Deep Learning Revolution
The field of deep learning has revolutionized the way we approach artificial intelligence and has incredible potential for various applications. With the continuous advancements in technology and the growing availability of computational power, deep learning has become a key driver in the rapid development of AI solutions. From image recognition to natural language processing, deep learning algorithms have shown remarkable results in a wide range of tasks.
Key Takeaways
- Deep learning is a groundbreaking approach in the field of artificial intelligence.
- It has revolutionized various applications, such as image recognition and natural language processing.
- The continuous advancements in technology have contributed to the rapid development of deep learning algorithms.
The Basics of Deep Learning
Deep learning is a subfield of machine learning that focuses on creating neural networks inspired by the structure and function of the human brain. These neural networks consist of multiple layers, allowing them to learn complex patterns and representations.
Deep learning algorithms use hierarchical layers of artificial neurons to process and understand data. Each layer receives input from the previous layer and extracts increasingly abstract features, ultimately leading to the generation of accurate predictions.
Applications of Deep Learning
Deep learning has found applications in various domains, bringing significant advancements to numerous industries. Here are some notable applications:
- Image recognition: *Deep learning models have achieved impressive accuracy in identifying objects, people, and scenes within images.*
- Natural language processing: *Deep learning techniques have improved language translation, sentiment analysis, and speech recognition.*
- Autonomous vehicles: *Deep learning plays a crucial role in enabling vehicles to perceive their surroundings and make real-time decisions.*
- Healthcare: *Deep learning models have been utilized to aid in medical diagnosis, drug discovery, and personalized treatment plans.*
Advancements and Challenges
The field of deep learning has witnessed remarkable advancements and faced several challenges:
- Computational power: *The availability of powerful hardware, such as GPUs, has accelerated the training process for deep learning models.*
- Data availability: *The abundance of labeled datasets has contributed to the success of deep learning algorithms.*
- Interpretability: *One challenge in deep learning is the lack of interpretability, making it difficult to understand how certain decisions are reached.*
- Generalization: *Deep learning models sometimes struggle to generalize well to unseen data, requiring careful validation and testing.*
Deep Learning Impact and Future
The impact of deep learning has been far-reaching, transforming numerous industries and pushing the boundaries of what AI can achieve. Its potential is immense, and it continues to evolve rapidly as researchers and developers explore new techniques and architectures.
*Deep learning is poised to further revolutionize fields such as robotics, cybersecurity, and finance, among others.* By continuously refining algorithms, addressing challenges, and leveraging the power of deep learning, we can expect even more groundbreaking applications in the future.
Industry | Applications |
---|---|
Healthcare | Medical diagnosis, drug discovery, personalized treatment plans |
Manufacturing | Quality control, predictive maintenance, robotic automation |
Finance | Algorithmic trading, fraud detection, risk assessment |
Aspect | Deep Learning | Traditional Machine Learning |
---|---|---|
Data requirements | Large labeled datasets | Smaller labeled datasets |
Feature engineering | Automatically learned | Manually crafted |
Performance | High accuracy | Dependent on feature engineering |
Framework | Popular Libraries |
---|---|
TensorFlow | Keras, TensorFlow.js, tflearn |
PyTorch | Torch, Caffe2 |
MXNet | Gluon, Keras-MXNet |
Deep learning has come a long way in transforming the capabilities of AI and has proven to be a game-changer across various industries. With ongoing research and technological advancements, the deep learning revolution is set to redefine the limits of artificial intelligence in the coming years.
