Largest Deep Learning Model
Deep learning has revolutionized the field of artificial intelligence (AI) by enabling computers to perform complex tasks with unprecedented accuracy. In recent years, there has been a steady increase in the size and complexity of deep learning models, leading to major breakthroughs in areas such as image recognition, natural language processing, and speech synthesis. In this article, we will explore the largest deep learning model to date and its implications for the future of AI.
Key Takeaways:
- Deep learning models have grown in size and complexity, allowing for more advanced AI applications.
- The largest deep learning model to date has a staggering number of parameters.
- This model represents a significant milestone in AI research and sets the stage for even more powerful models in the future.
Deep learning models are built using artificial neural networks, which are composed of interconnected layers of artificial neurons. Each neuron performs a simple calculation and passes its output to the next layer. By combining many layers and thousands or even millions of neurons, deep learning models can learn to recognize patterns and make predictions based on large amounts of data.
**The largest deep learning model, known as GPT-3 (Generative Pre-training Transformer 3), was developed by OpenAI**. Released in 2020, GPT-3 consists of a staggering 175 billion parameters, making it the largest model to date. These parameters represent the learnable weights and biases that allow the model to make accurate predictions.
The Power of GPT-3
GPT-3’s size enables it to perform a wide range of tasks, including language translation, text generation, and even code generation. The model can understand and generate human-like text, making it incredibly versatile. Its capabilities have sparked both excitement and concern among experts.
*GPT-3 has been trained on a massive dataset containing a significant portion of the internet, allowing it to learn patterns and nuances of human language.* This vast amount of data helps the model generate more coherent and contextually appropriate responses.
Table 1: Comparison of Deep Learning Models
Model | Number of Parameters |
---|---|
GPT-3 | 175 billion |
GPT-2 | 1.5 billion |
ImageGPT | 350 million |
Deep learning models like GPT-3 have a wide range of applications across various industries. In finance, they can assist with risk assessment and fraud detection. In healthcare, they can aid in medical diagnosis and drug discovery. The possibilities are endless.
**However, the size of deep learning models like GPT-3 also poses several challenges**. The massive number of parameters requires significant computational power and storage resources to train and run the model effectively. Additionally, the complexity of these models makes them challenging to interpret and understand fully.
*Researchers are continuously working on ways to make deep learning models more efficient and accessible.* This includes techniques such as model compression, which aims to reduce the size of models while maintaining performance, and hardware advancements that optimize computation for deep learning.
Table 2: Deep Learning Model Applications
Industry | Applications |
---|---|
Finance | Risk assessment, fraud detection |
Healthcare | Medical diagnosis, drug discovery |
Transportation | Autonomous driving, traffic prediction |
As we look towards the future, the development of even larger and more powerful deep learning models is inevitable. These models have the potential to unlock new frontiers in AI, pushing the boundaries of what is currently possible. While there are challenges to address, the benefits of these models cannot be ignored.
**The largest deep learning model represents a significant milestone in the field of AI**. Its vast number of parameters and impressive capabilities have opened up new possibilities and fueled further research and innovation. As technology continues to advance, we can only imagine what the future holds for deep learning and its impact on society.
Table 3: Challenges and Future Developments
