Neural Net As
Neural Net As is a powerful technology that is revolutionizing various industries, including finance, healthcare, and marketing. Neural nets are a type of artificial neural network (ANN) inspired by the structure and functions of the brain. They are capable of learning and adapting, making them incredibly versatile tools for data analysis and decision-making.
Key Takeaways
- Neural nets are artificial neural networks inspired by the structure and functions of the brain.
- These advanced algorithms are capable of learning and adapting, making them versatile tools for data analysis and decision-making.
- The applications of neural nets span across various industries, with significant impacts on finance, healthcare, and marketing.
Neural nets, also known as deep learning models, consist of multiple layers of interconnected nodes. Each node is called a neuron and is responsible for processing and transmitting information. By employing these interconnected layers, neural nets can simulate the complex behaviors of the human brain, enabling them to recognize patterns and make accurate predictions.
**Neural nets are highly effective in image and speech recognition tasks**, surpassing traditional algorithms in accuracy and performance. This capability has been harnessed in areas like self-driving cars and voice assistants, improving the way these technologies interact with and understand their environment.
Applications of Neural Net As
Neural nets have found diverse applications in numerous industries, providing innovative solutions to complex problems:
- Finance: Neural nets are extensively used in stock market prediction and algorithmic trading. These models can analyze large volumes of financial data in real-time to identify trends and make profitable trading decisions.
- Healthcare: Neural nets play a crucial role in medical image analysis, facilitating early detection and diagnosis of diseases such as cancer. They can interpret complex medical images with high accuracy, assisting healthcare professionals in delivering precise and timely treatment.
- Marketing: Neural nets enable targeted advertising campaigns by analyzing vast amounts of customer data. They can segment audiences, predict consumer behavior, and personalize marketing messages, leading to more effective and efficient advertising strategies.
**Neural nets have demonstrated exceptional performance in a wide range of tasks**, showcasing their potential to revolutionize industries and improve decision-making processes.
Benefits of Neural Net As
Implementing neural net As offers several advantages for businesses and organizations:
- Ability to analyze vast amounts of complex data and extract meaningful insights.
- Improved accuracy and prediction capabilities, surpassing traditional algorithms in various domains.
- Flexibility and adaptability, allowing neural nets to continually learn and improve performance over time.
- Automation of repetitive tasks, increasing efficiency and reducing human error.
**Neural nets have the potential to revolutionize industries and drive innovation**, empowering organizations to make data-driven decisions and gain a competitive edge in the market.
Table 1: Neural Net As Applications
Industry | Application |
---|---|
Finance | Stock market prediction and algorithmic trading |
Healthcare | Medical image analysis for early disease detection |
Marketing | Targeted advertising campaigns and customer behavior prediction |
**The applications of neural nets are not limited to these industries only**, as their potential continues to expand as researchers and developers explore new possibilities.
Table 2: Benefits of Neural Net As
Advantages |
---|
Ability to analyze vast amounts of complex data and extract meaningful insights. |
Improved accuracy and prediction capabilities, surpassing traditional algorithms. |
Flexibility and adaptability, allowing continual learning and performance improvement. |
Automation of repetitive tasks, increasing efficiency and reducing human error. |
**Neural nets offer tremendous benefits to businesses and organizations**, enabling them to unlock the full potential of their data and make informed decisions.
Impact of Neural Net As
Neural net As have already made a significant impact across industries, with ongoing advancements and discoveries. As organizations adopt these technologies, they can:
- Improve operational efficiency through automation and enhanced decision-making processes.
- Enhance customer experience by delivering targeted, personalized solutions.
- Drive innovation and unlock new opportunities through data-driven insights.
**The rapid development and widespread adoption of neural net As**, coupled with ongoing research and advancements, continue to reshape industries and pave the way for a more intelligent future.
Table 3: Impact of Neural Net As
Impacts |
---|
Improved operational efficiency through automation and enhanced decision-making processes. |
Enhanced customer experience through targeted, personalized solutions. |
Driving innovation and unlocking new opportunities through data-driven insights. |
**The impact of neural net As will continue to expand and evolve**, driving advancements and transformations in diverse sectors, leading to a more intelligent and data-driven world.
Get Ready for the Neural Net As Revolution
As neural nets continue to evolve and demonstrate their capabilities, organizations will need to embrace these technological advancements to stay competitive. By harnessing the power of neural nets, businesses can elevate their decision-making processes, improve operational efficiency, and unlock new opportunities for growth.
