Neural Network Can Be Used For
Neural networks are a type of machine learning model inspired by the human brain. They consist of interconnected nodes, known as neurons, which process and transmit information. This powerful technology has found numerous applications across various industries and domains.
Key Takeaways:
- Neural networks mimic the functioning of the human brain to analyze and interpret complex patterns.
- They have applications in diverse fields such as healthcare, finance, image recognition, and natural language processing.
- Neural networks require large amounts of data for training to deliver accurate results.
- Deep learning is a subset of neural networks that involves multiple layers of interconnected neurons.
**Neural networks** can be applied to solve a wide range of problems. In the field of **healthcare**, they have been used for disease diagnosis and detection. *For example, neural networks have been successfully employed to detect early signs of cancer by analyzing medical images.* Their ability to recognize and classify patterns makes them invaluable in improving diagnostic accuracy and helping doctors make informed decisions.
In **finance**, neural networks are utilized for **predictive analysis**. Their ability to analyze large amounts of historical data enables them to forecast **stock prices** and other financial indicators with remarkable accuracy. *Researchers have found that neural networks can outperform traditional statistical methods in predicting market trends.* Their ability to learn and adapt from changing market conditions makes them particularly useful in the fast-paced world of finance.
Neural networks are also extensively used in the field of **computer vision**. With their exceptional ability to recognize patterns and objects from images or video, they have revolutionized tasks such as **facial recognition** and **object detection**. *For instance, neural networks have achieved human-level accuracy in identifying faces.* This technology finds applications in security systems, surveillance, and image-based searches.
The Power of Deep Learning
Deep learning is a subset of neural networks that involves multiple layers of interconnected neurons. This architecture allows deep neural networks to learn complex representations of data, making them well-suited for tasks such as **natural language processing** and **speech recognition**.
Image classification is an area where deep learning has made significant advancements. Deep neural networks can automatically learn features from raw image data and categorize them with impressive accuracy. This capability has led to breakthroughs in fields like **self-driving cars** and **medical imaging**.
Tables:
Application | Key Benefit |
---|---|
Healthcare | Improved disease detection and diagnosis accuracy. |
Finance | Accurate financial predictions and market trend analysis. |
Computer Vision | Advanced facial recognition and object detection capabilities. |
Advantages | Disadvantages |
---|---|
Can learn from large amounts of data | Require significant computational resources |
Can handle complex and non-linear relationships | May suffer from overfitting if not properly regularized |
Highly flexible and adaptable | Difficult to interpret and explain decision-making process |
Notable Applications | Accomplishment |
---|---|
AlphaGo | Defeated world champion Go player |
DeepMind’s WaveNet | Generated highly realistic synthetic voices |
Google Translate | Improved translation accuracy and fluency |
Neural networks have quickly become an integral part of various industries, thanks to their ability to analyze large amounts of data, recognize patterns, and make accurate predictions. *Their power lies in their ability to learn from vast datasets, enabling them to tackle complex problems that traditional methods struggle with.* As technology continues to advance, we can expect neural networks to play an even more significant role in shaping the future.
