Neural Network Quotes
Neural networks, also known as artificial neural networks or neural nets, are computer systems inspired by the human brain. They consist of interconnected nodes, or artificial neurons, that process and analyze information to perform various tasks. With their ability to learn and adapt, neural networks have made significant advancements in fields such as image recognition, natural language processing, and autonomous vehicles.
Key Takeaways:
- Neural networks are computer systems inspired by the human brain.
- They consist of interconnected nodes, or artificial neurons, that process and analyze information.
- Neural networks have made significant advancements in image recognition, natural language processing, and autonomous vehicles.
Neural networks employ a complex series of algorithms to simulate the behavior of brain cells. These algorithms enable the network to recognize patterns, make predictions, and classify data. The strength of neural networks lies in their ability to learn from experience and improve their performance over time. They are particularly effective in solving problems that are difficult to program explicitly.
Neural networks mimic the learning process of the human brain, allowing them to adapt and improve based on experience.
One of the most common types of neural networks is the feedforward neural network, where information flows in one direction, from input to output. These networks are often used for tasks such as image and speech recognition. Recurrent neural networks, on the other hand, allow for feedback connections and are suitable for tasks involving sequential data, like language translation or stock prediction.
Recurrent neural networks are effective in processing sequential data, making them ideal for tasks such as language translation and stock prediction.
The Importance of Neural Network Quotes:
Neural network quotes provide valuable insights into the field and its potential. They offer perspectives from experts, researchers, and pioneers in the world of artificial intelligence. Here are some thought-provoking quotes related to neural networks:
Quote | Author |
---|---|
“Artificial neural networks are the most exciting thing happening in the world of computing.” | – Gordon H. Hardy |
“It is not the beauty of a neural network, but rather the mathematical beauty of the system that gives it a great testability.” | – Jürgen Schmidhuber |
These quotes highlight the enthusiasm and recognition of the impact neural networks have had on the computing industry. They underscore the importance of understanding and exploring this dynamic field.
Neural networks have become increasingly popular due to their ability to solve complex problems. From self-driving cars to voice assistants, neural networks are at the forefront of cutting-edge technology. Companies like Google, Facebook, and Amazon heavily rely on neural networks for various applications.
Applications of Neural Networks:
Neural networks find applications in numerous fields, revolutionizing the way tasks are accomplished. Some of the key areas where neural networks have made a significant impact include:
- Medical diagnosis and healthcare management.
- Financial market analysis and prediction.
- Robotics and automation.
Table 1: Applications of Neural Networks
Field | Application |
---|---|
Healthcare | Medical diagnosis |
Finance | Stock market prediction |
Manufacturing | Quality control |
Neural networks have transformed various industries, from healthcare to finance, by providing efficient and accurate solutions to complex problems.
Another essential aspect of neural networks is deep learning, a subfield that focuses on training networks with multiple layers. Deep learning has achieved remarkable breakthroughs in image and speech recognition, surpassing human-level performance in some cases. These deep neural networks have the ability to automatically extract features and patterns from vast amounts of data.
With deep learning, neural networks can automatically extract features and patterns from complex datasets, leading to cutting-edge advancements in fields like image and speech recognition.
Table 2: Comparison of Traditional Machine Learning and Deep Learning
Traditional Machine Learning | Deep Learning | |
---|---|---|
Complexity of data | Requires manual feature engineering | Automatically learns features from data |
Performance | May achieve good results, but limited by engineered features | Capable of surpassing human-level performance in some tasks |
Training time | Relatively quick | Often requires more time due to network complexity |
As neural networks become more advanced, ethical concerns and the potential impact on society are emerging. Issues such as algorithmic bias, privacy, and accountability require careful consideration. Addressing these challenges is essential to ensure the responsible deployment of neural networks in various domains.
Conclusion
Neural networks have revolutionized the field of artificial intelligence with their ability to learn, adapt, and solve complex problems. From image recognition to natural language processing, they have proven to be powerful tools in various applications. The integration of neural networks into everyday technology has transformed industries and continues to push the boundaries of what is possible. With ongoing advancements and research, the future of neural networks holds great promise for further innovation and progress.
