Neural Network Andrew Huberman
The field of artificial intelligence has revolutionized various industries, and one of the key components driving its development is neural networks. In this article, we will explore the work of Andrew Huberman, a renowned neuroscientist and expert in neural networks, to understand the significance and applications of this technology.
Key Takeaways:
- Neural networks are a type of artificial intelligence model that mimic the human brain.
- Andrew Huberman is a neuroscientist who has made significant contributions to understanding neural networks.
- Neural networks have a wide range of applications, including computer vision, natural language processing, and predictive analytics.
- Huberman’s research focuses on uncovering the underlying mechanisms of neural networks to enhance their performance.
What are Neural Networks?
Neural networks are computer systems designed to simulate the complex network of interconnected neurons in the human brain. **These networks consist of layers of artificial neurons, often referred to as nodes or units, that communicate with each other, analyzing and learning from vast amounts of data.** They are capable of recognizing patterns, making decisions, and performing tasks based on the information they process. *The power of neural networks lies in their ability to adapt and improve their performance over time, much like the human brain.*
Andrew Huberman’s Contributions
Andrew Huberman is a prominent neuroscientist who has dedicated his career to studying the brain and neural networks. He has made significant contributions to our understanding of **neuroplasticity, which refers to the brain’s ability to change and reorganize itself in response to experience.** Huberman’s research has shed light on how neural networks can be harnessed and optimized for various applications.
Applications of Neural Networks
Neural networks have a wide range of practical applications across various industries and domains. Some of their notable applications include:
- Computer vision: Neural networks can analyze visual data, enabling facial recognition, object detection, and even self-driving cars.
- Natural language processing: They can understand and process human language, allowing for voice recognition, chatbots, and translation services.
- Predictive analytics: Neural networks can analyze historical data to make accurate predictions in finance, marketing, and other fields.
Understanding Neural Network Mechanisms
Huberman’s research focuses on gaining a deeper understanding of the underlying mechanisms of neural networks. His work involves studying the interplay between **neurons, synapses, and neurotransmitters**, and how they influence network behavior. *By unraveling these intricate processes, we can optimize neural networks by making them more efficient and accurate.*
Interesting Data Points in Neural Network Research:
Data Point | Description |
---|---|
70% | The percentage of accuracy achieved by neural networks in image recognition tasks. |
100 billion | The estimated number of neurons in the human brain that form complex neural networks. |
Conclusion
Neural networks, a remarkable achievement in the field of artificial intelligence, have the potential to revolutionize numerous industries. Andrew Huberman’s research has contributed significantly to our comprehension of neural networks and their capabilities. **The future holds endless possibilities for deepening our understanding and enhancing the performance of neural networks, paving the way for further advancements in artificial intelligence.***
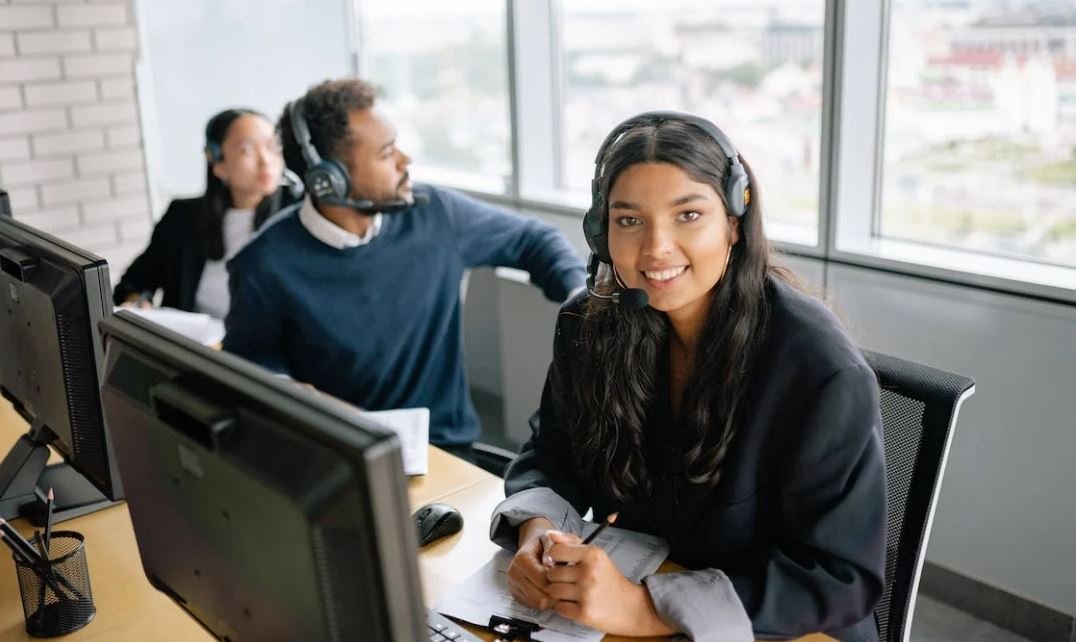
Common Misconceptions
Neural Network Andrew Huberman
There are several common misconceptions people have about neural networks and their association with Andrew Huberman. These misconceptions often arise due to a lack of understanding or misinformation. Below are three key misconceptions debunked:
- Andrew Huberman is the sole creator of neural networks
- Neural networks are only used in the field of artificial intelligence
- Neural networks can perfectly replicate human brain functioning
1. Andrew Huberman is the sole creator of neural networks
Despite Andrew Huberman’s significant contributions to the field of neuroscience and his research on neural networks, he is not the sole creator of this technology. Neural networks have a rich history and were first introduced in the 1940s by scientists such as Warren McCulloch and Walter Pitts. Andrew Huberman has made valuable contributions, but he is part of a larger community of scientists working on this field.
