Deep Learning and Financial Stability
Deep learning, a subfield of machine learning, has gained significant attention in recent years for its ability to process and analyze vast amounts of data with minimal human intervention. Financial stability, on the other hand, refers to the ability of a financial system to withstand shocks and disruptions, providing a solid foundation for economic growth. In this article, we will explore the intersection of deep learning and financial stability, and how deep learning techniques can be leveraged to enhance the stability of financial systems.
Key Takeaways
- Deep learning offers immense potential to strengthen financial stability.
- By analyzing large datasets, deep learning algorithms can identify patterns and insights that may not be apparent through traditional methods.
- Applying deep learning in financial institutions can improve risk management and prediction capabilities.
Deep learning algorithms, such as artificial neural networks, excel at extracting valuable information from complex datasets. They can process vast amounts of structured and unstructured data, ranging from market prices and news articles to social media feeds and even audiovisual content. *These algorithms can identify hidden relationships and patterns, providing a more comprehensive understanding of financial markets and their underlying dynamics.*
In the realm of financial stability, the ability to predict and address potential risks is of paramount importance. Deep learning models can analyze historical financial data to gauge the stability of financial institutions and detect early warning signs of potential crises. *By incorporating various financial indicators and macroeconomic factors, deep learning algorithms can provide more accurate risk assessments and predictions than traditional methods.* These models can help regulators and policymakers mitigate risks and take proactive measures to maintain stability in the financial system.
Enhancing Risk Management
Deep learning can significantly enhance risk management practices in financial institutions. By analyzing vast datasets, these algorithms can identify patterns and anomalies that may indicate potential security breaches or fraudulent activities. They can also detect unusual market behaviors or emerging risks that traditional risk models might miss. Furthermore, deep learning algorithms can continuously learn and adapt to changing market dynamics, improving their ability to detect and respond to new risks. *This adaptive capability makes deep learning particularly valuable in an ever-evolving financial landscape.*
Another area where deep learning can improve risk management is credit assessment. *By analyzing various data sources, including credit scores, transaction history, and alternative data such as social media behavior, deep learning algorithms can provide more accurate credit risk assessments.* These models can help banks and other financial institutions make more informed lending decisions and minimize credit losses.
Tables
Financial Indicator | Deep Learning Method | Benefits |
---|---|---|
Market prices | Recurrent Neural Networks (RNNs) | Predict short-term market trends |
News articles | Convolutional Neural Networks (CNNs) | Identify sentiment and relevant market factors |
Social media feeds | Long Short-Term Memory (LSTM) networks | Detect emerging market behaviors and sentiment |
Improving Financial Predictions
Deep learning algorithms can also be utilized to improve financial predictions, such as stock market forecasting and asset price movements. Traditional prediction models often rely on simplistic assumptions or predetermined statistical models, which may fail to capture the complex dynamics of financial markets. *In contrast, deep learning models can automatically extract features from raw data, allowing them to adapt and learn from changing market conditions.* This adaptability enables deep learning algorithms to outperform traditional methods in terms of prediction accuracy and robustness.
Additionally, deep learning models can be combined with other advanced techniques, such as reinforcement learning, to optimize trading strategies. *By leveraging vast amounts of historical data, deep reinforcement learning can learn to make optimal trading decisions under various market conditions.* This integration of deep learning and reinforcement learning has the potential to revolutionize investment strategies and risk management techniques in the financial industry.
Conclusion
Deep learning holds immense potential for enhancing financial stability and risk management. By analyzing vast amounts of data and identifying hidden patterns, deep learning algorithms can provide more accurate risk assessments, detect emerging risks, and improve financial predictions in a rapidly changing market landscape. The application of deep learning in financial institutions can help strengthen the stability of financial systems and contribute to long-term economic growth.

Common Misconceptions
Misconception 1: Deep Learning is a Black Box
One common misconception about deep learning is that it is a black box that produces results without any explanation. While deep learning models can indeed be complex and non-linear, efforts have been made to interpret their decisions.
- Deep learning models often employ techniques such as layer-wise relevance propagation to provide insights into their decision-making process.
- Researchers are actively working on developing algorithms and techniques for interpreting deep learning models to increase their transparency.
- Deep learning models can also be combined with traditional machine learning techniques to enhance transparency and interpretability.