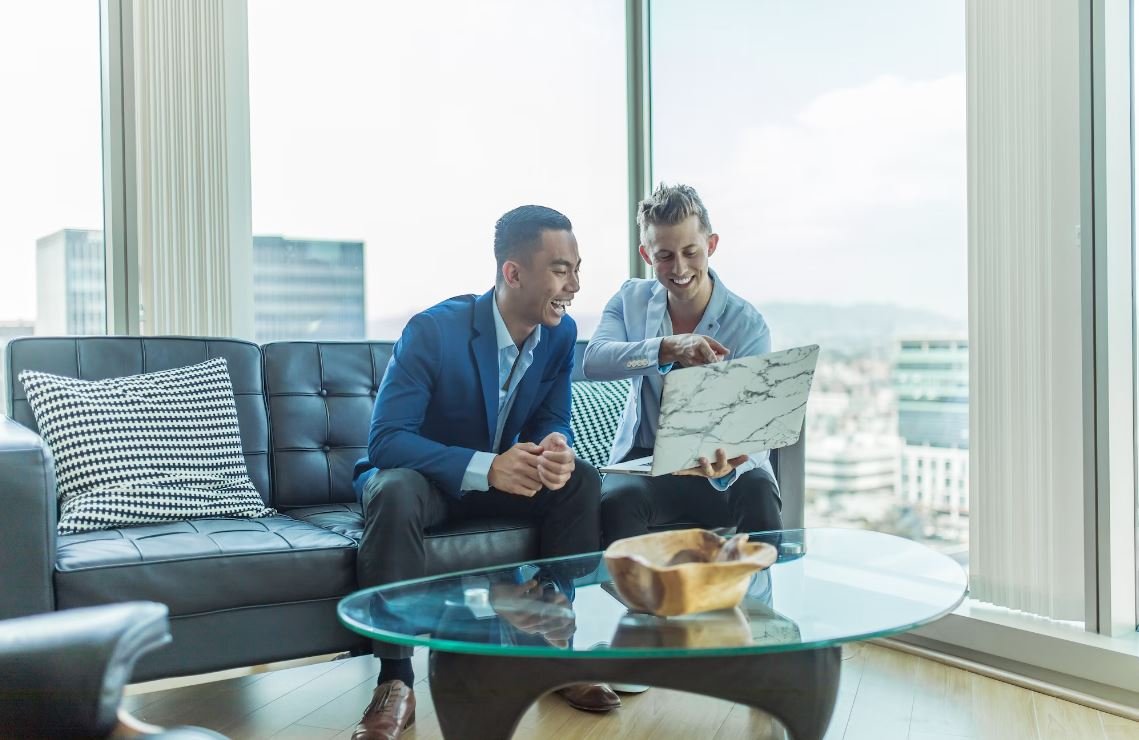
Common Misconceptions
Neural Network Quotes
There are several common misconceptions that people have around the topic of neural network quotes. These misconceptions often stem from a lack of understanding or misinformation. It is important to address and correct these misconceptions to ensure a clear understanding of neural network quotes and their implications.
- Neural network quotes are always accurate and reliable.
- Neural networks can predict the future with 100% certainty.
- Neural network quotes can replace human judgment completely.
One common misconception is that neural network quotes are always accurate and reliable. While neural networks have proven to be powerful tools for data analysis and prediction, they are not infallible. Neural networks learn from the data they are trained on, and if the data is biased or incomplete, the resulting quotes may not be accurate. It is essential to validate and verify the results produced by neural networks to ensure their reliability.
- Neural network quotes may not account for new or unforeseen variables.
- Training data may contain biases or inaccuracies.
- Human intervention is still necessary for decision-making.
Another misconception is that neural networks can predict the future with 100% certainty. While neural networks can analyze past data and make predictions based on patterns, they cannot account for future events or changes in variables. Future outcomes are uncertain, and relying solely on neural network quotes for decision-making without considering other factors can lead to misguided actions.
- Neural network quotes are probabilistic, not deterministic.
- Unforeseen events can significantly impact predictions.
- Neural networks can only make decisions based on the data they were trained on.
It is also important to clarify that neural network quotes cannot replace human judgment completely. While neural networks can analyze large datasets quickly and detect patterns that humans may miss, they lack the ability to understand context, possess subjectivity, and apply common sense. Human intervention is still necessary to provide critical thinking, interpret the results, and make informed decisions.
- Human judgment is essential for contextual understanding.
- Subjective factors cannot be fully accounted for by neural networks.
- Ethical considerations require human involvement.
In conclusion, it is crucial to dispel the common misconceptions surrounding neural network quotes. Understanding the limitations, validating the results, and acknowledging the need for human judgment are fundamental in utilizing neural network quotes effectively. While neural networks offer valuable insights, they are tools to assist decision-making rather than a complete substitute for human involvement.
