Activity tracker data, health goals | Enhanced physical well-being |
8. Sentiment Analysis in Social Media
Sentiment analysis powered by machine learning allows businesses to gain insights from social media posts and analyze public opinions and user sentiment. The table presents different applications of sentiment analysis in social media.
Application | Data Sources | Advantages |
---|---|---|
Brand Reputation Management | Customer reviews, social media posts | Early detection of negative feedback or potential crises |
Trend Analysis | Hashtag usage, online discussions | Identifying emerging trends |
9. Speech Recognition in Transcription Services
Machine learning algorithms enable accurate and efficient transcription services by converting spoken language into written text. Explore the different applications of speech recognition in transcription services through the following table.
Application | Data Features | Benefits |
---|---|---|
Speech-to-Text Conversion | Audio recordings, language models | Time-saving and increased productivity |
Language Translation | Spoken and written text in different languages | Improved communication across language barriers |
10. Energy Optimization in Smart Grids
Machine learning techniques facilitate energy optimization in smart grid systems by predicting energy demand, optimizing distribution, and promoting energy efficiency. The examples below demonstrate machine learning applications in smart grids.
Application | Data Inputs | Advantages |
---|---|---|
Load Forecasting | Historical energy usage, weather data | Efficient energy distribution |
Energy Theft Detection | Anomaly detection, consumption patterns | Reduced revenue losses for utilities |
The applications of machine learning and artificial intelligence are vast and continue to expand. From finance to healthcare, these technologies revolutionize how industries operate. As technology progresses, the potential for further innovation and improvements is incredibly promising.
Frequently Asked Questions
What is machine learning?
Machine learning is a subset of artificial intelligence (AI) that focuses on teaching machines to learn from data and improve their performance over time without being explicitly programmed. It involves using algorithms and statistical models to enable computers to make accurate predictions or decisions.
How does machine learning work?
Machine learning works by training models using large amounts of data. The model learns patterns and relationships in the data and uses this knowledge to make predictions or perform specific tasks. The process involves collecting, preprocessing, and selecting relevant features from the data, choosing an appropriate algorithm, training the model using the data, and evaluating its performance.
What are the types of machine learning?
There are several types of machine learning, including supervised learning, unsupervised learning, semi-supervised learning, reinforcement learning, and deep learning. Supervised learning involves training a model with labeled examples, unsupervised learning involves finding patterns in unlabeled data, semi-supervised learning combines both labeled and unlabeled data, reinforcement learning uses rewards and punishments to train models, and deep learning involves training deep neural networks with multiple hidden layers.
What is artificial intelligence?
Artificial intelligence (AI) is a branch of computer science that aims to create intelligent machines that can perform tasks that typically require human intelligence. It encompasses various techniques, including machine learning, natural language processing, computer vision, and robotics, to simulate human-like intelligence in computers.
What are the applications of machine learning in AI?
Machine learning plays a crucial role in various AI applications. It is used for image and speech recognition, natural language processing, recommendation systems, fraud detection, autonomous vehicles, predictive analytics, healthcare diagnostics, and many other domains. Machine learning algorithms enable AI systems to learn from data, make accurate predictions, and automate complex tasks.
What are the challenges in machine learning and AI?
Machine learning and AI face several challenges, including the need for large quantities of high-quality labeled data, potential biases in data and algorithms, interpretability and transparency of models, ethical considerations, scalability, and security concerns. Additionally, keeping up with the rapid advancements and changing landscape of these fields requires continuous learning and adaptation.
How can machine learning and AI benefit businesses?
Machine learning and AI offer numerous benefits to businesses. They can help automate repetitive tasks, improve decision-making, optimize resource allocation, enhance customer experience, increase efficiency and productivity, enable personalized recommendations and marketing campaigns, detect anomalies and fraud, and enable predictive maintenance. By leveraging machine learning and AI, businesses can gain a competitive advantage and unlock new opportunities.
What skills are required for a career in machine learning and AI?
A career in machine learning and AI requires a strong foundation in mathematics, statistics, and programming. It is beneficial to have knowledge of linear algebra, calculus, probability, and algorithms. Proficiency in a programming language like Python or R, familiarity with machine learning libraries/frameworks, and understanding of data preprocessing, feature engineering, model evaluation, and deployment are essential. Additionally, critical thinking, problem-solving, and creativity are valuable skills in this field.
What are the future prospects of machine learning and AI?
Machine learning and AI have a promising future with vast potential. Advancements in these fields are driving innovation across industries. The integration of machine learning and AI into various applications and systems is expected to continue growing. However, there are also concerns surrounding AI ethics, privacy, and job displacement. As the technology matures, it will be crucial to address these challenges and ensure responsible and ethical development and deployment of AI systems.
Where can I learn machine learning and AI?
There are several online platforms, universities, and educational institutions that offer courses and programs in machine learning and AI. Some popular resources include Coursera, edX, Udacity, Stanford University, Massachusetts Institute of Technology (MIT), and Carnegie Mellon University. Additionally, there are numerous books, tutorials, and open-source libraries available to learn and practice machine learning and AI.