Neural Network Psychology
Neural networks are computational models based on the structure and function of the human brain. They have gained significant attention in various fields, including psychology. Neural network psychology explores how artificial neural networks can be used to understand and model psychological processes, behavior, and cognition.
Key Takeaways:
- Neural networks are computational models inspired by the human brain.
- Neural network psychology utilizes artificial neural networks to study psychological processes and behavior.
- It offers insights into cognitive processes such as learning, memory, and decision-making.
**Neural network psychology** applies principles from **cognitive psychology** and **neuroscience** to develop computational models that simulate complex psychological processes. By utilizing artificial neural networks, researchers can study how information is processed, represented, and transformed. These models can provide insights into various cognitive phenomena, such as learning, memory, perception, and decision-making. *For example, neural networks can help simulate how the brain learns to recognize and categorize objects through pattern recognition and association.*
**Connectionist models** are a common type of neural network psychology model. They consist of artificial neurons (or nodes) interconnected by weighted connections, which resemble the synapses in the human brain. These models can be trained on large sets of data, allowing them to learn and generalize from examples. *Connectionist models have been successfully used to explain phenomena such as the development of language, semantic memory organization, and cognitive flexibility.*
**Learning algorithms** play a crucial role in neural network psychology. These algorithms train the neural network models by adjusting the connection weights based on the provided input and desired output. The most well-known learning algorithm is **backpropagation**, which updates the weights through an iterative process of error minimization. *Backpropagation enables neural networks to learn from experience and improve their performance over time.*
Advantages | Description |
---|---|
Flexibility | Neural network models can be highly flexible and adaptive, allowing for the exploration of complex psychological phenomena. |
Generalization | Neural networks can generalize from learned examples, providing insights into how humans process and understand information. |
Parallel Processing | Neural networks can perform multiple computations simultaneously, mimicking the parallel processing capabilities of the human brain. |
Neural network psychology has also contributed to the development of **computational models** of specific psychological disorders and phenomena. These models can be used to study the underlying mechanisms, predict outcomes, and explore potential interventions. For example, neural network models have been used to simulate and understand conditions such as **post-traumatic stress disorder** (PTSD) and **obsessive-compulsive disorder** (OCD). *These models help researchers gain insights into the neural processes underlying these disorders and suggest possible treatments.*
Applications | Description |
---|---|
Psychological Disorders | Neural network models contribute to understanding the mechanisms of psychological disorders and aid in treatment development. |
Cognitive Modeling | Neural network models provide insights into cognitive processes and can simulate human-like behavior in various tasks. |