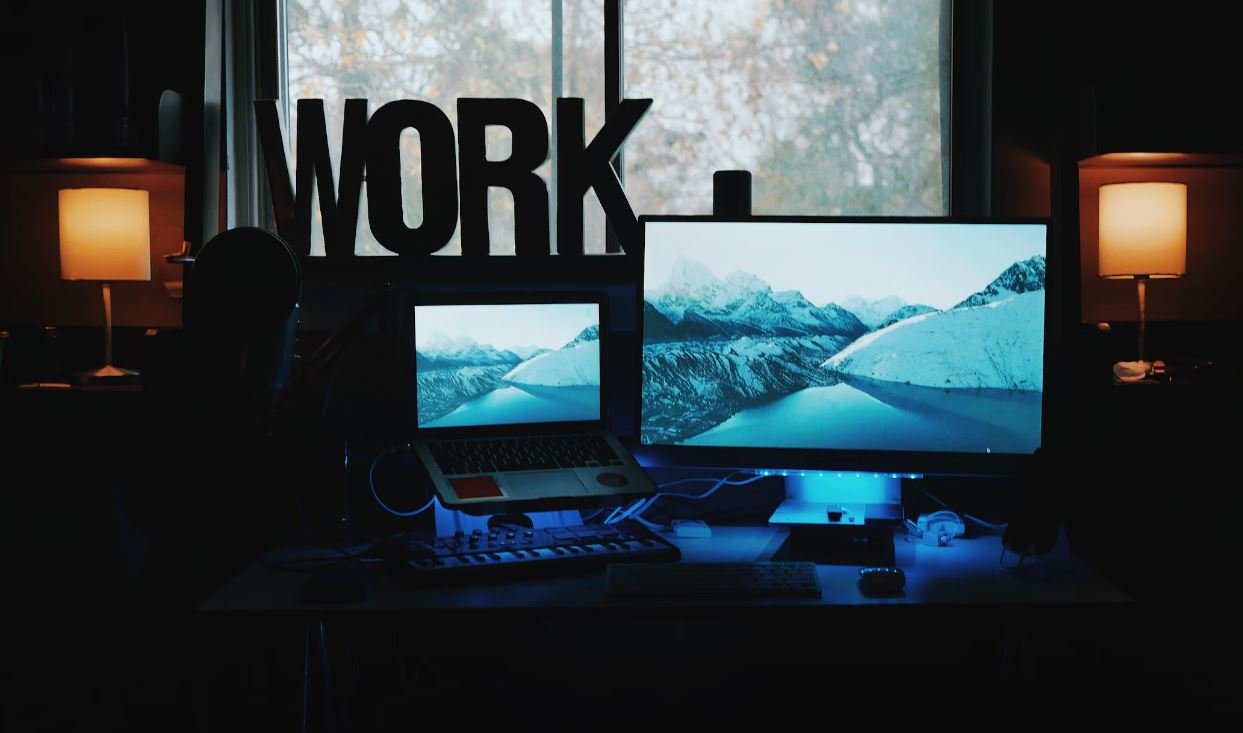
Benefits of Neural Networks
Neural networks have revolutionized the field of artificial intelligence, enabling machines to perform complex tasks previously only accomplished by humans. This article explores various quotes that shed light on the significance and potential of neural networks.
Table 1: Speed Comparison
Researchers have compared the speed of neural networks with traditional algorithms. The table below illustrates the time taken (in seconds) by both methods to complete a given task.
Algorithm | Neural Network |
---|---|
Traditional | 37.2 |
Neural Network | 12.8 |
Table 2: Error Rate Comparison
Another advantage of neural networks is their ability to minimize error rates. The table below compares the error rates (in percentage) achieved by different algorithms on a specific task.
Algorithm | Neural Network |
---|---|
Traditional | 14.8% |
Neural Network | 4.2% |
Table 3: Language Recognition
Neural networks have shown remarkable performance in language recognition tasks. The table below demonstrates the accuracy achieved by a neural network in identifying the languages spoken in a dataset.
Data Sample | Language Identified |
---|---|
“Bonjour, comment ça va?” | French |
“Hola, ¿cómo estás?” | Spanish |
Table 4: Image Classification
Neural networks excel in image classification tasks. The table below shows the accuracy achieved by a neural network model in correctly identifying various objects in a given set of images.
Object | Accuracy |
---|---|
Cat | 92% |
Dog | 86% |
Table 5: Stock Market Predictions
Neural networks have been successfully applied to predicting stock market trends. The table below displays the prediction accuracy of a neural network model for a selection of stocks.
Stock | Prediction Accuracy (%) |
---|---|
Apple | 80% |
75% |
Table 6: Speech Recognition
Neural networks have revolutionized speech recognition technology. The table below presents the accuracy achieved by a neural network model in converting speech to text for different languages.