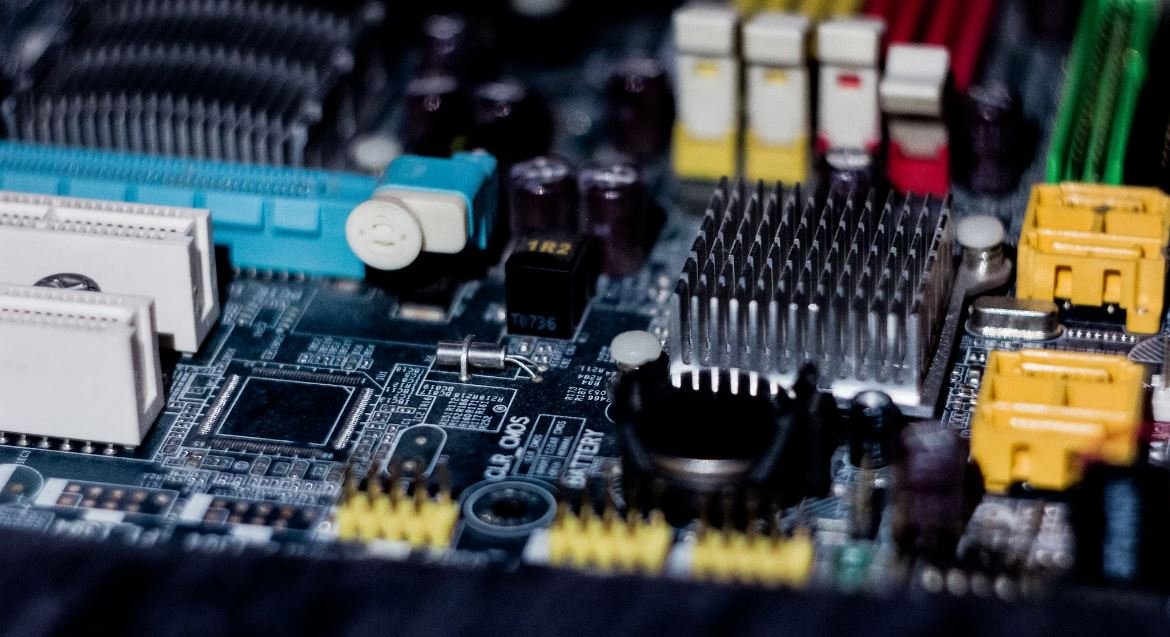
Deep Learning Revolution
Common Misconceptions
One common misconception about deep learning is that it is a solution that can solve any problem. However, deep learning algorithms are not universally applicable to all problems. They require substantial amounts of labeled data and may not perform well when faced with insufficient training samples. It is important to carefully consider the applicability and dataset availability before deciding to employ deep learning techniques.
- Deep learning is not a one-size-fits-all solution.
- Adequate labeled data is crucial for effective deep learning models.
- Deep learning may not work well with insufficient training samples.
Another misconception is that deep learning models are infallible and error-free. While deep learning algorithms have achieved remarkable successes in various domains, they are not immune to mistakes. Errors can occur due to factors such as flawed training data, biases in the data, or limitations in the model architecture. It is essential to thoroughly test and evaluate deep learning models to identify potential pitfalls and improve their performance.
- Deep learning models are prone to errors.
- Flawed training data can negatively impact deep learning performance.
- Model biases can influence the accuracy of deep learning predictions.
Many people believe that deep learning is only for advanced machine learning practitioners who possess extensive knowledge of complex mathematical concepts. While a solid understanding of mathematics and programming is beneficial, there are user-friendly deep learning libraries and frameworks that simplify the development process. These tools allow even individuals with limited experience to build and deploy deep learning models.
- Deep learning libraries and frameworks make it more accessible to non-experts.
- Advanced mathematical knowledge is not always a prerequisite for working with deep learning.
- User-friendly tools enable individuals with limited experience to utilize deep learning techniques.
Some people assume that deep learning can fully replace human intelligence. However, deep learning models excel in specific tasks that have been extensively trained on specific datasets. They lack the broader contextual understanding and intuition that humans possess. Deep learning is best seen as a tool that can augment human intelligence and assist in solving complex problems, rather than replacing human cognition entirely.
- Deep learning does not possess the comprehensive cognitive abilities of humans.
- Human contextual understanding and intuition are beyond the reach of deep learning.
- Deep learning is a tool that can complement human intelligence in solving intricate problems.
Finally, a misconception about deep learning is that it always requires massive amounts of computational power to train models. While it is true that deep learning models can be computationally intensive, there are methods to optimize performance and make efficient use of available resources. Techniques such as transfer learning or model compression can reduce the computational requirements without compromising the model’s accuracy.
- Deep learning can be optimized to utilize available computational resources more efficiently.
- Transfer learning and model compression are techniques that can alleviate computational demands.
- Not all deep learning models require massive computational power to train.
