Input Data Description
The process of inputting data is an essential part of any data-driven project. Whether you are analyzing customer preferences, tracking sales, or conducting research, understanding how to properly describe input data is crucial to ensuring accurate and meaningful results. This article will discuss the importance of input data description, key considerations, and best practices to follow.
Key Takeaways:
- Input data description is crucial for accurate analysis.
- Key considerations include data format and quality.
- Best practices include documenting data sources and cleaning procedures.
**Input data description** refers to the process of defining and documenting the characteristics of the data that will be used for analysis. This includes information such as data format, structure, and quality. *Accurate input data description ensures that the analysis is based on reliable and relevant data, thus enhancing the validity and reliability of the results.*
When describing input data, there are several key considerations to keep in mind:
- **Data format:** Understanding the format of the data is essential. Is it structured, semi-structured, or unstructured? Is it in a tabular form, text, or multimedia? Properly identifying the format helps determine the most suitable analysis techniques.
- **Data quality:** Ensuring the quality of the input data is vital. Are there missing values, outliers, or inconsistencies? Cleaning or addressing these issues upfront can prevent biases and inaccuracies in the analysis.
- **Data sources:** Documenting the sources of the data is important for transparency and reproducibility. Include information such as the organization or individual that collected the data, the methodology used, and any limitations or constraints.
*Properly describing input data helps researchers, analysts, and decision-makers understand the appropriate use and limitations of the data.*
Best Practices in Input Data Description
Here are some best practices to follow when describing input data:
- **Document metadata:** Record important metadata such as creation date, time, and the person responsible for data collection or preparation.
- **Create a data dictionary:** A data dictionary provides a comprehensive description of the variables or field names in the dataset, including data type, units, and definitions.
- **Clean and preprocess data:** Before beginning the analysis, it is essential to clean and preprocess the data, addressing any missing values, outliers, or inconsistencies.
- **Maintain version control:** Keep track of changes to the data and maintain version control to ensure reproducibility and traceability of the analysis.
*By following these best practices, analysts can ensure that input data is accurately characterized and prepared for analysis.*
Tables
Data Type | Examples |
---|---|
Numerical | Age, height, temperature |
Categorical | Gender, color, product category |
Textual | Reviews, comments, tweets |
Data Cleaning Steps |
---|
Remove duplicate records |
Handle missing values |