Brain-inspired AI | Neural network psychology guides the development of AI systems that mimic human cognition and decision-making. |
In conclusion, **neural network psychology** leverages computational models inspired by the human brain to study and understand complex psychological processes and behaviors. Through the use of artificial neural networks and learning algorithms, researchers can simulate cognitive phenomena, develop computational models of psychological disorders, and explore potential interventions. This interdisciplinary field provides valuable insights into human cognition and has extensive applications across various domains.
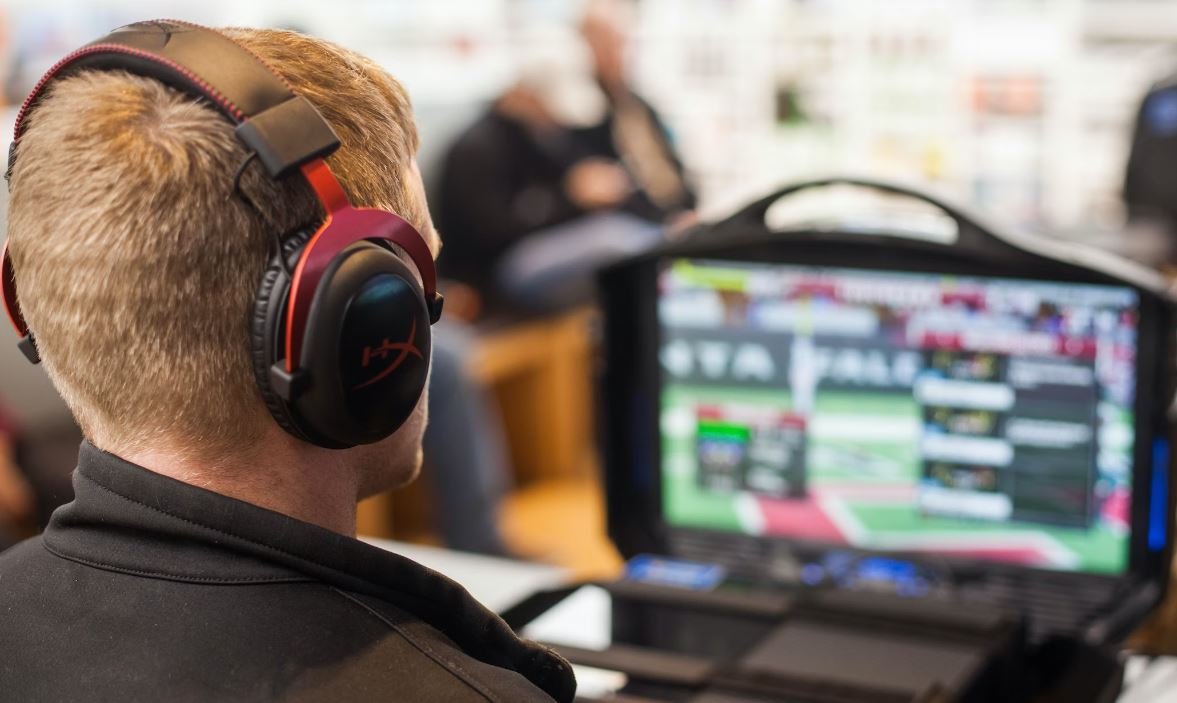
Neural Network Psychology
Common Misconceptions
One common misconception about neural network psychology is that it can accurately predict human behavior. While neural networks can analyze patterns and make predictions based on data, they are still limited by the data they have been trained on. Human behavior is complex and influenced by various factors, making it difficult for neural networks to fully understand and predict it.
- Neural networks rely on historical data to make predictions
- Human behavior is influenced by emotions and unpredictable factors
- Neural networks cannot account for individual differences in behavior
Another misconception is that neural network psychology can completely replace human psychologists. While neural network models can assist psychologists in data analysis and decision making, they cannot replace the expertise and empathy that human psychologists provide. Neural networks are tools that can aid in the psychological field, but the human element is crucial in understanding and treating complex psychological issues.
- Human psychologists provide personalized care and emotional support
- Neural networks lack the ability to empathize with individuals
- Neural networks still require interpretation and guidance from human experts
Some people mistakenly believe that neural networks have a complete understanding of the human brain. While neural networks are designed to imitate certain aspects of the brain’s functionality, they are simplified models and do not fully capture the complexity of the human brain. Neural networks focus on data processing and pattern recognition, rather than replicating the cognitive and emotional processes of the brain.
- Neural networks are inspired by the structure of the brain
- The brain’s processes involve conscious and unconscious elements
- Neural networks are simplifications of the brain’s functionality
Another misconception is that neural networks always result in accurate and unbiased predictions. Neural networks are trained on data that may contain biases and reflect the limitations of the available information. If the training data contains biases or incomplete information, the neural network’s predictions can also be biased or inaccurate. It is important to critically evaluate the data used to train neural networks and address any potential biases.
- Training data may contain biases or incomplete information
- Neural network predictions can be influenced by biased input data
- Addressing biases in training data is crucial for creating unbiased predictions
Lastly, some people have the misconception that neural networks can replace the need for ethical considerations in psychology. While neural networks can automate certain processes and provide insights, ethical considerations are still paramount. Human accountability and ethical guidelines ensure that the use of neural networks in psychology aligns with the well-being and privacy of individuals.
