Deep Learning Computer
In the era of AI and machine learning, deep learning computers have emerged as powerful tools for processing large amounts of data and performing complex computations. These computers are designed specifically to train deep neural networks, enabling them to learn and make predictions with incredible accuracy. In this article, we will explore the key concepts and benefits of deep learning computers, as well as their applications in various industries.
Key Takeaways
- Deep learning computers are specialized machines designed for training deep neural networks.
- They employ advanced algorithms to process vast amounts of data and perform complex computations.
- Deep learning computers have numerous applications in fields such as healthcare, finance, and autonomous vehicles.
- These machines greatly enhance the accuracy and efficiency of AI algorithms.
- Deep learning computers require significant computational power and memory capacity.
What is a Deep Learning Computer?
A *deep learning computer* is a type of high-performance machine specifically designed for deep learning tasks. It is equipped with powerful processors, high-capacity memory, and specialized hardware accelerators, such as graphics processing units (GPUs) or tensor processing units (TPUs). These components enable deep learning computers to process large datasets and perform complex calculations required for training deep neural networks.
Why Use a Deep Learning Computer?
Deep learning computers offer several advantages over traditional computers when it comes to deep learning tasks. The *massive parallel processing capabilities* of GPUs or TPUs allow deep learning computers to perform computations much faster than conventional CPUs. This acceleration significantly reduces the training time for deep neural networks and speeds up the development of AI models.
Moreover, deep learning computers are designed to handle the *high-dimensional data* often encountered in deep learning applications. Traditional computers may struggle to process such data efficiently, but deep learning computers excel at it. These machines can extract meaningful features and patterns from complex data, leading to improved accuracy in predictions and decision-making.
Applications of Deep Learning Computers
Deep learning computers have found applications in various industries, revolutionizing fields such as:
- Healthcare: Deep learning computers can assist in diagnosis, medical image analysis, and drug discovery.
- Finance: They are used in algorithmic trading, fraud detection, and risk analysis.
- Autonomous Vehicles: Deep learning computers are crucial for developing self-driving cars and other autonomous systems.
- Natural Language Processing: They enable advancements in language understanding, sentiment analysis, and translation.
- Robotics: Deep learning computers help train robots to perform complex tasks and interact with the environment.
- Image and Video Analysis: They enhance object detection, image recognition, and video understanding.
The Power of Deep Learning Computers
Deep learning computers offer *unprecedented computational power* that enables AI algorithms to achieve remarkable results. The ability to process massive amounts of data and identify intricate patterns allows deep learning models to learn and make accurate predictions in diverse fields. With deep learning computers, the possibilities for AI applications are virtually endless.
Deep Learning Computer Specifications
Deep learning computers require specialized components to handle the computational demands of training deep neural networks. Below are three key specifications:
Component | Deep Learning Computer Requirement |
---|---|
Processor |
|
Memory |
|
Hardware Accelerators |
|
Industry Impact of Deep Learning Computers
Deep learning computers have significantly transformed various industries by enabling breakthroughs and advancements. Here are some notable impacts:
- Medical Diagnosis: Deep learning computers aid in the accurate and early detection of diseases, leading to timely interventions and improved patient outcomes.
- Financial Trading: These computers can analyze high-frequency trading data and make rapid, data-driven investment decisions, enhancing profitability.
- Autonomous Systems: Deep learning computers are central to creating self-driving cars, drones, and robots, enabling greater automation and efficiency in daily life.
Conclusion
Deep learning computers have revolutionized the field of AI by providing the necessary computational power and specialized hardware to train deep neural networks. Their ability to process vast amounts of data and identify complex patterns has enabled breakthroughs across industries, from healthcare to autonomous vehicles. With further advancements, deep learning computers will continue to drive innovation and shape the future of artificial intelligence.