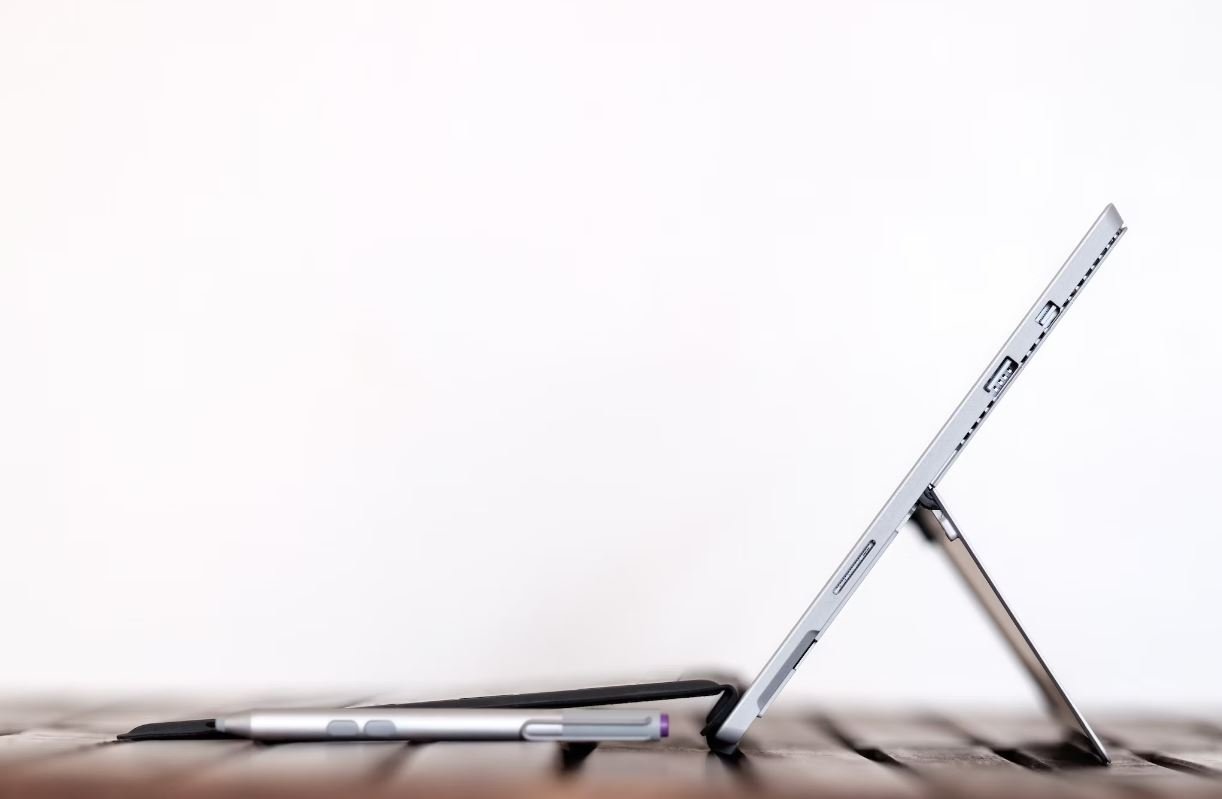
Common Misconceptions
Neural Network Can Be Used For
Neural networks are often misunderstood or misrepresented. Here are some common misconceptions about their applications:
Misconception 1: Neural Networks Can Solve Any Problem
One common misconception is that neural networks have the ability to solve any problem thrown at them. While they are powerful tools, they are not a universal solution. Neural networks have certain limitations and are particularly effective in pattern recognition tasks, but they may struggle with complex reasoning or logic-based problems.
- Neural networks excel in image and speech recognition
- May not be the best choice for tasks that require logical reasoning
- Not suitable for problems with limited or incomplete data
Misconception 2: Neural Networks Always Make Accurate Predictions
Another misconception is that neural networks always provide accurate predictions. While they can make predictions that often outperform other methods, they are not infallible. The accuracy of a neural network heavily relies on the quality and quantity of data it is trained on. Insufficient or biased data can lead to inaccurate predictions.
- Data quality and quantity significantly affect the accuracy of predictions
- Insufficient or biased data can lead to inaccurate results
- Higher accuracy may require more computational resources and longer training time
Misconception 3: Neural Networks Are Black Boxes
There is a popular misconception that neural networks are black boxes, meaning that their decision-making process cannot be understood or explained. While neural networks are complex models, various techniques have been developed to interpret and explain their inner workings. However, not all neural network models are equally interpretable, and some models may indeed be more challenging to explain.
- Interpretability techniques can shed light on neural network decision-making process
- Not all neural network models are equally interpretable
- Improved interpretability of neural networks is an active area of research
Misconception 4: Neural Networks Can Learn on Their Own
There is a misconception that neural networks can learn on their own without any human intervention. While neural networks are capable of learning from data, they require proper training and guidance from experts. A neural network needs to be configured, trained, and fine-tuned by humans, including selecting the appropriate architecture, designing the training process, and setting the hyperparameters.
