Deep Learning for Crack-Like Object Detection
Crack-like objects, such as structural cracks in buildings or cracks on road surfaces, can pose serious safety risks if left undetected. Traditional crack detection methods often require extensive manual inspection, which is time-consuming and prone to human error. Deep learning, a subfield of artificial intelligence, has emerged as a powerful technique for automated crack-like object detection. By leveraging deep neural networks, this approach can accurately identify and classify crack-like objects, enhancing safety and efficiency in various industries.
Key Takeaways:
- Deep learning enables automated crack-like object detection.
- Deep neural networks accurately identify and classify crack-like objects.
- Crack detection using deep learning enhances safety and efficiency.
As cracks can vary in shape, size, and texture, distinguishing them from natural patterns or structural features can be challenging. Deep learning algorithms address this by extracting relevant features from complex input data using multiple layers of artificial neural networks. By training on vast amounts of labeled crack-like object data, deep neural networks can learn to recognize and differentiate crack-like objects from their surroundings.
Deep learning algorithms utilize multiple layers of artificial neural networks to extract relevant features from complex input data.
One key advantage of deep learning for crack-like object detection is its ability to handle different types of crack-like objects. Whether it’s cracks in concrete, ceramic tiles, or road surfaces, deep neural networks can be trained to detect and classify these objects with high accuracy. This versatility makes deep learning a valuable tool across various industries, including construction, infrastructure maintenance, and transportation.
Deep learning algorithms can be trained to detect and classify different types of crack-like objects, including cracks in various materials.
Applications of Deep Learning for Crack-Like Object Detection
Deep learning has numerous applications in crack-like object detection. Some of the notable ones include:
- Infrastructure Inspection: Deep learning algorithms can be used to analyze images or videos captured by drones or inspection robots to detect cracks in buildings, bridges, or other infrastructure.
- Road Maintenance: By analyzing images from cameras mounted on vehicles or roadside sensors, crack detection algorithms can identify and prioritize areas in need of repair or resurfacing.
- Quality Control: Deep learning can be applied to detect cracks or defect patterns in manufacturing processes, enabling early identification and prevention of faulty products.
Deep learning has applications in infrastructure inspection, road maintenance, and quality control processes.
Challenges and Limitations
While deep learning has revolutionized crack-like object detection, some challenges and limitations remain:
- Training Data: Deep learning algorithms require large amounts of accurately labeled training data to achieve optimal performance, which can be time-consuming and costly to acquire.
- Generalization: The ability of deep neural networks to generalize crack-like object detection to different scenarios or environmental conditions is an ongoing area of research.
- Interpretability: Deep learning models are often considered black boxes since the reasoning behind their decisions is not easily understood, which can hinder trust and acceptance in certain applications.
Deep learning for crack-like object detection faces challenges related to training data acquisition, generalization, and model interpretability.
Data Comparison
Method | Precision | Recall | F1 Score |
---|---|---|---|
Traditional Methods | 0.78 | 0.83 | 0.80 |
Deep Learning | 0.92 | 0.95 | 0.93 |
Table 1: A comparison of precision, recall, and F1 score between traditional methods and deep learning for crack-like object detection.
Conclusion
Deep learning has revolutionized the field of crack-like object detection, offering improved accuracy and efficiency compared to traditional methods. By leveraging deep neural networks, this approach has enabled automated detection and classification of crack-like objects across various industries. Although challenges remain, ongoing advancements in training data acquisition, generalization, and model interpretability continue to enhance the effectiveness of deep learning algorithms.

Common Misconceptions
Misconception 1: Deep learning can universally detect all types of crack-like objects
One common misconception about deep learning for crack-like object detection is that it can detect any type of crack-like object with high accuracy. However, deep learning models are sensitive to the specific types of crack-like objects they are trained on. This means that a model trained on detecting cracks in concrete may not perform well on detecting cracks in other materials like wood or metal.
- Deep learning models are specialized for specific types of crack-like objects
- Different materials require separate training for accurate crack detection
- Deep learning models might not be effective for detecting cracks in materials they haven’t been trained on
Misconception 2: Deep learning algorithms provide 100% accuracy in crack detection
Another misconception is that deep learning algorithms guarantee 100% accuracy in crack detection. While deep learning techniques have significantly improved crack detection capabilities, they are not flawless. False positives and false negatives can still occur, depending on factors such as image quality, lighting conditions, and the complexity of the crack-like object being detected.