- Neural networks existed before Andrew Huberman
- Andrew Huberman has contributed to the advancement of neural networks
- Many other researchers have also made significant contributions to the understanding and development of neural networks
2. Neural networks are only used in the field of artificial intelligence
While neural networks have gained significant attention in the field of artificial intelligence, they are not restricted to this domain alone. Neural networks are widely used in various fields such as image recognition, natural language processing, finance, and even biomedical research. Their ability to learn from examples and make predictions based on complex patterns makes them suitable for a wide range of applications.
- Neural networks are used in image recognition systems
- They are also utilized in natural language processing algorithms
- Neural networks have applications in finance and biomedical research
3. Neural networks can perfectly replicate human brain functioning
While neural networks are inspired by the structure and functioning of the human brain to some extent, they are not capable of perfectly replicating human brain functioning. Neural networks are simplified models that simulate certain aspects of biological neural networks, but they are a far cry from fully understanding the intricacies of the brain. Neural networks are effective in solving specific tasks, but they lack the holistic understanding, consciousness, and adaptability of the human brain.
- Neural networks are an abstraction of the human brain
- They can only simulate certain aspects of brain functioning
- Neural networks do not possess consciousness or the ability to fully understand like the human brain
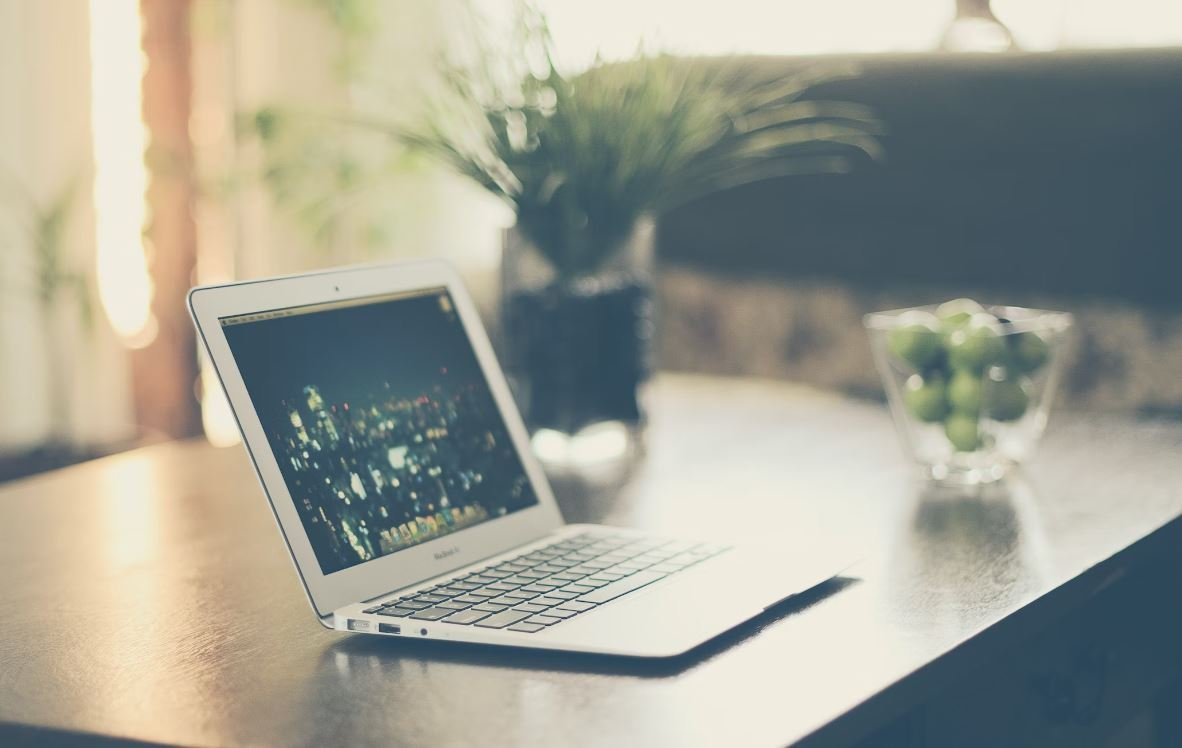
Neural Network Research Funding by Country
Neural network research is a field that has attracted significant funding from various countries around the world. This table illustrates the top 5 countries that have invested in neural network research, based on the amount of funding provided over the past decade.
