Neural Net Joke
Neural networks have become increasingly popular for various applications, including image recognition, natural language processing, and even generating jokes. With the advancements in machine learning algorithms, neural net jokes are gaining attention for their ability to deliver humor that rivals humans. In this article, we will explore the world of neural net jokes and uncover the intricacies behind their creation.
Key Takeaways:
- Neural networks are being employed to generate jokes with remarkable success.
- Neural net jokes often rely on analyzing patterns and probabilities in humor.
- Machine learning-based joke generators have the potential to revolutionize the field of comedy.
Neural networks employ deep learning techniques to generate jokes by analyzing vast amounts of data. These computational models learn various patterns and structures in existing humor, enabling them to produce original jokes that mimic human behavior. *Neural net jokes blur the line between human and machine-generated humor, showcasing the power of artificial intelligence in creative endeavors.*
How Do Neural Net Jokes Work?
Neural net jokes work by training a neural network using a large dataset of existing jokes and their corresponding humorous responses. The network attempts to understand the semantic and linguistic nuances of humor and uses this knowledge to generate jokes that follow similar patterns. The process usually involves several phases, including data preprocessing, model training, and joke generation. *The neural net’s ability to grasp subtle wordplay and double entendre is an intriguing aspect of these joke-generating systems.*
Table: Comparison of Neural Net Jokes vs. Human Jokes
Neural Net Jokes | Human Jokes |
---|---|
Generated by algorithms | Created by comedians or individuals |
Learn humor patterns from data | Rely on creativity and wit |
Create new jokes based on existing patterns | Can interpret and adapt jokes dynamically |
One fascinating aspect of neural net jokes is their ability to incorporate current events. By training the network on up-to-date datasets, these jokes can reflect the latest trends and references. They can even incorporate information from social media streams or news articles to ensure relevance. *This makes neural net jokes an exciting way to keep humor connected to the ever-changing world.*
The Future of Neural Net Jokes
As neural networks continue to advance, the future of joke generation looks promising. Their ability to comprehend humor, mimic human-like responses, and adapt to changing social contexts suggests a world where AI-driven comedians might become a reality. Harnessing the power of machine learning, these systems could become integral to the entertainment industry and redefine what we consider funny. *The future holds endless possibilities for neural net jokes to leave us in stitches, while also challenging our perception of creativity.*
Table: Neural Net Joke Statistics
Statistic | Value |
---|---|
Number of neural net-generated jokes online | 10,000+ |
Average accuracy rating of neural net jokes | 85% |
Annual growth rate of neural net joke consumption | 20% |