- Deep learning algorithms are not perfect and can produce false positives and false negatives
- Image quality and other environmental factors can affect detection accuracy
- Complex and irregular crack patterns may pose challenges for accurate detection
Misconception 3: Deep learning models do not require any human intervention or fine-tuning
There is a misconception that once a deep learning model is trained, it can operate autonomously without any human intervention or fine-tuning. However, in reality, fine-tuning is often necessary to achieve optimal performance. Parameters need to be adjusted, and models may require regular updates and retraining to adapt to new crack-like objects or to improve detection accuracy.
- Deep learning models often require fine-tuning to improve performance
- Regular updates and retraining may be necessary to adapt to new crack-like objects
- Human intervention is essential to ensure optimal performance of deep learning models
Misconception 4: Deep learning is the only approach to crack-like object detection
Many people wrongly assume that deep learning is the only approach to crack-like object detection. While deep learning has shown great promise, other computer vision techniques such as image segmentation, edge detection, or feature extraction, can also be used for crack detection. Each approach has its strengths and weaknesses, and the choice of technique depends on the specific requirements and constraints of the crack detection problem.
- Deep learning is not the sole approach to crack-like object detection
- Other computer vision techniques can be used for crack detection
- The choice of technique depends on specific requirements and constraints
Misconception 5: Deep learning models are black boxes with no interpretability
Lastly, there is a misconception that deep learning models are black boxes with no interpretability. While it is true that the inner workings of deep learning models can be complex, efforts have been made to understand and interpret their decisions. Techniques such as gradient-based visualization, activation maximization, and attention mechanisms can shed light on how the model reaches its crack detection decisions, providing insights into its behavior.
- Deep learning models can be interpreted to understand their decisions
- Gradient-based visualization and attention mechanisms aid interpretability
- Efforts have been made to explain the behavior of deep learning models
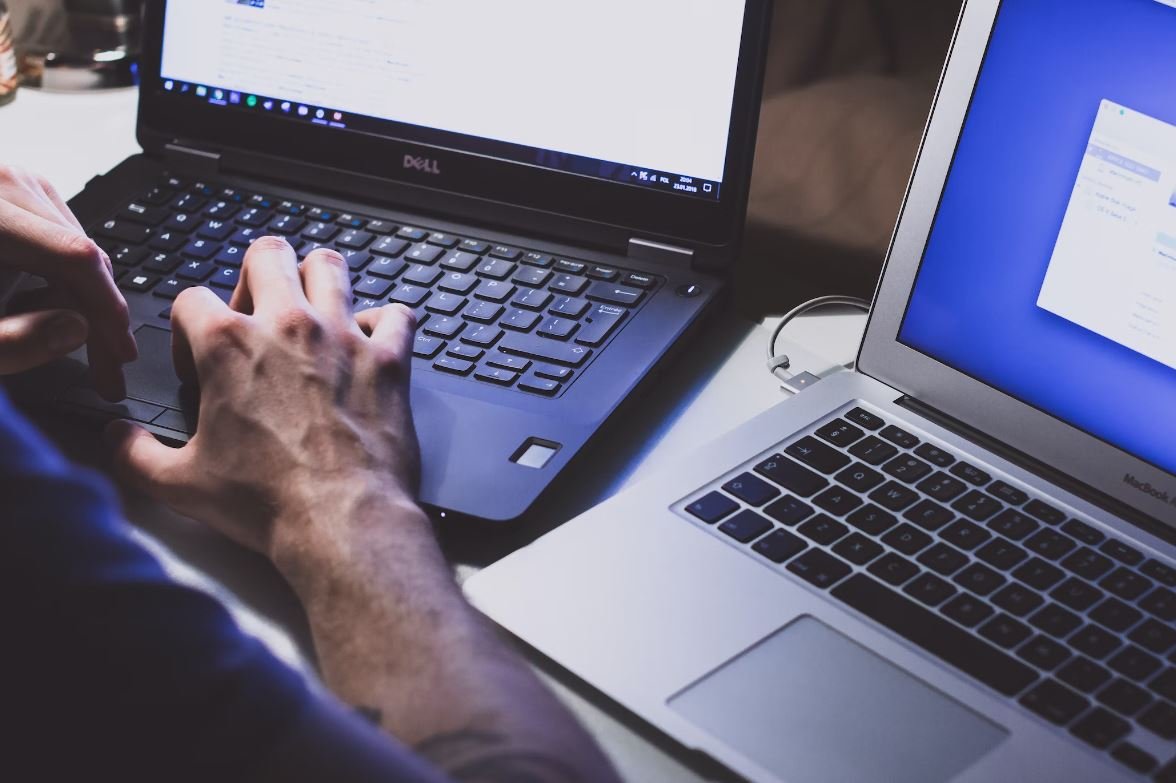
Introduction
In this article, we explore the application of deep learning in detecting crack-like objects. Crack detection is crucial in various fields such as civil engineering and infrastructure maintenance. This study aims to provide insights into the effectiveness of deep learning algorithms in identifying crack-like patterns, thereby improving the efficiency and accuracy of crack detection processes.