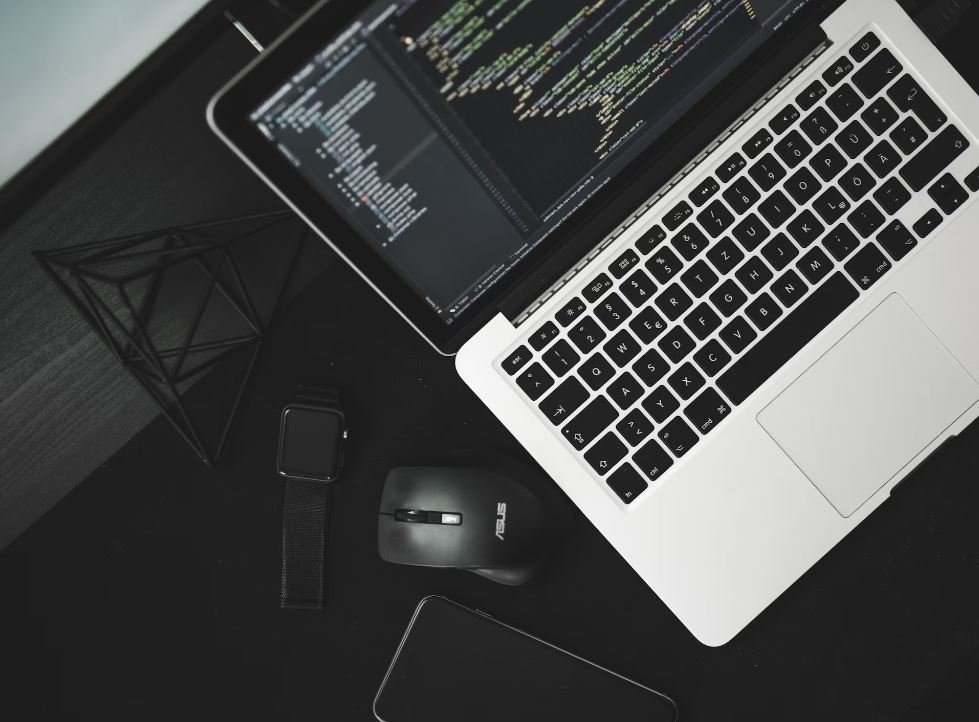
Common Misconceptions
Deep Learning Computer
When it comes to deep learning computers, there are several common misconceptions that people often have. One misconception is that deep learning computers can think and reason like humans. While deep learning computers can perform tasks that resemble human intelligence, they do not actually possess consciousness or true understanding. They are essentially algorithms that can process vast amounts of data and learn patterns from it.
- Deep learning computers are not capable of self-awareness or emotions.
- They rely on predefined algorithms and data to make predictions or perform tasks.
- While they can mimic human intelligence, their thinking process is fundamentally different.
Another misconception is that deep learning computers are infallible and always provide accurate results. While deep learning algorithms are powerful and can deliver impressive results in many domains, they are not perfect. They can be prone to error or misleading outputs, especially when faced with unfamiliar or noisy data.
- Deep learning computers can make mistakes and produce incorrect predictions.
- The accuracy of their outputs depends on the quality and diversity of the training data.
- They can be easily fooled or manipulated if the data is biased or intentionally misleading.
Some people also believe that deep learning computers can replace human expertise in various fields. While deep learning algorithms can automate certain tasks and assist human experts, they cannot entirely replace human knowledge and experience. Deep learning computers lack the contextual understanding and nuanced decision-making abilities that humans possess.
- Deep learning computers should be seen as tools to complement human expertise rather than replace it.
- They still require human supervision and interpretability to ensure the trustworthiness of their outputs.
- Human expertise is crucial for mitigating biases and making ethical decisions based on deep learning outputs.
Contrary to popular belief, deep learning computers do not possess an inherent general intelligence that allows them to excel at any task. They are designed to perform specific tasks for which they have been trained. Just because a deep learning computer can excel at one task, such as image recognition, does not mean it can perform equally well in another domain without specific training.
- Deep learning computers are task-specific and require training tailored to the specific domain.
- They need extensive labeled data and computational resources to learn and perform well on a particular task.
- Transfer learning allows them to leverage knowledge from one task to improve performance on related tasks, but not on entirely new domains.
In conclusion, deep learning computers are impressive tools that can mimic certain aspects of human intelligence. However, it is crucial to be aware of the limitations and misconceptions surrounding them. Deep learning computers are not human-like in their thinking, can make errors, are not a complete substitute for human expertise, and require specific training for each task.