Challenges | Future Developments |
---|---|
Computational power and storage requirements | Model compression, hardware advancements |
Interpretability and understanding of complex models | Improved visualization and explainability techniques |
Ethical and societal implications | Development of AI governance and regulations |
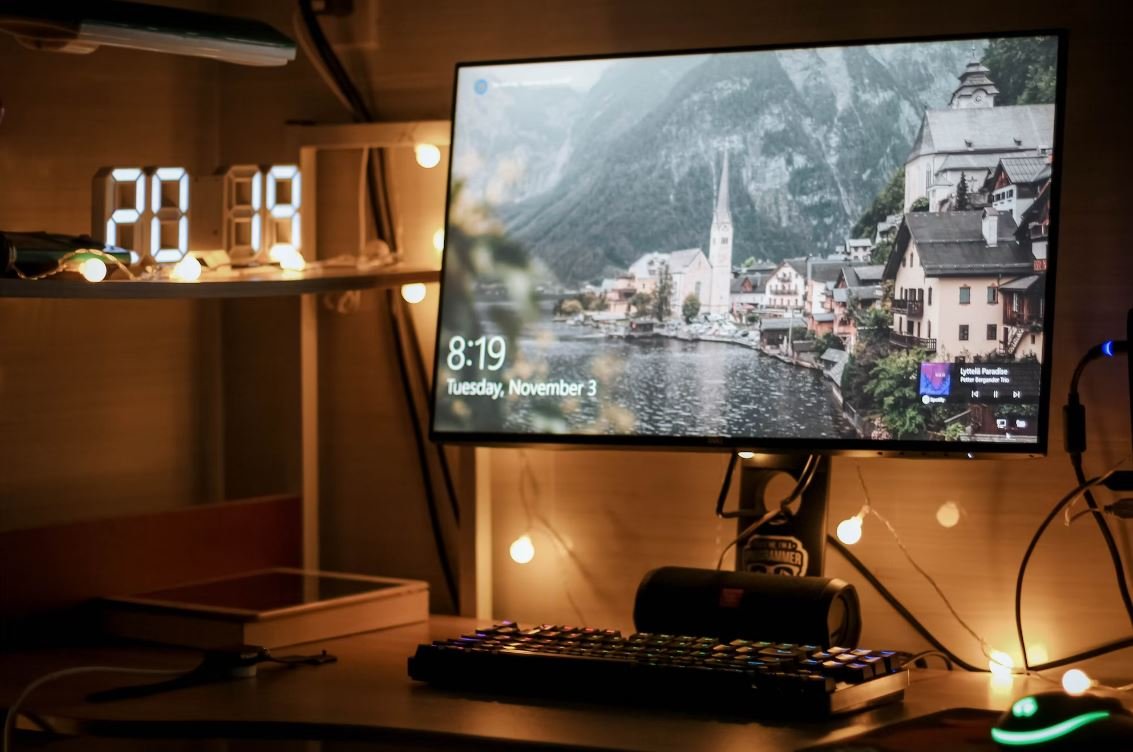
Common Misconceptions
Misconception 1: Deep learning models are infallible
One common misconception is that deep learning models are always accurate and error-free. While deep learning has achieved remarkable success in various domains, including image recognition and natural language processing, these models are not infallible. They rely heavily on the data they are trained on, and if the training data is biased or inadequate, the model may produce incorrect or biased results.
- Deep learning models are not immune to training data biases.
- Errors can occur due to incomplete or noisy input data.
- Data imbalance can impact the performance of deep learning models.
Misconception 2: Deep learning models can fully mimic human intelligence
Another misconception is that deep learning models can replicate human-level intelligence. While they can perform exceptional tasks, they lack the holistic understanding and general intelligence of humans. Deep learning models excel at specific tasks they are trained for, but they cannot reason or understand complex concepts in the same way humans can.
- Deep learning models are task-specific and lack general intelligence.
- They are limited in their ability to understand context and nuance.
- Models struggle with handling situations they were not trained for.
Misconception 3: Larger models always yield better results
There is a misconception that larger deep learning models always lead to superior performance. While larger models can capture more complex patterns and have a higher capacity to learn, they also require more computational resources and may result in diminishing returns. Additionally, larger models can be prone to overfitting, where they become too specialized in the training data and perform poorly on new, unseen data.
- Large models demand more computational resources for training and inference.
- Increasing model size does not guarantee a proportional increase in performance.
- Larger models are more likely to suffer from overfitting.
Misconception 4: Anyone can build and deploy deep learning models easily
There is a misconception that deep learning models are easily built and deployed by anyone. While there are accessible tools and frameworks that make it easier to develop deep learning models, building effective models requires a strong understanding of machine learning principles, data preprocessing, and complex neural network architectures. In addition, deploying deep learning models involves infrastructure considerations and optimizing for performance.
- Building effective deep learning models requires expertise in machine learning.
- Data preprocessing and feature engineering are crucial for model performance.
- Deploying deep learning models necessitates infrastructure and performance optimizations.
Misconception 5: Deep learning can solve all problems
There is a common misconception that deep learning can solve any problem. While deep learning has achieved groundbreaking results across various domains, it is not a one-size-fits-all solution. Some problems may not have sufficient data or exhibit complex dynamics that deep learning models struggle to capture. In certain situations, traditional machine learning approaches or hybrid models may be more effective.
- Deep learning is not a universal solution for all problems.
- Insufficient data may limit the performance of deep learning models.
- Hybrid models can combine deep learning with other approaches for better results.