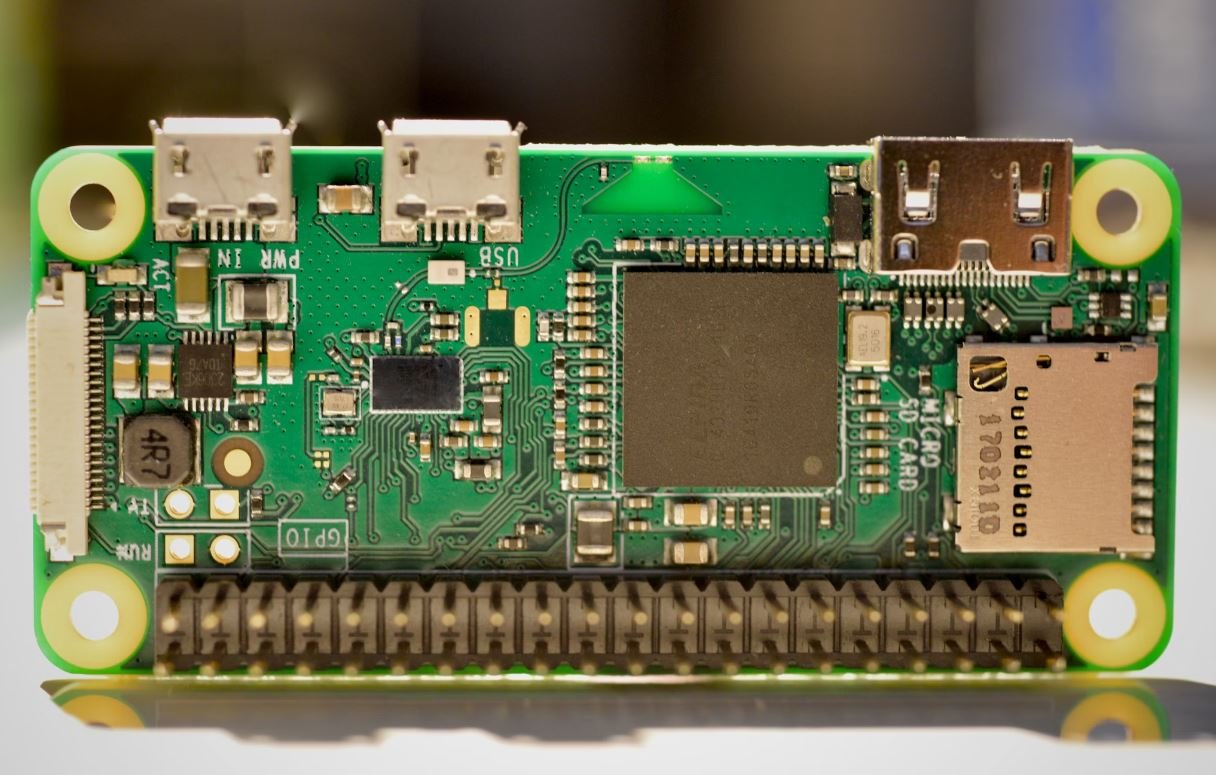
The Rise of Deep Learning
In recent years, deep learning has emerged as a powerful technique in the field of artificial intelligence. By mimicking the structure and functionality of the human brain, deep learning algorithms have revolutionized tasks such as image recognition, natural language processing, and speech recognition. This collection of tables explores various aspects of the deep learning revolution, highlighting its impact across different domains.
Enhancing Image Recognition Accuracy
Deep learning has significantly improved image recognition accuracy compared to traditional methods. This table showcases the accuracy percentages achieved by deep learning models in different image recognition tasks, demonstrating the superior performance of this technology.
Transforming Natural Language Processing
Deep learning has revolutionized natural language processing, enabling computers to understand, generate, and interpret human language. The table below presents the effectiveness of deep learning models in various natural language processing tasks, illustrating their ability to comprehend and manipulate textual data.
Advancing Speech Recognition Performance
Deep learning algorithms have significantly advanced speech recognition accuracy, making voice-controlled assistants and speech-to-text technology more feasible. The table illustrates the improvement in speech recognition performance achieved by deep learning models, emphasizing the increased accuracy and reliability of these systems.
Revolutionizing Autonomous Vehicles
Deep learning has played a pivotal role in the development of autonomous vehicles, enhancing their perception and decision-making capabilities. The following table presents the number of accidents per million miles driven comparing self-driving cars equipped with deep learning algorithms to traditional human-driven vehicles.
Improving Medical Diagnosis Models
The application of deep learning in the healthcare industry has led to significant improvements in medical diagnosis accuracy. This table highlights the performance of deep learning models in detecting various conditions, demonstrating their superior diagnostic capabilities compared to traditional methods.
Transforming Financial Market Predictions
Deep learning has revolutionized financial market predictions, enabling more accurate price forecasting and risk assessment. The table below presents the performance of deep learning models in predicting stock prices for different companies, showcasing their ability to capture market trends and patterns.
Enhancing Cybersecurity Measures
Deep learning has proven crucial in bolstering cybersecurity measures, improving the detection and prevention of cyber threats. This table displays the accuracy rates of deep learning models in identifying various types of malware and cyber attacks, demonstrating their effectiveness in safeguarding digital systems.