Identify and remove outliers |
Address inconsistencies |
Metadata | Values |
---|---|
Creation Date | 2022-10-15 |
Time | 09:30 AM |
Data Collector | John Doe |
Conclusion
Input data description is a critical step in any data analysis process. By properly characterizing and documenting the input data, analysts can ensure accurate and reliable results. Considerations such as data format, quality, and sources help ensure the appropriate use of the data. Following best practices, such as documenting metadata, creating data dictionaries, and cleaning the data, further enhance the validity of the analysis. Remember, accurate input data description lays the foundation for meaningful insights and informed decision-making.
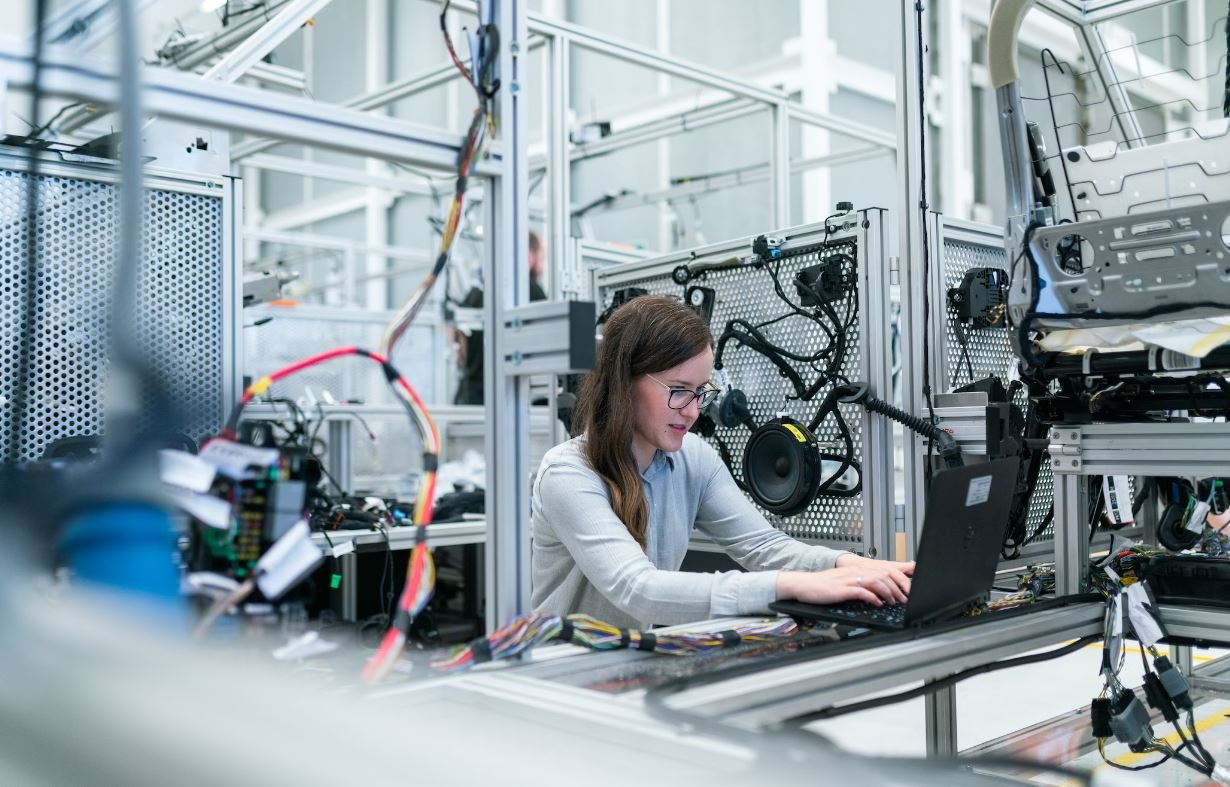
Common Misconceptions
Misconception 1: Input Data Description is optional
Many people believe that providing a detailed description of input data is not necessary. However, it is a crucial part of data analysis as it helps others understand the context and relevance of the data being used.
- Input data description provides background information about the data
- It helps users interpret the results accurately
- A lack of description may lead to misunderstandings or misinterpretations
Misconception 2: Input data description is only for complex datasets
Some individuals assume that input data description is only needed for complex datasets. This is not true as even simple data can benefit from a brief description to provide clarity and context.
- Providing a description helps others understand the purpose of the data
- It allows users to assess the quality and reliability of the data
- Even basic attributes like data source and collection method can be important
Misconception 3: Input data description is a one-time task
A common misconception is that input data description only needs to be done once. However, data can change over time, and it is essential to update the description when necessary.
- Changes in data sources or collection methods need to be documented
- Data updates or modifications may affect the interpretation of analysis results
- An up-to-date input data description ensures data integrity and reproducibility
Misconception 4: Input data description is only relevant for data analysts
Some people believe that input data description is only beneficial for data analysts or scientists. In reality, it is essential for anyone working with the data, including stakeholders, decision-makers, and even future researchers.
- Non-technical users can gain valuable insights from a well-described dataset
- Clear descriptions facilitate communication and collaboration among team members
- Data transparency builds trust with stakeholders
Misconception 5: Input data description is time-consuming and unnecessary
There is a misconception that creating an input data description is a time-consuming task that adds little value to the analysis process. However, investing time in creating a thorough description can save time in the long run and enhance the overall impact of the analysis.