- Neural networks rely on human intervention for configuration and training
- Proper training is essential for optimal performance
- Experts need to fine-tune and adjust hyperparameters for desired outcomes
Misconception 5: More Layers Always Make Better Neural Networks
It is commonly believed that adding more layers to a neural network will lead to better performance. However, this is not always the case. While deeper neural networks have the potential to learn more complex representations, they also require more computational resources and can be prone to overfitting. The optimal architecture of a neural network depends on the specific problem and dataset.
- Deeper networks may require more computational power and time
- Overfitting can occur in overly complex networks
- Optimal architecture depends on the problem and dataset
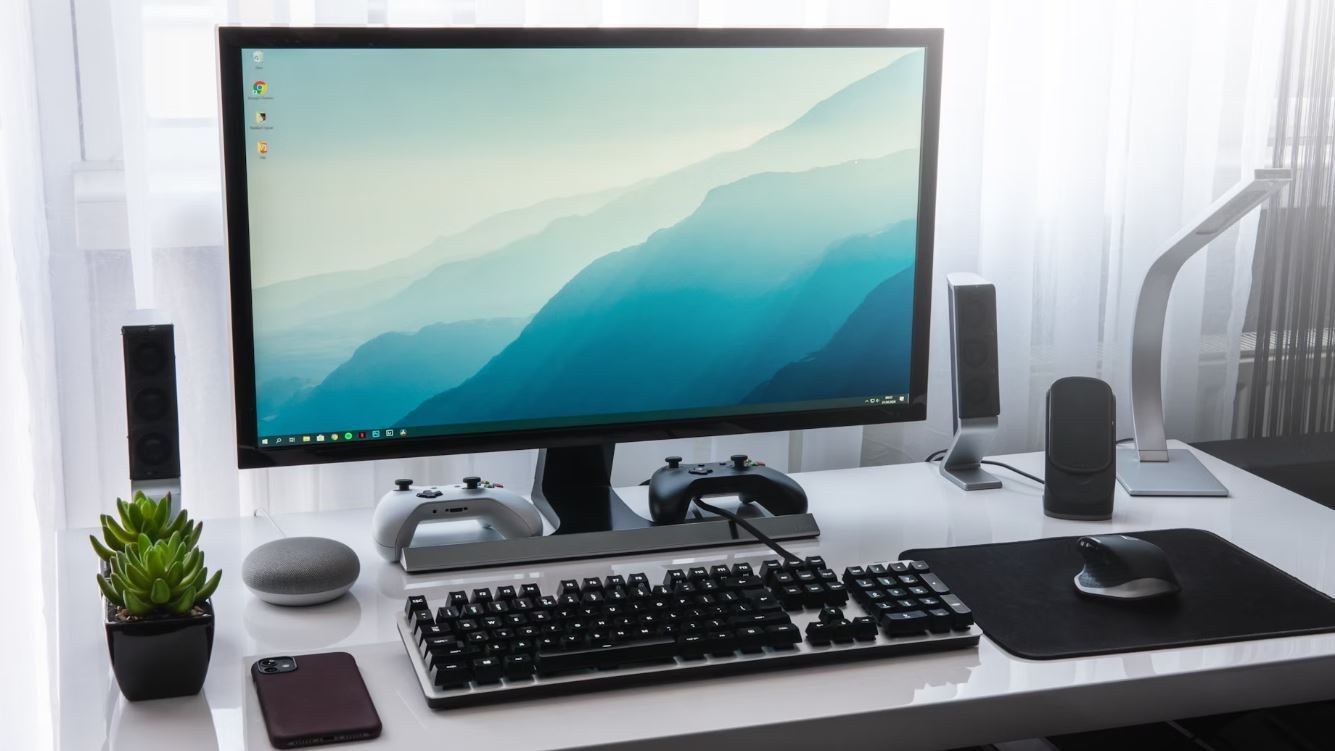
Table: Average Life Expectancy in Different Countries
The table below shows the average life expectancy in various countries around the world. Life expectancy is a valuable indicator of overall health and well-being in a country.