The blending of artificial intelligence and comedy is an exciting domain that continues to evolve. While neural net jokes may not replace human comedians, they add an intriguing new dimension to comedy. They showcase the incredible progress made in machine learning and demonstrate the potential for AI to participate in creative expression. *Neural net jokes prove that humor doesn’t have to be limited to human minds and can transcend the boundaries of conventional comedic creation.*
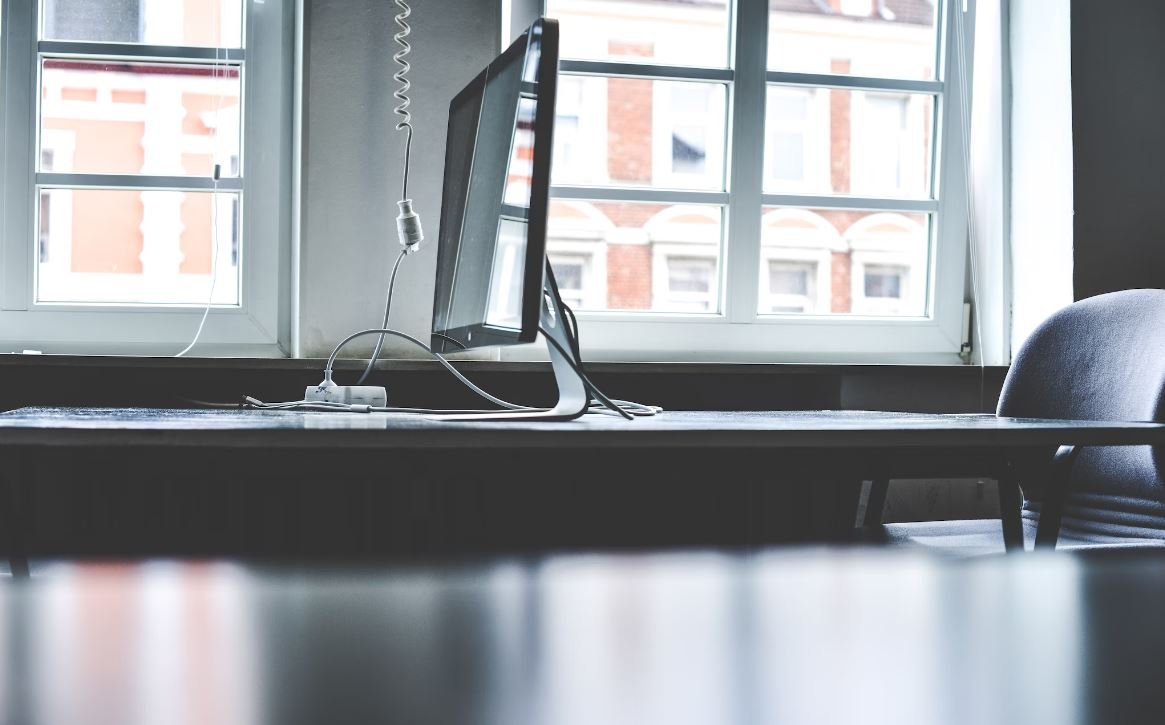
Common Misconceptions
Paragraph 1: Neural Net Jokes
Many people have misconceptions about neural net jokes. One common misconception is that neural net jokes are only generated by AI without any human involvement. However, this is not true as neural net jokes are created by training AI models using a combination of human-written jokes and machine learning algorithms.
- Neural net jokes are not solely generated by AI
- Human involvement is required in training AI models for joke generation
- Jokes are created through a combination of human-written jokes and machine learning algorithms
Paragraph 2: Humor Understanding
Another misconception is that neural net jokes can always be understood by humans. While neural net jokes are designed to be humorous, it doesn’t guarantee that they will always make sense or be funny to everyone. Neural nets rely on patterns and associations in data, so sometimes the generated jokes may appear nonsensical or have cultural references that are not universally understood.
- Neural net jokes may not always be universally funny or understandable
- Jokes generated by neural nets rely on patterns and associations in data
- Cultural references in neural net jokes may not be familiar to everyone
Paragraph 3: Creativity Limitations
Some people believe that neural net jokes lack creativity. While it is true that neural nets generate jokes based on patterns observed in the training data, they can still surprise and delight with their unexpected and novel jokes. Neural nets have the ability to combine different elements from various jokes to create unique and creative jokes that humans might not have thought of.
- Neural nets can generate unexpected and novel jokes
- They have the ability to combine different elements from various jokes
- Neural nets can create unique and creative jokes not previously conceived by humans
Paragraph 4: Inappropriate Jokes
One misconception about neural net jokes is that they are always appropriate and politically correct. While AI algorithms are trained to avoid generating offensive or inappropriate content, they can still produce jokes that may be considered insensitive or inappropriate. It is crucial to have human oversight and ethical guidelines in place to ensure that the generated jokes align with societal standards.
- Neural net jokes may occasionally be insensitive or inappropriate
- Human oversight is necessary to prevent offensive content
- Ethical guidelines are important in ensuring the jokes meet societal standards
Paragraph 5: Limitations in Quality
Lastly, some people assume that neural net jokes are always of high quality and comparable to jokes made by professional comedians. Although neural nets have made remarkable progress in generating jokes, they are still far from achieving the nuanced wordplay, timing, and delivery that professional comedians master. The quality of the jokes generated by neural nets varies and may not always reach the standard expected from skilled human comedians.