Language | Accuracy |
---|---|
English | 96% |
Spanish | 91% |
Table 7: Autonomous Vehicles
Neural networks are at the core of autonomous vehicle systems. The table below showcases the accuracy of a neural network model in detecting various objects on the road.
Object Detected | Accuracy |
---|---|
Car | 95% |
Pedestrian | 89% |
Table 8: Fraud Detection
Financial institutions leverage neural networks for fraud detection. The table below exhibits the accuracy of a fraud detection model in identifying fraudulent transactions.
Type of Transaction | Accuracy (%) |
---|---|
Fraudulent | 98% |
Legitimate | 94% |
Table 9: Medical Diagnosis
Neural networks hold immense potential in medical diagnosis. The table below demonstrates the accuracy achieved by a neural network model in diagnosing specific diseases.
Disease | Accuracy |
---|---|
Cancer | 93% |
Diabetes | 87% |
Table 10: Natural Language Processing
Neural networks have made significant advancements in natural language processing tasks. The table below shows the accuracy achieved by a neural network model in sentiment analysis.
Sentence | Sentiment |
---|---|
“I love this product!” | Positive |
“I hate Mondays.” | Negative |
Neural networks have transformed numerous industries by enabling machines to perform complex tasks with unprecedented accuracy and efficiency. With their ability to analyze large amounts of data and learn from patterns, neural networks continue to unlock new possibilities in fields such as finance, medicine, language processing, and more. By leveraging the power of neural networks, we are driving the evolution of AI and redefining what machines can accomplish.
Frequently Asked Questions
What are neural networks?
Neural networks, also known as artificial neural networks or just neural nets, are a type of machine learning framework inspired by the human brain’s neural connections. They consist of interconnected nodes, called neurons, that work together to process and analyze data, enabling them to recognize patterns and make predictions.
What is the purpose of neural networks?
The main purpose of neural networks is to learn from data and extract meaningful insights or predictions. They can be used for various tasks such as classification, regression, clustering, image recognition, natural language processing, and much more. Neural networks excel at handling complex and non-linear relationships between input and output data.
How do neural networks work?
Neural networks are built using layers of interconnected nodes or neurons. Each neuron receives input data, applies a mathematical operation to it (often a weighted sum), followed by an activation function that introduces non-linearity. These calculations are performed through the network’s layers until an output is generated, representing the network’s prediction or classification.
What is the training process of neural networks?
Training a neural network involves providing labeled input data (input with known corresponding output) and adjusting the network’s parameters (weights and biases) iteratively. This is typically done using optimization algorithms like gradient descent, where the network’s predictions are compared to the true labels, and the model’s parameters are updated to minimize the prediction error.
What are the advantages of using neural networks?
Neural networks have several advantages, such as the ability to handle complex and unstructured data, automatic feature extraction, adaptability to new scenarios, and the ability to generalize from learned patterns. Additionally, they can provide state-of-the-art performance in many machine learning tasks when trained and tuned properly.
What are the limitations of neural networks?
While powerful, neural networks have some limitations. They require a large amount of labeled data for training, which can be time-consuming and expensive to acquire. Neural networks can also be computationally intensive, making them less suitable for resource-constrained devices. Additionally, they can be prone to overfitting if the training data is not representative of the real-world scenarios the model will encounter.
What is deep learning?
Deep learning is a subset of machine learning that focuses on using deep neural networks, typically with multiple hidden layers, to learn hierarchical representations of data. These deep architectures have shown remarkable success in various tasks such as image and speech recognition, natural language processing, and more, often outperforming other machine learning methods.
Are neural networks similar to the human brain?
Neural networks are inspired by the structure and functioning of the human brain but are greatly simplified compared to its complexity. Neural networks utilize artificial neurons and simplified neural connections, whereas the human brain consists of billions of interconnected neurons. Although the analogy is useful, neural networks are not biologically accurate models of the brain.
What is the role of activation functions in neural networks?
Activation functions introduce non-linearity in neural networks, allowing them to approximate complex relationships between input and output data. Common activation functions include the sigmoid, rectified linear unit (ReLU), and hyperbolic tangent (tanh). These functions determine the output of a neuron, and their choice can significantly impact the network’s learning capability and convergence speed.
Can neural networks be used for real-time applications?
Neural networks can be deployed in real-time applications, depending on the complexity of the network and available computational resources. Recent advancements in hardware acceleration and optimization techniques have made it possible to deploy deep neural networks in real-time scenarios, enabling applications such as autonomous vehicles, speech recognition systems, and more.