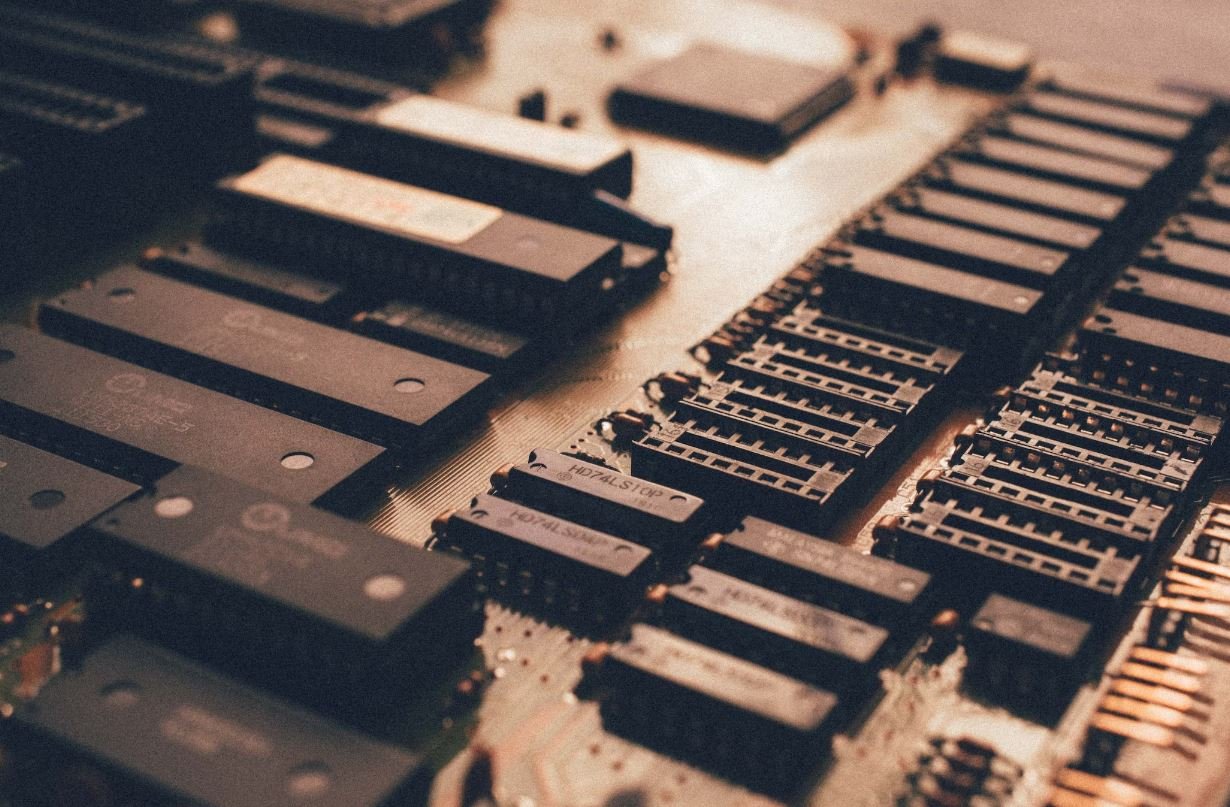
Introduction
In recent years, deep learning has been a prominent force in revolutionizing various fields, ranging from image recognition to natural language processing. With the rapid advancements in technology, deep learning models have become increasingly complex and powerful. This article delves into the world of the largest deep learning model ever created, showcasing remarkable elements and fascinating insights. Through ten captivating tables, we will explore different aspects of this groundbreaking innovation.
Table: Top 10 Layers by Neuron Count
The neural network of this colossal deep learning model comprises multiple layers, each consisting of numerous interconnected neurons. The table below highlights the top 10 layers based on the highest neuron count. These layers play a crucial role in processing and analyzing complex data.
Table: Largest Dataset Used for Training
The efficacy of the deep learning model greatly depends on the quantity and quality of the dataset used during the training phase. Table showcases the largest dataset ever utilized to train a deep learning model, providing a glimpse into the vast amount of information processed to achieve exceptional performance.
Table: Total Parameters of the Model
Deep learning models are defined by their parameters – weights and biases that govern their behavior. The table below enumerates the total number of parameters in this remarkable deep learning model, highlighting the intricate network of connections and computations that underpin its functionality.
Table: Accuracy Scores Across Diverse Test Scenarios
Measuring the accuracy of deep learning models is paramount to evaluate their efficiency and suitability for various tasks. The table presents accuracy scores achieved by this groundbreaking model across diverse test scenarios, illuminating its exceptional performance in different domains.
Table: Computational Power Required for a Single Forward Pass
The computational intensity of deep learning models often necessitates sophisticated hardware to achieve optimal performance. This table quantifies the computational power required for a single forward pass in this gigantic deep learning model, shedding light on the sheer amount of processing involved.