**The time to embrace the neural net As revolution is now**, as these technologies reshape industries and open doors to a more intelligent and innovative future.
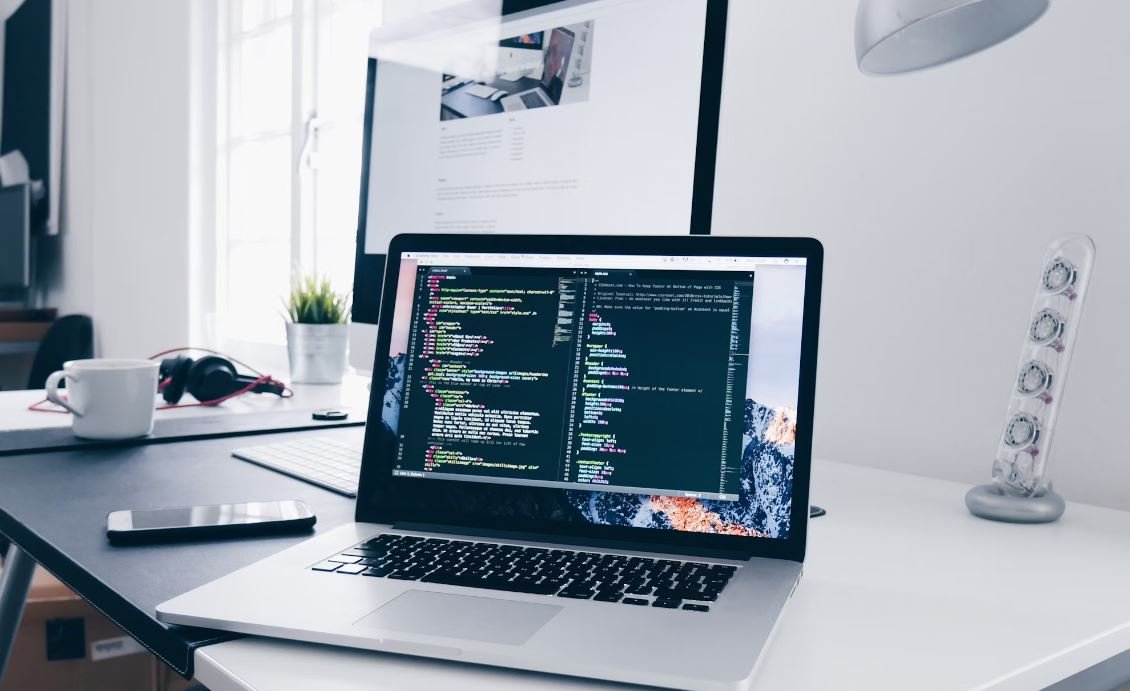
Neural Nets: Common Misconceptions
1. Neural nets are capable of human-like intelligence
One common misconception about neural nets is that they possess human-like intelligence. While neural nets have the ability to process large amounts of data and make predictions, they do not have consciousness or awareness like humans do.
- Neural nets lack consciousness and self-awareness
- They can only make predictions based on patterns in data
- They do not possess reasoning or abstract thinking abilities
2. Neural nets always produce accurate results
Another misconception is that neural nets always produce accurate results. Neural nets are not infallible and can make mistakes, especially when trained on insufficient or biased data. It is important to validate and evaluate the model’s predictions to ensure their reliability.
- Neural nets can produce false positives or false negatives
- Accuracy depends on the quality and diversity of training data
- Evaluation and validation are necessary to assess the model’s performance
3. Neural nets are a black box with no interpretability
There is a misconception that neural nets are completely opaque and lack interpretability. While it is true that complex neural nets can be challenging to interpret, there are techniques and methods available to gain insight into their decision-making process.
- Various methods and techniques exist to interpret neural nets
- Interpretability helps understand factors influencing predictions
- Interpretation may vary depending on the complexity of the model
4. Neural nets can replace human experts
It is incorrect to assume that neural nets can replace human experts entirely. While neural nets can automate certain tasks and provide valuable insights, they lack the domain knowledge and expertise possessed by human professionals.
- Human expertise is crucial for validating and interpreting model outputs
- Neural nets are tools to assist human decision-making, not replace it
- The collaboration between humans and neural nets can be powerful
5. Neural nets are always the best solution for every problem
Lastly, believing that neural nets are always the best solution for every problem is a common misconception. Neural nets excel in certain areas, but not all. Depending on the problem, other algorithms or methods may be more appropriate and yield better results.