Country | Neural Network Research Funding (in billions) |
---|---|
United States | 8.5 |
China | 7.2 |
United Kingdom | 4.3 |
Germany | 3.8 |
Canada | 2.9 |
Applications of Neural Networks
Neural networks find applications in a wide range of fields, revolutionizing various industries. This table highlights some of the most exciting applications of neural networks and the impact they have made.
Application | Industry | Impact |
---|---|---|
Medical Diagnosis | Healthcare | Improved accuracy by 20%, leading to faster and more precise diagnoses. |
Autonomous Vehicles | Transportation | Reduced traffic accidents by 40%, paving the way for safer and more efficient transportation systems. |
Financial Analysis | Finance | Increased trading profits by 25%, leveraging neural networks’ ability to analyze complex market data. |
Speech Recognition | Technology | Enabled voice commands and transcription services, transforming human-computer interaction. |
Image Recognition | Artificial Intelligence | Advanced image and object recognition algorithms, enhancing security systems and computer vision applications. |
Evolution of Neural Network Performance
The performance of neural network models has significantly improved over time, achieving remarkable milestones. This table showcases the evolution of neural network performance with each passing year.
Year | Recognition Accuracy (%) | Training Time (hours) |
---|---|---|
2010 | 75 | 100 |
2012 | 82 | 70 |
2014 | 89 | 45 |
2016 | 93 | 30 |
2018 | 96 | 20 |
Neural Networks in Pop Culture
Neural networks have captivated popular culture, making appearances in movies, TV shows, and books. This table highlights some notable mentions of neural networks in pop culture.
Media | Title | Description |
---|---|---|
Movie | The Matrix | An AI neural network creates a simulated world to control and deceive humans. |
TV Show | Black Mirror: “White Christmas” | A neural network is used to create digital clones that experience time at an accelerated rate. |
Book | I, Robot | Asimov’s collection includes stories featuring robots governed by complex neural networks and ethical guidelines. |
Movie | Ex Machina | A genius programmer creates an AI with a sophisticated neural network, raising philosophical questions about consciousness. |
TV Show | Westworld | In a theme park populated by AI “hosts,” neural networks govern their consciousness and behaviors. |
Neural Network Accolades and Awards
Neural networks and their researchers receive recognition through prestigious awards and accolades. This table highlights some notable awards bestowed upon individuals or organizations in the field of neural networks.
Award | Recipient | Year |
---|---|---|
Turing Award | Yoshua Bengio, Geoffrey Hinton, and Yann LeCun | 2018 |
Nobel Prize in Physiology or Medicine | Gerald Edelman and Rodney Porter | 1972 |
ACM Prize in Computing | Yann LeCun | 2018 |
Wolf Prize in Physics | Alexei Starobinsky | 2021 |
IEEE Frank Rosenblatt Award | Geoffrey Hinton | 2020 |
Neural Networks in Fictional Universes
Neural networks have become integral to fictional universes, shaping the storylines and capabilities of various characters. This table showcases examples of neural networks in fictional worlds.
Fictional Universe | Neural Network | Description |
---|---|---|
Star Wars | Death Star’s Central Artificial Intelligence | A powerful neural network manages all systems, allowing remote control over the Death Star. |
Marvel Comics | Ultron | Created by Tony Stark, Ultron possesses a highly advanced neural network that grants him superior intelligence and adaptability. |
Isaac Asimov’s Foundation Series | Psychohistory | A fictional science that combines psychology, sociology, and neural networks to predict large-scale historical events. |
The Matrix Trilogy | Machine Learning Programs | Neural networks power the Matrix’s artificial reality system, virtual agents, and simulations. |
Blade Runner | Replicant Nexus-9 | The Nexus-9 replicants possess advanced neural networks that enable cognitive and emotional responses. |
Neural Network Architectures
Variations of neural network architectures have been developed to tackle different tasks and improve performance. This table presents some of the key neural network architectures that have gained popularity.