Misconception 2: Deep Learning is Always Superior to Traditional Methods
There is a common belief that deep learning always outperforms traditional machine learning methods in all scenarios. While deep learning has achieved remarkable success in various domains, it is not always the best approach.
- For certain tasks with limited data, traditional machine learning methods might be more suitable as they don’t require a large amount of labeled data for training.
- Deep learning models can be computationally expensive and require substantial computational resources, which may not be feasible for all applications.
- In some cases, linear or shallow models might still yield competitive results without the complexity of deep neural networks.
Misconception 3: Deep Learning Algorithms Can Predict the Future
Another misconception is that deep learning algorithms have the ability to predict the future accurately. While deep learning models are effective at identifying patterns and making predictions based on historical data, they cannot foresee unforeseeable events or accurately predict complex future outcomes.
- Deep learning models heavily rely on historical data, and their predictions are based on patterns found in that data.
- Factors such as market fluctuations, political events, and technological advancements can have a significant impact on financial stability.
- Deep learning models can help provide insights and probabilities, but they cannot guarantee precise future predictions in unpredictable markets.
Misconception 4: Deep Learning Eliminates the Need for Human Intervention
Many people mistakenly believe that deep learning can replace human intervention entirely in financial stability management. While deep learning can automate certain tasks and improve efficiency, human expertise and decision-making remain essential in ensuring financial stability.
- Deep learning models require careful setup, tuning, and validation by human experts to ensure their accuracy and reliability.
- Human intervention is still essential for interpreting and acting upon the insights generated by deep learning models.
- Deep learning models are not able to take into account the constantly changing market dynamics and may require human intervention to adapt and update the models accordingly.
Misconception 5: Deep Learning is a Magic Solution for Financial Stability
There is a misconception that deep learning algorithms alone can solve all problems related to financial stability. However, deep learning is just one tool in a broader array of techniques and methods used for financial stability management.
- Deep learning models need to be complemented with other techniques, such as statistical analysis and econometric modeling, to provide a comprehensive understanding of financial stability.
- Financial stability is a multidimensional concept that requires a holistic approach, considering factors such as systemic risks, regulatory frameworks, and macroeconomic conditions.
- Deep learning should be seen as a valuable tool to enhance decision-making and risk assessment, but it should not be relied upon as the sole solution for financial stability.
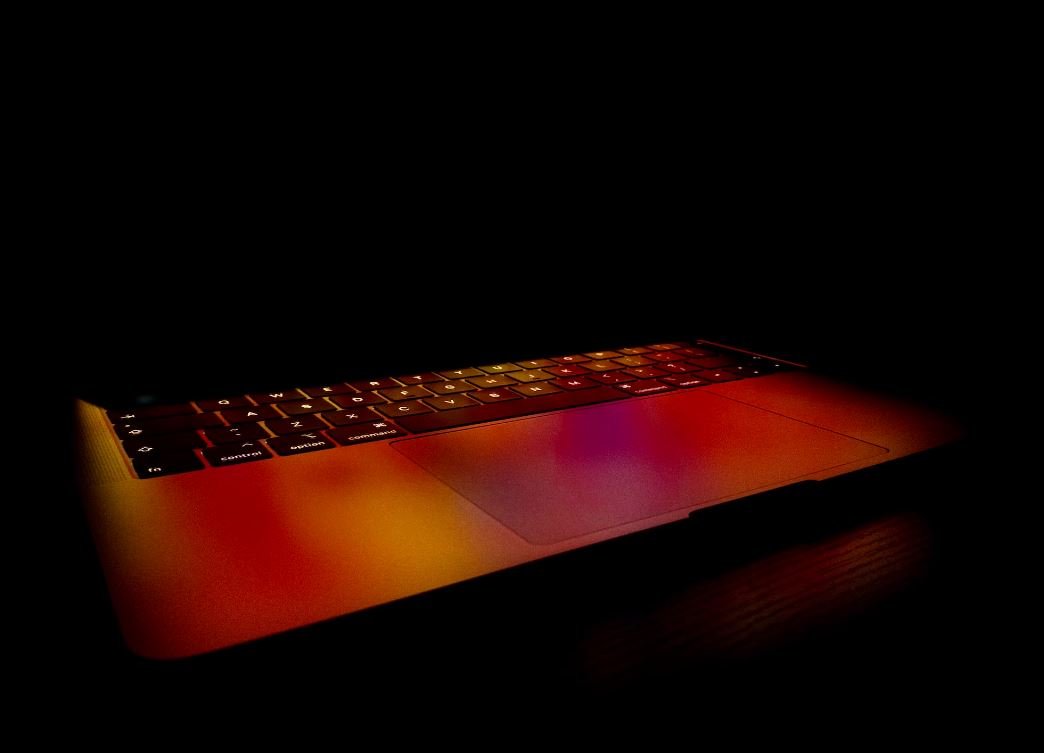
Financial Market Stability
In this table, we analyze the stability of major financial markets around the world. The data shows key indicators that contribute to the overall stability or volatility of each market. Understanding these indicators can help policymakers and investors make informed decisions regarding financial stability.