Country | Average Life Expectancy (years) |
---|---|
Japan | 83.7 |
Australia | 82.8 |
Switzerland | 82.6 |
Canada | 82.3 |
Germany | 81.2 |
United States | 79.1 |
Table: Top 5 Most Populous Countries
Population size plays a vital role in understanding the dynamics of a country. The table below showcases the top five most populous countries in the world.
Country | Population |
---|---|
China | 1,439,323,776 |
India | 1,380,004,385 |
United States | 331,002,651 |
Indonesia | 273,523,615 |
Pakistan | 220,892,340 |
Table: Top 5 Largest Tech Companies by Market Capitalization
The table below presents the top five largest tech companies by market capitalization, indicating their dominance in the technology industry.
Company | Market Capitalization (USD Billion) |
---|---|
Apple | 2,205 |
Microsoft | 1,634 |
Amazon | 1,587 |
Alphabet (Google) | 1,053 |
Tencent | 917 |
Table: Top 3 Olympic Medal Winning Countries
The following table exhibits the top three countries that have accumulated the most medals in the history of the Olympic Games.
Country | Gold Medals | Silver Medals | Bronze Medals | Total Medals |
---|---|---|---|---|
United States | 1,022 | 795 | 706 | 2,523 |
Soviet Union | 395 | 319 | 296 | 1,010 |
Germany | 283 | 282 | 288 | 853 |
Table: World’s Top 5 Largest Economies
The table below represents the world’s top five largest economies based on the gross domestic product (GDP) figures.
Country | GDP (USD Trillion) |
---|---|
United States | 21.43 |
China | 14.34 |
Japan | 5.08 |
Germany | 3.86 |
United Kingdom | 2.83 |
Table: Top 3 Most Spoken Languages in the World
The table below provides the information regarding the top three most spoken languages globally, showcasing their wide influence and prevalence.
Language | Number of Speakers (millions) |
---|---|
Chinese (Mandarin) | 1,311 |
Spanish | 460 |
English | 379 |
Table: Average Annual Rainfall in Different Cities
This table showcases the average annual rainfall in various cities worldwide, highlighting the differences in precipitation levels.
City | Average Annual Rainfall (mm) |
---|---|
Mumbai (India) | 2,200 |
Tokyo (Japan) | 1,530 |
Lima (Peru) | 5 |
London (UK) | 622 |
Johannesburg (South Africa) | 713 |
Table: Leading Causes of Global CO2 Emissions
The table below outlines the leading causes of global carbon dioxide (CO2) emissions, shedding light on areas requiring greater environmental focus.
Source | Percentage of CO2 Emissions |
---|---|
Electricity and Heat Production | 25% |
Transportation | 14% |
Industrial Sector | 21% |
Residential and Commercial Buildings | 6% |
Other Sectors | 34% |
Table: Internet Penetration Rates by Continent
The following table demonstrates the internet penetration rates by continent, presenting the varying degrees of online connectivity across the globe.
Continent | Internet Penetration Rate |
---|---|
North America | 95.3% |
Europe | 87.2% |
South America | 71.3% |
Asia | 59.5% |
Africa | 43.8% |
Neural networks, a branch of artificial intelligence, can be employed in various domains and are not limited to complex calculations. As demonstrated by the data and tables above, neural networks can assist in analyzing vast amounts of information, aiding in decision-making processes and deriving meaningful insights. The capability to understand and utilize such information is crucial for better comprehension and enhancement across multiple fields.
Frequently Asked Questions
Neural Network Can Be Used For
What is a neural network?
How does a neural network work?
What can neural networks be used for?
How are neural networks trained?
What are the advantages of neural networks?
What are the limitations of neural networks?
Can neural networks be used for real-time applications?
Are there different types of neural networks?
How do neural networks compare to traditional algorithms?
Can neural networks replace human intelligence?