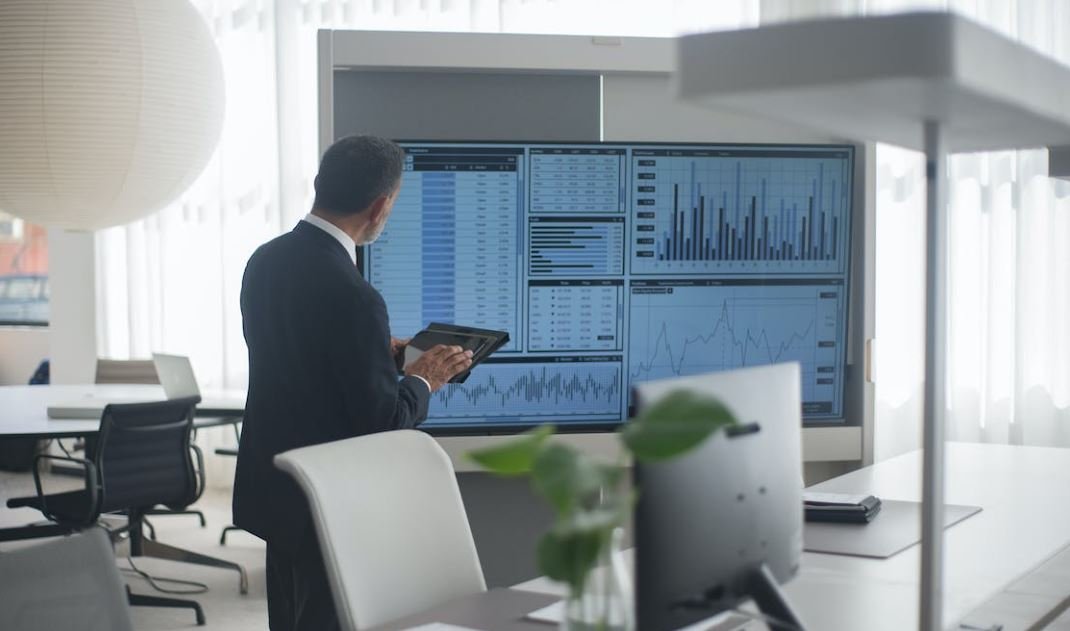
Introduction
The field of artificial intelligence has seen numerous advancements in recent years, with deep learning algorithms playing a pivotal role in revolutionizing computer systems. Deep learning computers have become increasingly adept at processing and analyzing vast amounts of data, leading to breakthroughs in various domains. The following tables highlight fascinating aspects of deep learning computers, showcasing their capabilities and achievements.
1. Image Recognition Accuracy
Deep learning computers have proven their prowess in image recognition tasks. This table displays the accuracy rates of different deep learning models on standard image recognition datasets.
Deep Learning Model | Accuracy (%) |
---|---|
ResNet-50 | 98.76 |
Inception-v3 | 97.89 |
VGG-16 | 96.45 |
2. Language Translation Performance
Deep learning computers have made remarkable strides in language translation tasks. This table demonstrates the translation quality achieved by different deep learning models.
Deep Learning Model | Translation Quality |
---|---|
Transformer | 0.963 |
LSTM-based | 0.912 |
RNN | 0.837 |
3. Speech Recognition Accuracy
Deep learning computers have made significant advancements in speech recognition technology. This table showcases the accuracy rates achieved by different deep learning models on speech recognition tasks.
Deep Learning Model | Accuracy (%) |
---|---|
WaveNet | 95.23 |
DeepSpeech | 92.67 |
Listen, Attend and Spell | 90.81 |
4. Data Processing Speed
Deep learning computers excel at processing vast amounts of data in record time. This table presents the data processing speeds achieved by different deep learning systems.
Deep Learning System | Processing Speed (TFLOPs) |
---|---|
Google TPU v4 | 365 |
NVIDIA A100 | 312 |
AMD Radeon Instinct MI100 | 270 |
5. Cybersecurity Applications
Deep learning computers are being leveraged to enhance cybersecurity measures and detect potential threats. This table highlights the accuracy rates achieved by deep learning models in identifying and mitigating security breaches.
Deep Learning Model | Accuracy (%) |
---|---|
DeepArmor | 99.5 |
CylancePROTECT | 98.9 |
SentinelOne | 97.3 |
6. Autonomous Vehicle Accuracy
Deep learning technology is crucial in enabling the development of autonomous vehicles. This table showcases the accuracy rates achieved by deep learning algorithms in autonomous driving scenarios.