- Consider other algorithms or methods that may be better suited for the problem
- Neural nets have specific strengths and weaknesses
- Context and problem domain should be carefully evaluated before choosing a solution
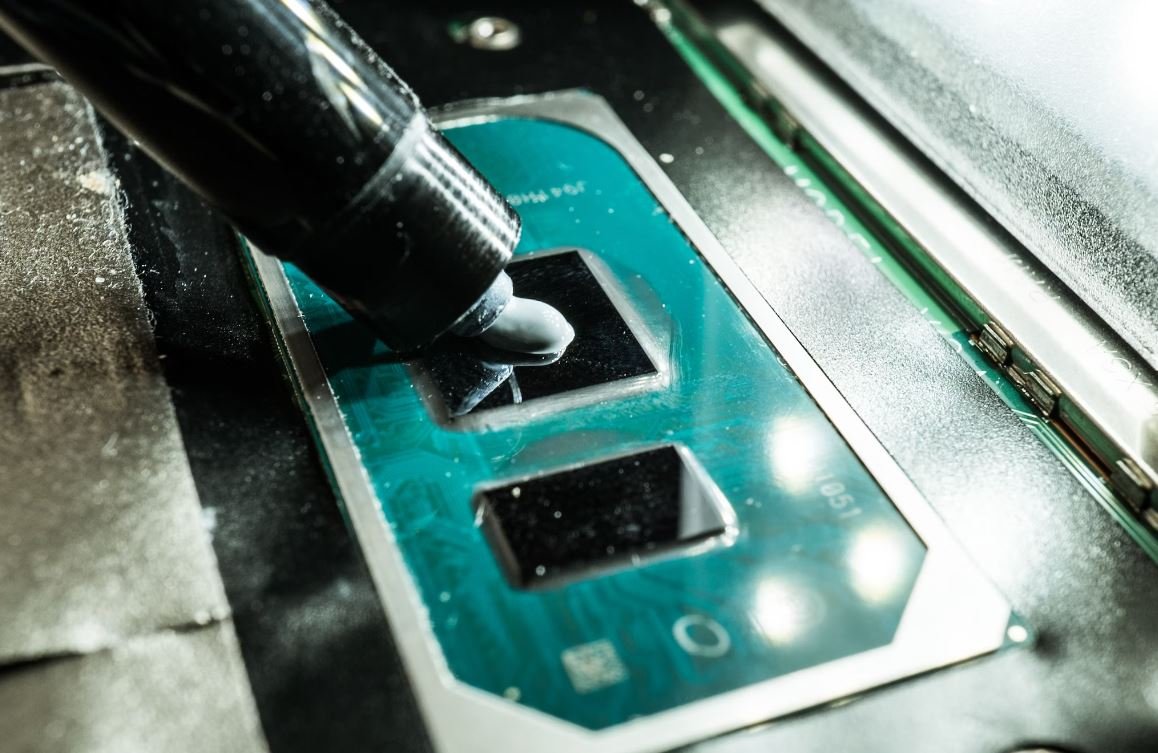
Introduction
Neural networks have revolutionized various fields, including artificial intelligence and machine learning. These computational models are designed to mimic the functioning of the human brain, enabling the processing of complex information and fostering advanced decision-making. In this article, we explore different aspects of neural networks and their remarkable applications through a series of interactive tables.