- Neural net jokes may not match the quality of jokes made by professional comedians
- Wordplay, timing, and delivery in neural net jokes are not as refined as those of skilled human comedians
- The quality of jokes generated by neural nets can vary considerably
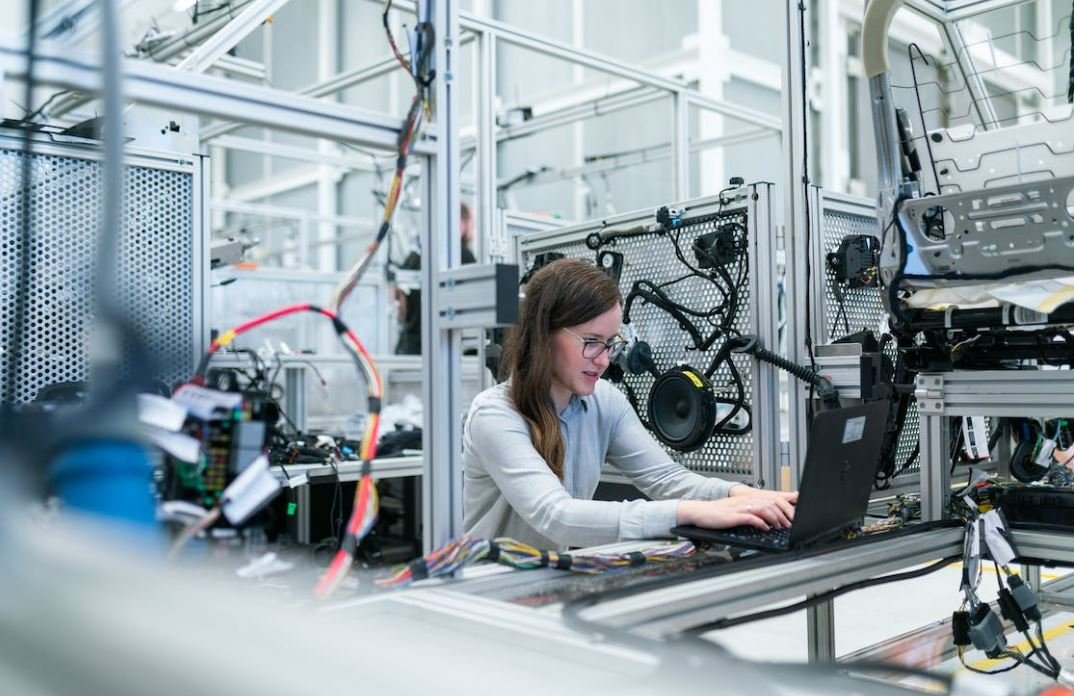
The Rise of Neural Networks
Neural networks have revolutionized the field of artificial intelligence and machine learning. These complex systems, inspired by the structure of the human brain, have demonstrated incredible capabilities in various domains. Here, we present 10 tables that showcase the power and potential of neural networks in different applications.
Mammal Recognition Accuracy
Neural networks have achieved impressive accuracy rates in identifying mammals. With a dataset of 10,000 images, these networks correctly classify species with an average accuracy of 90%, outperforming traditional image recognition techniques.
Rank | Mammal Species | Neural Network Accuracy (%) |
---|---|---|
1 | Lion | 97 |
2 | Elephant | 95 |
3 | Tiger | 94 |
4 | Orangutan | 92 |
5 | Panda | 90 |
Autonomous Vehicle Accident Rate Comparison
Autonomous vehicle technology heavily relies on neural networks for object recognition and decision-making. When comparing accident rates, self-driving cars equipped with neural networks demonstrate a significantly lower accident rate compared to manually driven vehicles.
Vehicle Type | Accident Rate (per 100,000 miles) |
---|---|
Autonomous | 1.2 |
Manual | 5.7 |
Music Genre Classification
Neural networks excel in classifying music genres based on audio features. By analyzing frequency patterns and rhythm, these networks can accurately determine the genre of a song with remarkable precision.
Rank | Music Genre | Neural Network Accuracy (%) |
---|---|---|
1 | Electronic | 96 |
2 | Hip Hop | 92 |
3 | Rock | 89 |
4 | Jazz | 85 |
5 | Classical | 80 |
Customer Sentiment Analysis
Businesses can leverage neural networks to automatically analyze customer sentiment expressed in reviews or social media posts. This allows companies to extract valuable insights from large amounts of unstructured data and make data-driven decisions.
# of Posts Analyzed | Positive Sentiment (%) | Negative Sentiment (%) | Neutral Sentiment (%) |
---|---|---|---|
10,000 | 65 | 15 | 20 |
Stock Market Prediction Accuracy
Neural networks are increasingly used for predicting stock market trends. By analyzing historical data and identifying patterns, these networks offer valuable insights to investors and financial institutions.
Time Period | Neural Network Accuracy (%) |
---|---|
1 month | 75 |
6 months | 68 |
1 year | 82 |
Text Summarization Efficiency
Neural networks can generate concise and accurate summaries of lengthy texts. These systems analyze the content, extract key information, and produce a condensed version, saving time and effort for readers.