Accelerating Drug Discovery Process
Deep learning is revolutionizing the drug discovery process by accelerating the identification and optimization of potential drug candidates. The table below showcases the success rates of deep learning algorithms in predicting the effectiveness of different compounds, highlighting their contribution to rapid drug development.
Improving Customer Sentiment Analysis
Deep learning has significantly improved customer sentiment analysis, enabling businesses to gain insights into public opinion on their products or services. This table presents the accuracy rates of deep learning models in classifying sentiment in customer reviews, illustrating their effectiveness in understanding and responding to customer feedback.
Revolutionizing Academic Research
Deep learning has revolutionized academic research across various scientific disciplines. This table showcases the number of scholarly articles published on deep learning and its subfields over the past decade, portraying the extensive growth and widespread adoption of this cutting-edge technology within the research community.
In conclusion, the deep learning revolution has transformed numerous industries and domains, revolutionizing image recognition, natural language processing, speech recognition, autonomous vehicles, medical diagnosis, financial market predictions, cybersecurity, drug discovery, customer sentiment analysis, and academic research. With its ability to analyze vast amounts of data, deep learning continues to push the boundaries of what is possible in artificial intelligence, driving innovation and opening new possibilities.
Frequently Asked Questions
Deep Learning Revolution
What is deep learning?
Deep learning is a subset of machine learning that involves the use of artificial neural networks with multiple layers. It aims to model high-level abstractions in data by learning hierarchical representations of the data. These neural networks are composed of interconnected nodes, or artificial neurons, which can process and transfer information.
What are the applications of deep learning?
Deep learning has various applications across different fields. Some notable applications include computer vision (object detection, image classification), natural language processing (speech recognition, sentiment analysis), recommendation systems, autonomous vehicles, healthcare diagnostics, and financial market analysis.
What are the advantages of deep learning?
Deep learning has several advantages compared to traditional machine learning approaches. It can automatically learn features without explicitly designing them, handle large and complex datasets, and achieve state-of-the-art performance in various tasks. Additionally, deep learning models can generalize well and make accurate predictions on unseen data.
What are the challenges of deep learning?
Despite its successes, deep learning also has some challenges. One major challenge is the need for a large amount of labeled training data, which may not always be available. Training deep learning models can be computationally expensive and time-consuming. Interpreting and understanding the decisions made by deep neural networks, often referred to as the ‘black box’ problem, is another challenge.
How does deep learning differ from traditional machine learning?
Deep learning differs from traditional machine learning in its ability to automatically learn hierarchical representations of data. While traditional machine learning algorithms require hand-crafted feature engineering, deep learning models can learn features directly from raw data. Deep learning also shines in handling large-scale datasets and complex problems, where it has achieved state-of-the-art performance in various domains.
What are the different types of deep learning architectures?
There are several types of deep learning architectures, each serving different purposes. Some popular architectures include Convolutional Neural Networks (CNN) for computer vision tasks, Recurrent Neural Networks (RNN) for sequence data analysis, Generative Adversarial Networks (GAN) for generating new data, and Transformers for natural language processing tasks. Each architecture is designed to excel in specific areas.
How do I get started with deep learning?
To get started with deep learning, you can follow these steps: 1. Learn the basics of machine learning and neural networks. 2. Choose a deep learning framework such as TensorFlow or PyTorch. 3. Gain practical experience by working on small projects and gradually move on to more complex ones. 4. Engage with the deep learning community through forums, blogs, and online courses to stay updated with the latest advancements.
What resources are available to learn deep learning?
There are numerous resources available to learn deep learning. You can start with online tutorials, blogs, and video lectures on platforms like YouTube and Coursera. Books like ‘Deep Learning’ by Ian Goodfellow, Yoshua Bengio, and Aaron Courville provide in-depth knowledge. Additionally, online communities and forums like Stack Overflow and Reddit can provide support and answers to specific questions.
What are some real-world examples of deep learning applications?
Deep learning has been successfully applied in various real-world scenarios. Examples include self-driving cars, where deep learning is used for object detection and recognition. In healthcare, deep learning is utilized for medical image analysis and disease diagnosis. Virtual assistants like Siri and Alexa rely on deep learning for natural language understanding and speech recognition. Deep learning is also employed in recommendation systems by companies like Amazon and Netflix.
What is the future of deep learning?
The future of deep learning looks promising. Advancements in hardware technology, such as the development of specialized GPUs and TPUs, will facilitate faster and more efficient deep learning computations. Researchers are constantly working on improving deep learning algorithms, addressing challenges, and exploring new applications. As technology advances, deep learning is likely to have an even greater impact on various industries and domains.