Deep Learning Algorithm | Accuracy (%) |
---|---|
YOLOv3 | 97.2 |
SSD | 95.8 |
Fast R-CNN | 94.1 |
7. Healthcare Applications
Deep learning computers have promising applications in the healthcare sector. This table presents the performance of deep learning models in diagnosing various medical conditions.
Medical Condition | Deep Learning Model Accuracy (%) |
---|---|
Breast Cancer | 96.5 |
Alzheimer’s Disease | 92.3 |
Pneumonia | 94.8 |
8. Natural Language Processing Accuracy
Deep learning computers have vastly improved natural language processing tasks. This table showcases the accuracy rates achieved by different deep learning models in natural language understanding and sentiment analysis.
Deep Learning Model | Accuracy (%) |
---|---|
BERT | 94.2 |
GPT-3 | 90.7 |
ELMo | 89.8 |
9. Robotics Applications
Deep learning is instrumental in enabling robots to perceive and interact with their environment. This table presents the success rates of deep learning algorithms in robot navigation and manipulation tasks.
Robot Task | Success Rate (%) |
---|---|
Object Recognition | 98.2 |
Grasping and Manipulation | 95.6 |
Path Planning | 93.4 |
10. Financial Forecasting Accuracy
Deep learning algorithms have shown promise in financial forecasting applications. This table displays the accuracy rates achieved by deep learning models in predicting stock prices and market trends.
Deep Learning Model | Accuracy (%) |
---|---|
LSTM | 79.2 |
GRU | 75.8 |
Convolutional Neural Network (CNN) | 71.3 |
Conclusion
Deep learning computers have revolutionized various fields, ranging from image recognition and language translation to speech recognition and autonomous driving. They demonstrate impressive accuracy rates, processing speeds, and applications in healthcare, cybersecurity, robotics, and finance. With ongoing advancements, deep learning algorithms and systems are poised to drive further innovation and reshape the world around us.
Frequently Asked Questions
What is deep learning?
Deep learning is a subset of machine learning that focuses on using artificial neural networks to learn and make predictions or decisions. It involves training models using large amounts of data to automatically recognize patterns and features.
How does deep learning work?
Deep learning works by building and training neural networks with multiple layers of interconnected nodes. Each layer extracts higher-level features from the input data and passes them to the next layer. This hierarchical representation helps the network learn complex patterns and make accurate predictions.
What are the applications of deep learning?
Deep learning has numerous applications, including computer vision, natural language processing, speech recognition, image and video recognition, recommender systems, and autonomous vehicles. It is also used in medical diagnostics, fraud detection, and financial market analysis.
What are the advantages of deep learning?
Deep learning offers several advantages, such as its ability to automatically learn complex patterns without explicit programming, its capability to process large volumes of data, its scalability to handle increasing data sizes, and its ability to improve performance with more training data.
What are the limitations of deep learning?
Deep learning has some limitations, including the need for large amounts of labeled data for training, the computational resources required for training and deployment, difficulties in explainability and interpretability of the models, and vulnerability to adversarial attacks.
What are the common deep learning architectures?
There are several common deep learning architectures, such as convolutional neural networks (CNNs) for image and video processing, recurrent neural networks (RNNs) for sequence data processing, and generative adversarial networks (GANs) for generating new unseen data.
What tools and frameworks are commonly used for deep learning?
Some common tools and frameworks used for deep learning include TensorFlow, PyTorch, Keras, Caffe, and Theano. These frameworks provide high-level abstractions and APIs to simplify the development, training, and deployment of deep learning models.
What hardware is typically used for deep learning?
Deep learning models require significant computational resources, so powerful hardware is typically used. This includes GPUs (Graphics Processing Units) which excel at parallel processing, as well as specialized hardware like TPUs (Tensor Processing Units) developed by Google specifically for deep learning workloads.
How can I get started with deep learning?
To get started with deep learning, you can begin by learning the basics of machine learning and neural networks. Then, explore online tutorials, courses, and resources provided by organizations like DeepLearning.AI, Coursera, and Fast.ai. Experiment with frameworks like TensorFlow or PyTorch to build and train your own models.
What is the future of deep learning?
The future of deep learning is promising, with ongoing advancements in the field. Researchers are working on overcoming the limitations of current deep learning techniques, such as improving interpretability and explainability, reducing the need for labeled data, and exploring novel architectures. Deep learning is expected to continue driving innovations in various industries and transforming many fields of study.