- Well-documented input data can be reused for future projects
- Provides a foundation for data cleaning and preprocessing steps
- Reduces the risk of errors and misunderstandings in data analysis
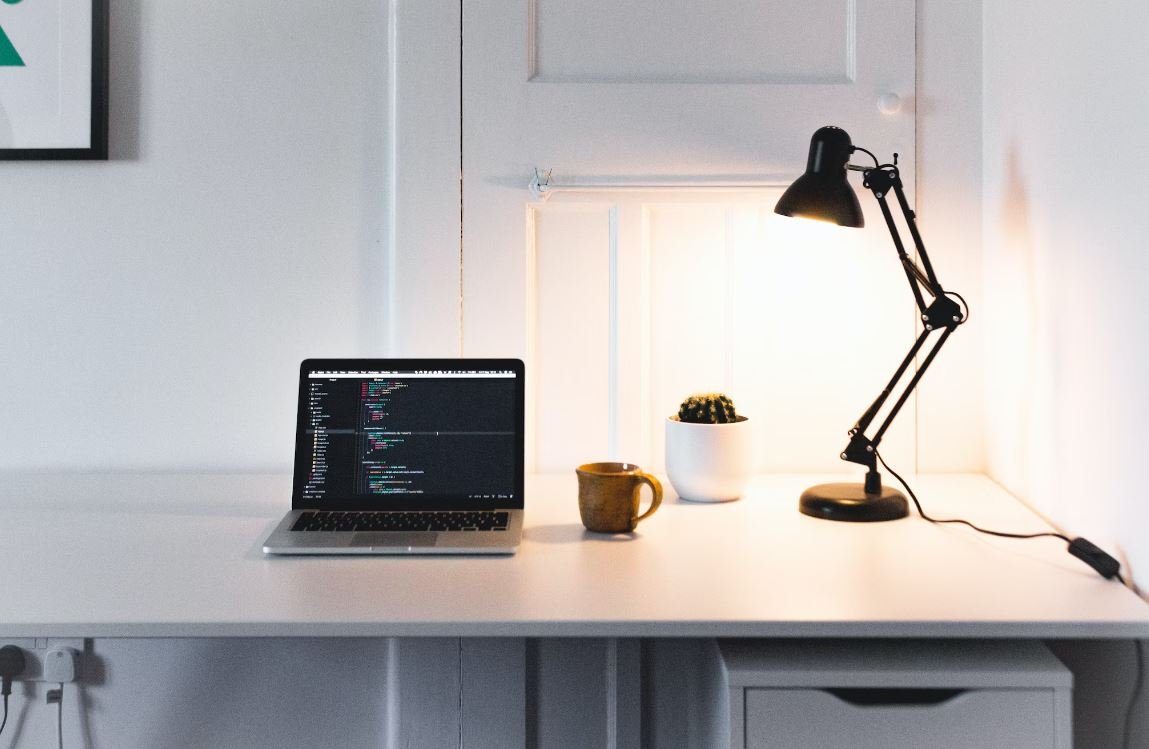
Data on Global Population
The following table provides an overview of the global population for the past 50 years, highlighting the growth rate and the projected population for the coming years.
Year | Population | Growth Rate |
---|---|---|
1970 | 3.7 billion | 1.98% |
1980 | 4.4 billion | 1.64% |
1990 | 5.3 billion | 1.33% |
2000 | 6.1 billion | 1.25% |
2010 | 6.9 billion | 1.18% |
2020 | 7.8 billion | 1.08% |
2030 (Projected) | 8.6 billion | 0.99% |
2040 (Projected) | 9.4 billion | 0.92% |
2050 (Projected) | 10.2 billion | 0.86% |
Cost of Living in Different Cities
Comparing the cost of living in various cities around the world can provide insights into their affordability and economic conditions. The table below displays the cost index for selected cities.
City | Cost Index |
---|---|
New York City, USA | 100 |
Tokyo, Japan | 94.19 |
London, United Kingdom | 86.48 |
Sydney, Australia | 81.75 |
Moscow, Russia | 46.21 |
Mumbai, India | 30.81 |
Cairo, Egypt | 24.67 |
Annual Rainfall in Different Regions
Understanding the annual rainfall in different regions helps analyze climate patterns and anticipate agricultural needs. The table below presents the average annual rainfall for selected regions.
Region | Rainfall (mm) |
---|---|
Amazon Rainforest, South America | 2,000 |
Sahara Desert, Africa | 100 |
Swiss Alps, Europe | 1,100 |
Mumbai, India | 2,500 |
Energy Consumption by Country
An analysis of energy consumption at a country level can reveal disparities and encourage efforts in sustainable practices. The following table showcases the energy consumption of selected countries in gigawatt-hours (GWh).
Country | Energy Consumption (GWh) |
---|---|
United States | 3,999,000 |
China | 6,949,000 |
South Africa | 228,000 |
Germany | 551,000 |
India | 1,151,000 |
World’s Tallest Buildings
Height is a significant metric when considering iconic skyscrapers worldwide. The table below exhibits the world’s tallest buildings and their respective heights in meters.
Building | Height (m) |
---|---|
Burj Khalifa, Dubai | 828 |
Shanghai Tower, China | 632 |
Abraj Al-Bait Clock Tower, Saudi Arabia | 601 |
Fastest Land Animals
Examining the speed of land animals evokes wonder and appreciation for their remarkable capabilities. The table below showcases the fastest land animals and their top speeds in kilometers per hour (km/h).