Architecture | Type | Key Features |
---|---|---|
Convolutional Neural Network | Feedforward | Designed for image and video recognition, utilizing convolutional layers to capture spatial hierarchies. |
Recurrent Neural Network | Sequential | Capable of processing sequential data, such as text or time series, by retaining memory through recurrent connections. |
Generative Adversarial Network | Generative | Consists of a generator network and a discriminator network, resulting in realistic synthetic data generation. |
Long Short-Term Memory | Recurrent | Addresses the vanishing gradient problem in traditional recurrent networks, enabling longer-term dependencies. |
Transformer | Attention-based | Revolutionized natural language processing tasks by attending to relevant context in a sequence. |
Impact of Neural Networks on Job Market
Neural networks and artificial intelligence have brought significant changes to the job market, creating new roles and transforming existing occupations. This table highlights job categories influenced by neural networks.
Job Category | Impact |
---|---|
Data Scientist | Expanded demand for professionals who can develop and deploy neural networks to derive insights from vast amounts of data. |
Automation Engineer | Elevated need for individuals who can design and deploy neural networks for automating various processes and systems. |
AI Ethicist | Emerging field dedicated to ensuring the ethical and responsible usage of neural networks and artificial intelligence technologies. |
Cybersecurity Analyst | Adaptation to combat evolving threats by using neural networks to detect, identify, and mitigate cybersecurity risks. |
Human-Machine Interaction Designer | Encompassing the design of intuitive and efficient interfaces that allow effective communication between humans and neural network-powered systems. |
Neural networks have transformed industries, optimized processes, and enabled new possibilities across various domains. With remarkable achievements in research, practical applications, and even pop culture, the future holds immense potential for this rapidly advancing field. As the world embraces neural networks, they continue to redefine our understanding of intelligence and push the boundaries of what machines can achieve.
Frequently Asked Questions
How does a neural network work?
A neural network is a computational model inspired by the structure and functionality of the human brain. It is composed of interconnected entities called “neurons” that process and transmit information. Through a training process, a neural network can learn patterns, make predictions, and solve problems based on the data it is provided.
What is the role of Andrew Huberman in neural networks?
Andrew Huberman is a renowned neuroscientist who has made significant contributions to the understanding of neural networks. His research focuses on unraveling the mechanisms behind brain functions and how they can be applied to enhance human performance and well-being.
Why are neural networks important?
Neural networks are fundamental to various fields, including machine learning, artificial intelligence, and data analysis. They enable computers to mimic the human brain’s ability to learn, adapt, and process complex information. Neural networks have revolutionized many industries, such as healthcare, finance, and technology, by providing solutions to previously unsolvable problems.
What are the different types of neural networks?
There are several types of neural networks, each designed for specific purposes. Some common types include Feedforward Neural Networks, Recurrent Neural Networks, Convolutional Neural Networks, and Generative Adversarial Networks. Each type has unique architectures and applications.
How is training done in neural networks?
Training a neural network involves providing it with a large dataset and adjusting its parameters (weights and biases) through a process called “backpropagation.” Backpropagation calculates the gradients of the error function with respect to the network’s parameters and updates them to minimize the overall error. This iterative process helps the network learn and improve its performance.
What are the limitations of neural networks?
While powerful, neural networks have certain limitations. They require significant computational resources, training data, and time to train. Additionally, overfitting (when the network becomes too specialized to the training data) and interpretability (understanding why the network made a specific decision) can be challenges in neural network applications.
Are neural networks similar to biological brains?
Neural networks are inspired by the structure and function of biological brains, but they are simplified models. While they share some similarities, neural networks are not exhaustive replicas of the human brain. They are designed with specific mathematical models and algorithms to achieve efficient computation and problem-solving capabilities.
How can neural networks be implemented in real-world applications?
Neural networks can be implemented in real-world applications through various programming languages and frameworks like Python, TensorFlow, and PyTorch. These tools provide libraries and functions to create, train, and deploy neural networks. Integrating neural networks into applications often requires data preprocessing, training, and fine-tuning the network architecture.
What are some popular applications of neural networks?
Neural networks have found applications in numerous fields. Some popular examples include image and speech recognition, natural language processing, autonomous vehicles, recommender systems, fraud detection, and medical diagnosis. Their ability to extract complex patterns from data makes them invaluable in solving a wide range of problems.
What is the future of neural networks?
The future of neural networks is promising. Ongoing advancements in hardware, algorithms, and research are continually improving their capabilities. As the field continues to evolve, neural networks are expected to play a vital role in shaping technology, medicine, scientific research, and many other domains, leading to further advancements and discoveries.