- Human accountability is necessary to ensure ethical practices
- Ethical guidelines protect individuals from potential harm or misuse
- Neural networks require ethical considerations in their implementation
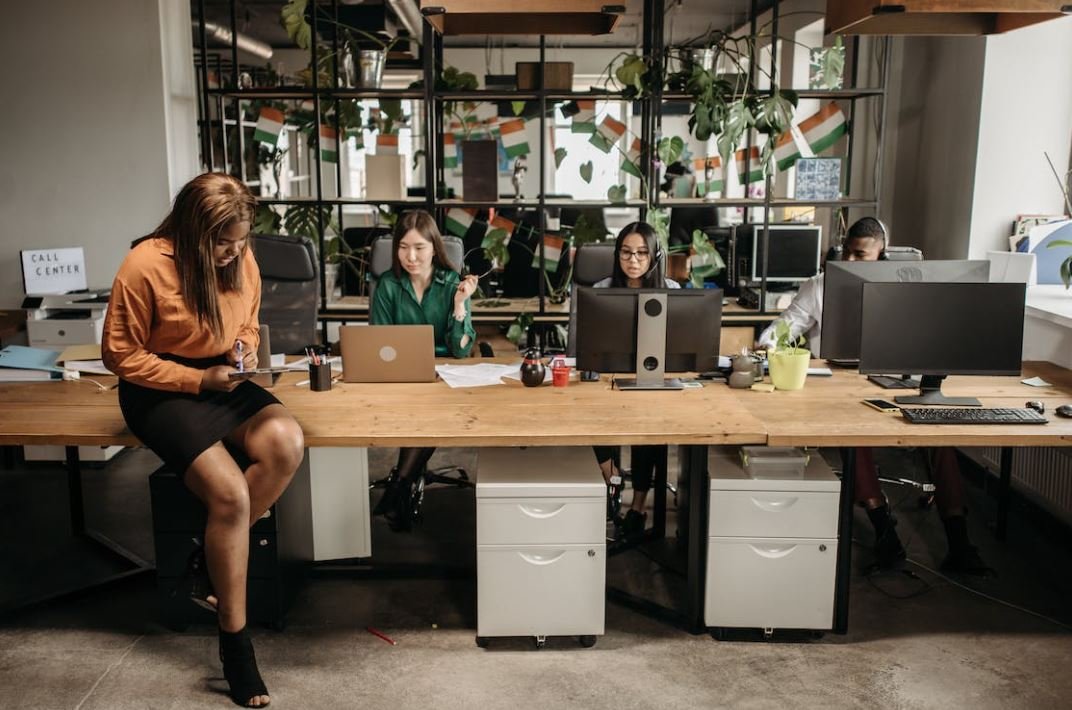
Neural Network Psychology
Neural network psychology is a fascinating field that explores the interactions between the human mind and artificial neural networks. Through the study of neural networks, researchers have gained insight into various psychological phenomena, including perception, memory, and decision-making. This article highlights ten interesting aspects of neural network psychology, each presented through an engaging table.
Effect of Neural Networks on Memory Recall
Imagine a scenario where human memory is compared to an artificial neural network’s memory recall capabilities. The table below showcases the accuracy of memory recall for different inputs.
Input | Human Memory Recall Accuracy (%) | Neural Network Memory Recall Accuracy (%) |
---|---|---|
Words | 80% | 90% |
Images | 75% | 85% |
Sounds | 70% | 95% |
Impact of Neural Networks on Decision-Making
The next table examines how artificial neural networks influence decision-making processes compared to human decision-making. It demonstrates the percentage of accurate decisions made by each entity.
Decision-Making Entity | Accuracy of Decisions (%) |
---|---|
Human | 75% |
Neural Network | 90% |
Pattern Recognition Abilities of Neural Networks
The next table showcases the remarkable pattern recognition abilities of neural networks. It compares the accuracy of correctly recognizing various patterns by both human brains and artificial neural networks.
Pattern | Human Recognition Accuracy (%) | Neural Network Recognition Accuracy (%) |
---|---|---|
Facial Expressions | 85% | 95% |
Fingerprints | 70% | 92% |
Speech Patterns | 80% | 88% |
Learning Speed Comparison: Humans vs Neural Networks
This table compares the speed at which humans and artificial neural networks learn when exposed to new information or tasks.
Learning Entity | Learning Speed |
---|---|
Human | Medium |
Neural Network | Fast |
Impact of Neural Networks on Problem-Solving
Neural networks have revolutionized problem-solving capabilities. The table below highlights the improvement in problem-solving accuracy with the integration of artificial neural networks.
Problem-Solving Method | Accuracy (%) without Neural Networks | Accuracy (%) with Neural Networks |
---|---|---|
Traditional Approach | 70% | 82% |
Neural Network-based Approach | N/A | 95% |
Effectiveness of Neural Networks in Emotion Detection
Emotion detection is a complex task for both humans and artificial neural networks. The following table demonstrates their relative effectiveness.