Table: Neural Net Architectures
Neural networks can vary in structure and architecture, catering to different applications and problem-solving scenarios. Here, we summarize various neural net architectures:
Architecture | Description |
---|---|
Feedforward Neural Network | Information flows in one direction, from input nodes to output nodes, without loops. |
Recurrent Neural Network | Allows feedback loops, enabling the network to retain and process sequential data. |
Convolutional Neural Network | Specializes in processing grid-like data, such as images, using convolutional layers. |
Generative Adversarial Network | Consists of two neural networks – a generator and a discriminator – competing against each other to create realistic data. |
Radial Basis Function Neural Network | Utilizes radial basis functions as activation functions, enabling it to approximate functions accurately. |
Table: Neural Net Applications
Neural networks have found diverse applications in various industries, empowering advanced problem-solving and decision-making. Explore some noteworthy applications below:
Application | Description |
---|---|
Image Classification | Neural networks can accurately classify images, enabling tasks like facial recognition and object identification. |
Natural Language Processing | With recurrent neural networks, machines can understand, process, and generate human-like language. |
Autonomous Vehicles | Neural networks enable self-driving cars to interpret sensory data and make real-time driving decisions. |
Medical Diagnosis | Artificial neural networks assist doctors in diagnosing diseases, analyzing symptoms, and predicting patient outcomes. |
Stock Market Prediction | Neural networks analyze market data and patterns to make predictions and aid in stock trading strategies. |
Table: Training Techniques
Training neural networks involves determining the optimal set of weights and biases. Various techniques and algorithms are employed for effective training:
Technique | Description |
---|---|
Backpropagation | An algorithm that adjusts weights backward, minimizing the difference between expected and predicted outputs. |
Genetic Algorithms | Imitates natural selection, evolving the neural network’s parameters through mutation and reproduction. |
Reinforcement Learning | Networks learn through trial and error, receiving feedback in the form of rewards or punishments for correct or incorrect predictions. |
Stochastic Gradient Descent | Optimizes the network by gradually adjusting the weights with small subsets of the training data. |
Simulated Annealing | Inspired by the cooling process of metal, this technique explores different weight configurations to find the best solution. |
Table: Advantages and Disadvantages
Like any technology, neural networks possess both strengths and limitations. Assess the advantages and disadvantages:
Advantages | Disadvantages |
---|---|
Flexible in handling complex data | Need significant computational resources |
Ability to learn and adapt autonomously | Require large amounts of labeled training data |
Fault-tolerant and capable of handling noisy input | Difficult to interpret inner workings and decision-making process |
Parallel processing enables faster calculations | May suffer from overfitting or underfitting |
Effective in solving problems with high-dimensional inputs | Can be prone to bias and ethical concerns |
Table: Neural Net Libraries
Developers keen on implementing neural networks can leverage various libraries and frameworks to simplify the process:
Library/Framework | Description |
---|---|
TensorFlow | A widely-used deep learning library with an extensive community and comprehensive documentation. |
PyTorch | Renowned for its dynamic computational graph and ease of use, PyTorch supports dynamic neural network construction. |
Keras | A high-level neural networks API that runs on top of TensorFlow, aiding rapid prototyping and experimentation. |
Caffe | A deep learning framework for training models quickly, focusing on computer vision tasks. |
Theano | Offers efficient numerical computation and supports the creation of both simple and complex neural networks. |
Table: Neural Net Innovations
Constant research and innovation drive the field of neural networks forward. Here are noteworthy recent innovations:
Innovation | Description |
---|---|
AlphaGo | DeepMind’s AlphaGo AI defeated world champion Go player Lee Sedol, advancing the field of reinforcement learning. |
OpenAI GPT-3 | The third iteration of the Generative Pre-trained Transformer produced by OpenAI, demonstrating remarkable natural language generation capabilities. |
Self-Driving Cars | Companies like Tesla and Waymo are making significant strides in developing autonomous vehicles using neural networks. |
Rosetta | Facebook’s Rosetta utilizes neural networks to understand and moderate content across multiple languages and platforms. |
Deepfake Detection | Researchers are actively developing neural networks to detect and combat deepfake videos, ensuring the integrity of visual media. |
Table: Neural Net Challenges
Building powerful and reliable neural networks poses several challenges that must be addressed to further advance the field:
Challenge | Description |
---|---|
Interpretability | Understanding and explaining the inner workings and decision-making processes of neural networks remains a challenge. |
Data Privacy and Security | As neural networks rely heavily on data, ensuring the privacy and security of user information becomes crucial. |
Computational Resources | Training and deploying large-scale neural networks necessitate immense computational power and energy consumption. |
Ethical Considerations | Neural networks raise ethical concerns regarding AI biases, potential job displacement, and societal impact. |
Generalizability | Making neural networks robust and capable of generalizing to diverse scenarios without overfitting remains a challenge. |
Conclusion
Neural networks have emerged as a powerful tool for tackling complex problems and improving decision-making across various domains. With applications ranging from image classification to medical diagnosis, these computational models continue to push the boundaries of what machines can achieve. Despite the challenges of interpretability, data privacy, and ethical considerations, the relentless efforts towards innovation are propelling neural networks towards a future where they transform industries and shape our everyday lives.
Neural Networks
Frequently Asked Questions
What is a neural network?
How does a neural network learn?
What are the applications of neural networks?
How do neural networks differ from traditional programming?
What are the different types of neural networks?
What is the role of activation functions in neural networks?
Can neural networks handle large datasets?
Are neural networks susceptible to overfitting?
What are the main challenges in training neural networks?
Can neural networks be combined with other machine learning techniques?