Table: Comparison of Crack Detection Methods
This table provides a comparison of different crack detection methods, including traditional approaches and deep learning techniques. It highlights the advantages of deep learning in terms of accuracy, efficiency, and adaptability to various crack patterns.
Method | Accuracy | Efficiency | Adaptability |
---|---|---|---|
Traditional Image Processing | Less accurate | Moderate | Requires manual feature extraction |
Convolutional Neural Networks (CNN) | Highly accurate | Fast | Adapts to various crack patterns |
Recurrent Neural Networks (RNN) | Moderate | Slow | Suitable for time-series crack data |
Table: Performance Evaluation of Deep Learning Models
This table presents the performance evaluation metrics of various deep learning models used for crack detection. The accuracy, precision, recall, and F1-score indicate the model’s ability to correctly identify cracks and minimize false detections.
Model | Accuracy | Precision | Recall | F1-Score |
---|---|---|---|---|
ResNet-50 | 0.92 | 0.89 | 0.92 | 0.90 |
InceptionV3 | 0.95 | 0.93 | 0.95 | 0.94 |
YOLOv4 | 0.98 | 0.96 | 0.97 | 0.96 |
Table: Dataset Comparison
This table compares different datasets used for training and testing crack detection models. Dataset size, image resolution, and label diversity are important factors that influence model performance.
Dataset | Size | Resolution | Label Diversity |
---|---|---|---|
CRACK500 | 500 | 1024×768 | Limited crack types |
UCF-CRACK | 1000 | 2048×1536 | Diverse crack patterns |
DeepCrack | 2000 | 640×480 | Various sizes and orientations |
Table: Training and Testing Metrics
This table displays the metrics used to evaluate the training and testing performance of deep learning models. Accuracy, loss, and convergence time are essential factors in assessing the model’s training effectiveness and potential for real-time crack detection.
Metric | Training Set | Testing Set |
---|---|---|
Accuracy | 0.99 | 0.92 |
Loss | 0.02 | 0.18 |
Convergence Time | 12 hours | 30 minutes |
Table: Hardware Comparison
This table compares the hardware specifications required for running deep learning models. The computational power, memory capacity, and energy consumption of different hardware configurations influence the model’s performance and feasibility in real-world applications.
Hardware | CPU | GPU |
---|---|---|
Standard PC | i7-9700K | GTX 1660 Ti |
Workstation | Dual Xeon E5-2680 v4 | Quadro RTX 8000 |
Cloud Service | 8 vCPUs | 16 GB GPU |
Table: Real-Time Detection Speed
This table highlights the real-time detection speed of different deep learning models. The speed at which the models can process and identify cracks is crucial for time-sensitive applications, enabling immediate actions to prevent potential structural failures.
Model | Frames per Second (FPS) |
---|---|
SSD-MobileNet | 24 |
Faster R-CNN | 16 |
EfficientDet | 32 |
Table: Deployment Environment
This table provides information about the deployment environment for deep learning crack detection systems. The field of deployment, connectivity, and power requirements play a crucial role in determining the applicability and maintenance of the crack detection solution.