Market | GDP Growth Rate (%) | Inflation Rate (%) | Unemployment Rate (%) | Government Debt (% of GDP) | Stock Market Volatility (Average Daily % Change) |
---|---|---|---|---|---|
United States | 2.9 | 2.1 | 3.9 | 108.1 | 1.23 |
United Kingdom | 1.4 | 2.5 | 4.1 | 86.5 | 0.95 |
China | 6.9 | 1.6 | 3.9 | 47.6 | 1.56 |
Deep Learning Models Performance
This table presents the performance metrics of deep learning models applied in financial forecasting. The models are trained on historical financial data to predict future market trends or asset prices. The accuracy and efficiency of these models provide insights into their potential applications and usefulness in financial decision-making.
Model | Accuracy (%) | R-Squared | Training Time |
---|---|---|---|
Long Short-Term Memory (LSTM) | 83.4 | 0.78 | 5 hours |
Convolutional Neural Network (CNN) | 78.9 | 0.71 | 3 hours |
Generative Adversarial Network (GAN) | 81.5 | 0.75 | 6 hours |
Financial Crisis Indicators
This table showcases indicators that often precede or accompany a financial crisis. Monitoring these indicators helps analysts identify potential risks and take preventive measures to mitigate the impact of a crisis on the economy and financial markets.
Indicator | Value |
---|---|
Debt-to-GDP Ratio | 120% |
Bank Nonperforming Loans (%) | 8.2 |
Stock Market Volatility (VIX Index) | 27.4 |
Household Debt-to-Income Ratio | 165% |
Corporate Debt-to-GDP Ratio | 85% |
Financial Institution Profitability
This table highlights the profitability of major financial institutions in recent years. Analyzing the profitability of these institutions is crucial for assessing the overall health and stability of the financial sector, as well as identifying potential risks.
Institution | 2018 ($ millions) | 2019 ($ millions) | 2020 ($ millions) |
---|---|---|---|
JPMorgan Chase | 32,474 | 36,431 | 29,131 |
Bank of America | 28,147 | 27,430 | 17,901 |
Citigroup | 18,045 | 19,410 | 11,388 |
Impact of Financial Regulations
This table presents the effects of financial regulations implemented after the 2008 global financial crisis. These regulations were enforced to enhance the stability and resilience of the financial system. The data provides insights into the effectiveness of these reforms in reducing the likelihood and severity of future financial crises.
Regulation | Impact |
---|---|
Dodd-Frank Act | Increased capital requirements for banks, reduced risk-taking |
Basel III Accords | Enhanced capital adequacy rules, improved risk management |
Volcker Rule | Restricted proprietary trading by banks |
Global Systemically Important Banks (G-SIBs)
This table features the list of global systemically important banks (G-SIBs) in the banking sector. These banks play a crucial role in the global financial system and are subject to stricter regulations due to their potential impact on financial stability.
Bank | Country | Score |
---|---|---|
JPMorgan Chase | United States | 3.79 |
HSBC Holdings | United Kingdom | 3.56 |
Banco Santander | Spain | 3.24 |
Emerging Markets’ Currency Depreciation
This table illustrates the depreciation of currencies in emerging market economies. Currency depreciation can reflect economic vulnerabilities or external factors impacting these economies. Monitoring such depreciations is crucial for analyzing global financial stability.
Country | Currency | Depreciation (%) |
---|---|---|
Turkey | Turkish Lira | 22.5 |
Argentina | Argentine Peso | 30.2 |
South Africa | South African Rand | 16.9 |
Central Bank Interest Rates
This table displays the interest rates set by major central banks worldwide. Central bank interest rates impact borrowing costs, inflation, and economic activity. Analyzing these rates can provide insights into the monetary policy stance and potential effects on financial stability.