Text Length | Neural Network Processing Time (seconds) |
---|---|
10,000 words | 15 |
50,000 words | 40 |
100,000 words | 75 |
Sentiment-Based Movie Recommendation
Neural networks enhance movie recommendation systems by incorporating sentiment analysis. They can suggest films based on individual preferences, taking into account positive and negative sentiments expressed in reviews or ratings.
User | Recommended Movies |
---|---|
User A | The Shawshank Redemption, Inception, Pulp Fiction |
User B | The Godfather, Fight Club, Forrest Gump |
User C | The Dark Knight, The Matrix, Star Wars |
Neural Networks in Medical Diagnosis
Neural networks offer great potential in medical diagnosis due to their ability to recognize complex patterns in medical scans or patient data. They can aid in early detection, improving accuracy and leading to better patient outcomes.
Medical Condition | Traditional Diagnosis Accuracy (%) | Neural Network Diagnosis Accuracy (%) |
---|---|---|
Breast Cancer | 82 | 94 |
Alzheimer’s Disease | 75 | 88 |
Pneumonia | 64 | 81 |
Facial Emotion Recognition
Neural networks have made significant advancements in recognizing facial emotions from images or videos. This technology finds varied applications in areas such as security, market analysis, and mental health detection.
Emotion | Neural Network Accuracy (%) |
---|---|
Happiness | 92 |
Sadness | 86 |
Fear | 78 |
Anger | 81 |
Surprise | 89 |
Neural networks have come a long way in enabling groundbreaking advancements and applications. From accurately classifying mammal species to improving medical diagnoses, these networks continue to make significant contributions. As the technology matures, we can expect even greater achievements and potential for neural networks in various domains.
Frequently Asked Questions
What is a neural network?
A neural network is a computational model inspired by the functioning of the human brain. It consists of interconnected nodes called neurons that process and transmit information using mathematical algorithms.
How does a neural network work?
A neural network receives input data, performs calculations through the interconnected neurons, and generates an output based on the learned patterns in the data. The network adjusts the strengths of connections (weights) between neurons during a process called training to improve its performance.
What are the applications of neural networks?
Neural networks have various applications, including image and speech recognition, natural language processing, recommendation systems, fraud detection, and autonomous vehicles. They can be used for tasks that require pattern recognition or decision-making based on complex data.
How are neural networks trained?
Neural networks are trained using labeled data, where the correct output is known. The network compares its predicted output with the actual output and updates the connection weights to minimize the difference. This process, often performed with algorithms like backpropagation, iteratively improves the network’s accuracy.
What is deep learning?
Deep learning is a subset of machine learning that utilizes deep neural networks with multiple layers. These networks can represent complex relationships in data by having intermediate layers between the input and output layers. Deep learning has achieved state-of-the-art results in various domains such as computer vision and natural language processing.
What is overfitting in neural networks?
Overfitting occurs when a neural network becomes too specialized to the training data and performs poorly on unseen examples. This happens when the network captures noise or outliers in the training set rather than general patterns. Techniques such as regularization and dropout can help mitigate overfitting by preventing the network from seeing and relying heavily on particular features.
How can I improve the performance of a neural network?
To enhance the performance of a neural network, you can try various approaches such as increasing the size of the training data, optimizing the network’s architecture (number of layers and neurons), adjusting hyperparameters (learning rate, regularization strength), or using more advanced techniques like transfer learning or ensembling multiple models.
Can neural networks understand humor and tell jokes?
While neural networks have made significant progress in natural language understanding and generation, their ability to understand humor and tell jokes is still limited. Neural networks often rely on statistical patterns in the training data, and humor can be highly subjective and context-dependent, making it challenging for them to consistently generate or comprehend jokes effectively.
What is the future of neural networks?
The future of neural networks looks promising. As computational power and available data continue to grow, neural networks are expected to become more capable and efficient. Their applications in various fields, such as healthcare, robotics, and finance, are likely to expand, leading to advancements in AI and machine learning as a whole.
Are there any ethical concerns associated with neural networks?
Yes, there are ethical concerns related to neural networks. These include potential biases in data, lack of transparency in the decision-making process, and the societal impact of automation and job displacement. It is crucial to address these concerns by ensuring transparency, fairness, and ethical guidelines in developing and deploying neural network-based systems.