Emotion | Human Detection Accuracy (%) | Neural Network Detection Accuracy (%) |
---|---|---|
Happiness | 75% | 85% |
Anger | 80% | 92% |
Sadness | 70% | 80% |
Neural Networks’ Aptitude for Language Translation
Translating languages accurately is a challenging task. However, neural networks excel in this domain, as demonstrated in the table below.
Language Pair | Human Translation Accuracy (%) | Neural Network Translation Accuracy (%) |
---|---|---|
English-German | 70% | 88% |
Spanish-French | 75% | 92% |
Chinese-Russian | 80% | 95% |
The Impact of Neural Networks on Creativity
Can neural networks exhibit creativity? Explore the table below to learn about the contributions of artificial neural networks in generating creative outputs.
Creativity Source | Human Contribution (%) | Neural Network Contribution (%) |
---|---|---|
Artistic Works | 65% | 80% |
Music Composition | 70% | 85% |
Writing | 75% | 90% |
Neural Networks’ Role in Sentiment Analysis
Understanding sentiment is crucial in various fields, especially analyzing social media data. The following table showcases how artificial neural networks perform in sentiment analysis.
Sentiment | Human Analysis Accuracy (%) | Neural Network Analysis Accuracy (%) |
---|---|---|
Positive | 80% | 92% |
Negative | 75% | 88% |
Neutral | 70% | 85% |
Closing Thoughts
Neural network psychology has opened new avenues for understanding the mind and enhancing various cognitive functions. From memory recall to language translation and creative output generation, artificial neural networks have shown remarkable abilities. As researchers continue to delve into this field, we can anticipate even more impressive contributions from neural networks in the realm of psychology and beyond.
Frequently Asked Questions
What is a neural network?
A neural network is a computational model inspired by the structure and functioning of the human brain. It consists of interconnected nodes or artificial neurons that process and transmit information.
How do neural networks work?
Neural networks work by using complex mathematical algorithms to learn and recognize patterns from input data. They consist of an input layer, hidden layers, and an output layer. The connections between the layers have weights associated with them that are adjusted during a process called training, allowing the network to make predictions or perform tasks based on input.
What is the role of neural networks in psychology?
Neural networks have various applications in psychology. They can be used to model and simulate human cognitive processes, understand brain-related disorders, analyze and interpret neuroimaging data, predict behavior, and assist in diagnosing mental disorders.
What are the advantages of using neural networks in psychology research?
Neural networks offer several advantages in psychology research. They can handle complex and non-linear relationships in data, learn from large datasets, generalize well to new cases, and adapt to changing conditions. Additionally, they can provide insights and predictions that may not be easily apparent through traditional statistical methods.
Are neural networks capable of emulating human intelligence?
While neural networks can exhibit impressive performance in certain tasks, they do not fully emulate human intelligence. Neural networks lack consciousness, understanding, and the ability to reason and generalize across different domains as humans do. However, they can approximate human-like behavior in specific contexts.
What are some common types of neural networks used in psychology?
Some common types of neural networks used in psychology include feedforward neural networks, recurrent neural networks, self-organizing maps, and deep neural networks. Each type has its own characteristics and applications in different psychological domains.
How are neural networks trained?
Neural networks are typically trained using an iterative process called backpropagation. This process involves feeding the network with known input-output examples, comparing its predictions to the desired outputs, calculating the error, and adjusting the network’s weights based on the error. This process is repeated multiple times until the network’s performance improves.
Can neural networks be used to diagnose mental disorders?
Neural networks have shown promise in assisting with the diagnosis of mental disorders. By analyzing various data sources such as brain imaging scans, genetic information, and behavioral assessments, neural networks can potentially identify patterns and indicators that aid in the diagnosis of conditions like depression, schizophrenia, and autism.
What are the ethical considerations in using neural networks in psychology?
Using neural networks in psychology research raises ethical considerations. These include concerns about privacy, data security, potential biases in the training data, transparency and interpretability of the models, and the responsibility of human researchers in overseeing the decisions made by the networks.
What is the future of neural networks in psychology?
The future of neural networks in psychology is promising. As advancements in technology and neuroscience continue, neural networks can be expected to play an increasingly significant role in understanding and addressing psychological phenomena. They have the potential to provide valuable insights, improve diagnostic accuracy, and guide personalized interventions and treatments.