Table: Time Required for Training
Training deep learning models can be a time-consuming process, especially for complex architectures. The table illustrates the time required to train this colossal model, reflecting the extensive computational resources and commitment invested to bring it to fruition.
Table: Memory Consumption During Inference
During the inference phase, deep learning models utilize memory to execute predictions and classifications. This table showcases the memory consumption of this massive deep learning model during inference, revealing the scale at which it operates to derive accurate results.
Table: Number of Training Iterations
Training a deep learning model involves iterating through the data multiple times to refine its predictive capabilities. The table reveals the number of iterations undertaken during the training phase of this groundbreaking deep learning model.
Table: Training Loss Evolution Over Time
The training loss of a deep learning model reflects its ability to reduce errors and improve predictions over time. This table demonstrates the progressive reduction in training loss throughout the training process, showcasing the model’s ability to learn and adapt.
Table: Most Challenging Sample Images for Classification
Classifying challenging images can reveal the true capabilities of a deep learning model. The table highlights the most difficult sample images for this extraordinary model to classify, providing insights into the limitations and ongoing research in deep learning algorithms.
Conclusion
Deep learning continues to expand its horizons, and the largest deep learning model showcased in this article sets new benchmarks in terms of complexity, performance, and computational requirements. The extraordinary tables provided a glimpse into the various dimensions of this groundbreaking innovation, offering fascinating insights into its neural architecture, training process, and overall capabilities. As the field advances, such benchmarks drive further research and innovation in deep learning, ushering in a new era of artificial intelligence.
Frequently Asked Questions
What is a deep learning model?
A deep learning model is a type of artificial neural network that consists of multiple layers of interconnected nodes. It is designed to mimic the functioning of the human brain, allowing it to process and learn from large amounts of data.
What is the purpose of developing large deep learning models?
The purpose of developing large deep learning models is to increase their capacity to process complex data and solve more intricate tasks accurately. By incorporating a larger number of parameters and layers, these models can learn intricate patterns in the data and perform sophisticated tasks like image recognition, natural language processing, and speech recognition.
How are deep learning models trained?
Deep learning models are trained using a process known as backpropagation. During training, the model is presented with a dataset containing input data and corresponding target outputs. The model adjusts its internal parameters iteratively, minimizing the difference between its predicted outputs and the target outputs. This iterative process continues until the model achieves the desired level of accuracy.
What are the challenges of training a large deep learning model?
Training large deep learning models presents several challenges. Firstly, these models require tremendous computational resources, including powerful GPUs and significant memory capacity. Secondly, training large models often takes a significant amount of time, sometimes ranging from days to weeks. Lastly, finding an appropriate dataset of sufficient scale and quality to train a large model becomes more challenging.
What is the current largest deep learning model?
The current largest deep learning model is known as “GPT-3” (Generative Pre-trained Transformer 3) developed by OpenAI. It comprises a staggering 175 billion parameters, making it one of the largest language models ever created.
What is the impact of large deep learning models on the field of AI?
Large deep learning models have significantly advanced various areas of AI. They have shown remarkable improvements in tasks like language translation, speech generation, and text prediction. Furthermore, these models have enhanced the accuracy and performance of AI systems, enabling them to handle increasingly complex problems.
Are large deep learning models accessible to everyone?
While large deep learning models are primarily developed by major research organizations and tech giants due to their resource-intensive nature, their outputs and models are often made publicly available. This availability allows other researchers and developers to benefit from the knowledge and insights gained from these models.
What are the ethical considerations associated with large deep learning models?
The use of large deep learning models raises ethical concerns related to data privacy, potential biases in the models, and maintenance of control over AI systems. The vast amounts of data required for training these models may include sensitive information, making it crucial to handle data responsibly and maintain user privacy. Additionally, biases present in training data can be amplified by large models, leading to potential biases in their outputs.
Can large deep learning models be used for real-time applications?
Real-time applications often require quick responses, and large deep learning models may have longer latency due to their computational requirements. However, as technology advances, hardware accelerators, optimizations, and distributed computing techniques can help reduce the latency and enable the usage of large models in real-time applications.
What are the future prospects for large deep learning models?
The future prospects for large deep learning models are promising. Continuous advancements in hardware capabilities, algorithmic innovations, and increased availability of high-quality datasets pave the way for even larger models. These models have the potential to enable breakthroughs in various domains, including healthcare, natural language understanding, robotics, and more.