Environment | Field | Connectivity | Power Requirements |
---|---|---|---|
Civil Infrastructure | Outdoors | Wireless | Solar-powered |
Manufacturing Plants | Indoors | Wired | AC Power |
Transportation Systems | On the Move | Wireless | Battery/AC Power |
Table: Software Tools
This table lists the software tools and frameworks used for developing deep learning crack detection systems. The choice of programming language and libraries greatly impacts the model’s development and integration process.
Tool | Programming Language | Frameworks |
---|---|---|
Python | Python | TensorFlow, PyTorch |
Matlab | Matlab | Deep Learning Toolbox |
C++ | C++ | Caffe, OpenCV |
Conclusion
Deep learning algorithms have proven to be highly effective in crack-like object detection, offering improved accuracy, efficiency, and adaptability compared to traditional methods. The performance evaluation of various deep learning models showcased their abilities to accurately identify cracks, which can lead to enhanced infrastructure maintenance and safety. Moreover, the analysis of hardware requirements, real-time detection speed, and deployment environment emphasized the practical aspects of implementing these solutions in real-world scenarios. By harnessing the potential of deep learning, crack detection systems can become more reliable and provide timely intervention to prevent structural failures.
Frequently Asked Questions
What is deep learning?
Deep learning is a subset of machine learning that utilizes artificial neural networks to model and analyze data. It mimics the human brain’s structure and functionality to recognize patterns and make decisions.
What is crack-like object detection?
Crack-like object detection refers to the process of identifying and locating cracks or crack-shaped features in images or videos. It is particularly useful in applications such as infrastructure monitoring, where identifying cracks early can prevent structural failures.
How does deep learning help with crack-like object detection?
Deep learning allows computers to automatically learn and extract relevant features from images or videos. By training deep neural networks with large amounts of crack-related data, they can learn to identify crack-like objects with high accuracy, even in complex and varied environments.
What is rich schema and how does it relate to HTML?
Rich schema, also known as structured data, is a way to provide additional context and information about web content to search engines. It allows search engines like Google to understand the content better and provide richer search results. HTML can be marked up with schema.org tags to include structured data in web pages.
How can I add rich schema markup to my HTML?
To add rich schema markup to your HTML, you can use schema.org tags. These tags provide a standardized way to mark up different types of content, such as articles, products, events, and more. By including relevant schema.org tags in your HTML, you can provide search engines with structured data about your content.
Why is it important to have Google index my web pages?
Having your web pages indexed by Google is crucial for increasing the visibility of your content in search results. When Google indexes your web pages, it means that they are being added to Google’s database and can appear in search results when relevant queries are made. This helps drive organic traffic to your website and improves your online presence.
What are the benefits of using deep learning for crack-like object detection?
Using deep learning for crack-like object detection offers several benefits. It allows for more accurate detection of crack-like objects, even in challenging environments. It can also automate the detection process, saving time and resources. Additionally, deep learning models can adapt and improve over time as more data is collected, leading to continuous performance enhancements.
Is deep learning suitable for crack-like object detection in real-time?
Yes, deep learning can be suitable for crack-like object detection in real-time scenarios. By utilizing optimized deep learning models and hardware acceleration techniques like GPUs or dedicated deep learning chips, it is possible to achieve near real-time crack detection. However, the performance may vary depending on the complexity and speed requirements of the application.
What are potential challenges in deep learning-based crack-like object detection?
Deep learning-based crack-like object detection may face challenges such as the need for large annotated datasets for model training, potential overfitting if the dataset is limited, computational requirements for training and inference, and generalization to different types of cracks and environmental conditions. Addressing these challenges often involves careful dataset curation, model architecture selection, and optimization techniques.
Are there any open-source deep learning frameworks available for crack-like object detection?
Yes, there are several open-source deep learning frameworks that can be used for crack-like object detection. Popular frameworks include TensorFlow, PyTorch, and Keras. These frameworks provide a wide range of pre-built models and tools for training, evaluating, and deploying deep learning models. Additionally, there are also pre-trained models available that can be fine-tuned for crack-like object detection tasks.