Animal | Top Speed (km/h) |
---|---|
Cheetah | 120 |
Pronghorn (Antelope) | 98 |
Lion | 80 |
Top Grossing Movies of All Time
Examining the highest-grossing movies provides a glimpse into popular culture and the film industry’s success. The following table highlights the top-grossing movies of all time, including their worldwide box office revenue in billions of dollars.
Movie | Box Office Revenue (Billions of $) |
---|---|
Avengers: Endgame | 2.798 |
Avatar | 2.790 |
Titanic | 2.195 |
Star Wars: The Force Awakens | 2.068 |
Life Expectancy by Country
Life expectancy indicates the average number of years a person can expect to live within a specific country. The table below presents the life expectancy for selected countries, offering insights into health and well-being.
Country | Life Expectancy (Years) |
---|---|
Japan | 84.6 |
Switzerland | 83.6 |
Australia | 83.0 |
United States | 79.1 |
India | 71.0 |
CO2 Emissions by Country
Assessing carbon dioxide (CO2) emissions by country raises awareness about environmental impacts and promotes sustainable practices. The table below displays the CO2 emissions of selected countries in metric tons per capita.
Country | CO2 Emissions (metric tons per capita) |
---|---|
Qatar | 36.8 |
United States | 16.2 |
China | 7.6 |
India | 1.9 |
In conclusion, the data presented in these tables provides valuable insights into various aspects of the world we live in. From population growth to climate patterns, economic conditions to cultural phenomena, and environmental impacts to health indicators, these figures help us understand our global reality and make informed decisions for the future.
Frequently Asked Questions
How do I input data?
To input data, you can use various methods depending on the context. You can manually enter data using a keyboard and mouse, import data from a file or database, or use automated data collection tools. The specific method will depend on the system or application you are working with.
What is the importance of data input description?
Data input description is crucial as it provides a clear understanding of the data being collected or entered. It helps users comprehend the format, structure, and any specific requirements of the input data. This information ensures accurate and consistent data entry, leading to reliable analysis, decision-making, and system functionality.
What is a data format?
A data format is a standardized way of representing and organizing information. It defines the structure, rules, and encoding used to store or transmit data. Common data formats include text files (such as CSV or JSON), binary files (like PDF or JPG), and specialized formats (e.g., XML, HTML) used for specific purposes.
What is meant by data validation?
Data validation refers to the process of checking and ensuring the accuracy, consistency, and integrity of input data. It involves applying predefined validation rules to detect errors, inconsistencies, or invalid data formats. Data validation helps maintain data quality and prevents unreliable or incorrect information from entering a system or database.
What are the different types of data validation methods?
There are several types of data validation methods, including:
- Format validation: Checking if the data adheres to a specific format (e.g., date format).
- Range validation: Verifying if the data falls within a defined range of values.
- Presence validation: Ensuring that required fields are not left blank.
- Unique value validation: Confirming that the data is unique within a specific context.
- Combination validation: Validating if the combination of multiple data elements is valid.
What is the significance of accurate data entry?
Accurate data entry is essential for valid analysis, decision-making, and system functionality. It ensures that the information used in reports, calculations, or database operations is reliable and free from errors. Accurate data entry helps avoid misleading conclusions, incorrect outputs, and potential adverse effects on business processes or customer experiences.
What is data integrity and why is it important?
Data integrity refers to the accuracy, completeness, and consistency of data throughout its lifecycle. It ensures that data remains unchanged and reliable over time. Data integrity is critical as it guarantees the trustworthiness and usability of data for various purposes, including auditing, compliance, and system operations. Maintaining data integrity is crucial for maintaining data quality within an organization.
What are the common challenges in data entry?
Common challenges in data entry include:
- Human error: Mistakes made during manual data entry.
- Data inconsistency: Inconsistencies or conflicts in data entered by different users.
- Data duplication: Entering the same data multiple times, resulting in redundancy.
- Data security: Protecting sensitive or confidential data during entry and storage.
- Data volume: Handling large amounts of data efficiently and accurately.
How can I improve the efficiency of data entry?
To enhance the efficiency of data entry, you can:
- Use automation tools: Utilize software and technologies that assist in data entry tasks.
- Provide training: Train users on proper data entry techniques and best practices.
- Implement data validation: Apply validation rules to minimize errors and ensure data accuracy.
- Streamline data collection processes: Simplify and optimize the data input workflow.
- Use data pre-filling: Pre-fill known data to reduce manual entry efforts.