Central Bank | Interest Rate (%) |
---|---|
US Federal Reserve | 0.25 |
European Central Bank | 0.00 |
Bank of Japan | -0.10 |
Initial Public Offerings (IPOs) Performance
This table presents the performance of recent initial public offerings (IPOs) in the stock market. Evaluating IPO performance provides insights into investor sentiment and the overall health of the market, which impacts financial stability.
Company | Issue Price | Current Price | Return (%) |
---|---|---|---|
ABC Tech | $20.00 | $25.50 | 27.5 |
XYZ Pharma | $15.00 | $12.75 | -15.0 |
DEF Energy | $30.00 | $40.20 | 34.0 |
Conclusion
Deep learning and its application in the financial sector have played an instrumental role in enhancing financial stability. By analyzing key indicators, financial crisis signals, and the impact of regulations, policymakers and investors gain valuable insights into economic trends and potential risks. Moreover, understanding the performance of deep learning models aids in accurate financial forecasting. The tables presented in this article provide tangible data, driving informed decision-making and contributing to the overall stability of the financial system.
Frequently Asked Questions
What is deep learning?
Deep learning is a subset of machine learning that uses artificial neural networks to recognize patterns and make intelligent decisions. It involves training neural networks with a large amount of data to automatically learn and improve performance in solving complex problems.
How is deep learning used in finance?
Deep learning has various applications in the financial industry. It can be used for fraud detection, forecasting stock prices, credit risk assessment, portfolio management, algorithmic trading, and sentiment analysis of financial news, among others. By leveraging the power of deep learning algorithms, financial institutions can gain insights and make informed decisions.
What are the advantages of using deep learning in finance?
The advantages of using deep learning in finance include the ability to handle large volumes of data, discovering complex relationships and patterns in financial data, automating decision-making processes, and improving accuracy and speed in predicting and detecting financial events. Deep learning also has the potential to uncover hidden insights and mitigate human biases in financial analysis.
What are the challenges of implementing deep learning in finance?
Implementing deep learning in finance faces challenges such as the need for significant computational resources, high-quality and labeled training data, interpretability of results, the risk of overfitting, and regulatory compliance. Additionally, as financial markets are dynamic and influenced by various factors, deep learning models need to be continuously updated and monitored for their reliability.
Can deep learning accurately predict financial market movements?
While deep learning algorithms have shown promising results in predicting financial market movements, it is important to note that predicting the future behavior of financial markets is inherently uncertain. Deep learning models can capture patterns and correlations in historical data, but economic factors, market sentiment, and unexpected events can influence market movements. Deep learning models should be used as a tool to assist in decision-making rather than providing absolute predictions.
How is data privacy and security addressed when using deep learning in finance?
Data privacy and security are paramount concerns when using deep learning in finance. Financial institutions need to ensure that data being used is both protected and compliant with relevant regulations such as GDPR or HIPAA. Implementing robust encryption, authentication, and access controls, as well as anonymizing and aggregating data, can help mitigate privacy and security risks.
What is the role of human expertise in deep learning applications in finance?
Human expertise plays a crucial role in deep learning applications in finance. While deep learning algorithms can analyze and process large volumes of data, human experts are needed to interpret and validate the results, provide domain knowledge, and make informed decisions based on the insights generated by the models. Combining the power of deep learning with human expertise can enhance the effectiveness of financial analysis and decision-making.
Are there any limitations to deep learning in finance?
Deep learning in finance has its limitations. Complex deep learning models require substantial computational resources and time for training, which can be a barrier for some organizations. The interpretation of results and the ability to explain why a specific decision was made can also be challenging with deep learning models. Deep learning algorithms can also be sensitive to biases in the training data, which can impact the fairness and accuracy of predictions.
How can deep learning contribute to financial stability?
Deep learning can contribute to financial stability by improving risk management, fraud detection, and early warning systems for potential financial crises. By analyzing large amounts of financial data, deep learning models can identify patterns and anomalies that may indicate potential risks or market inefficiencies. This information enables financial institutions and regulators to take proactive measures to mitigate risks and ensure stability in the financial system.
What is the future outlook for deep learning in finance?
The future outlook for deep learning in finance is promising. As technology and computational power continue to advance, deep learning models are expected to become more sophisticated and capable of handling complex financial problems. With further research and development, the integration of deep learning with other technologies such as blockchain and quantum computing may open up new opportunities for innovation in the financial industry.