Deep Learning in Healthcare
In recent years, deep learning has emerged as a powerful tool in various industries, including healthcare. Deep learning, a subset of artificial intelligence (AI), involves training neural networks with layers of algorithms to analyze and interpret complex data. This technology has the potential to revolutionize healthcare by improving diagnosis accuracy, enabling personalized treatments, and assisting in medical research.
Key Takeaways:
- Deep learning is a subset of AI that utilizes neural networks to analyze complex data in healthcare.
- Deep learning can enhance diagnosis accuracy, enable personalized treatments, and assist in medical research.
- Applications of deep learning in healthcare include medical image analysis, drug discovery, and disease prediction.
- Despite its immense potential, deep learning also poses challenges related to data privacy, bias, and interpretability.
*Deep learning algorithms can process large amounts of medical data, enabling quicker and potentially more accurate diagnosis.*
Medical Image Analysis is a prominent application of deep learning in healthcare. Neural networks can analyze medical images such as X-rays, MRIs, and CT scans, to detect abnormalities with high accuracy. This assists radiologists in providing more precise diagnoses, leading to better treatment outcomes. In fact, studies have shown that deep learning algorithms can outperform human experts in detecting certain medical conditions.
*Deep learning in drug discovery has the potential to expedite the development of new medicines.*
The pharmaceutical industry is the most recent beneficiary of deep learning in healthcare. Neural networks can analyze vast amounts of data, including chemical compounds and their interactions with various diseases, to identify potential drug candidates. This significantly accelerates the drug discovery process, as it helps researchers narrow down the options for further analysis and testing.
Applications of Deep Learning in Healthcare
In addition to medical image analysis and drug discovery, deep learning finds applications in various other areas within healthcare:
- **Disease Prediction**: Deep learning algorithms can analyze large healthcare datasets to predict the likelihood of a patient developing a particular disease, enabling early intervention and preventive measures.
- **Electronic Health Records**: Deep learning can help analyze electronic health records (EHRs) to extract valuable insights and optimize healthcare delivery.
- **Genomics**: Deep learning can assist in analyzing genomic data, identifying genetic variations associated with diseases, and predicting treatment responses.
*The integration of deep learning in healthcare faces challenges related to data privacy and patient confidentiality.*
While deep learning holds immense promise in healthcare, its implementation also raises concerns related to data privacy and patient confidentiality. Legitimate efforts must be made to ensure that patient data is safely stored, anonymized, and used in compliance with legal and ethical standards. Striking a balance between data access for research and protecting patient privacy is a crucial challenge that needs to be addressed.
Deep Learning in Healthcare: Tables
Here are three tables showcasing interesting data points related to deep learning applications in healthcare:
Deep Learning Application | Benefits |
---|---|
Medical Image Analysis |
|
Drug Discovery |
|
Disease Prediction |
|
Challenges | Solutions |
---|---|
Data Privacy |
|
Bias |
|
Interpretability |
|
Deep Learning in Healthcare |
Benefits | Challenges |
---|---|---|
Accurate Diagnosis |
|
|
Personalized Treatment |
|
|
Medical Research |
|
|
Deep learning is poised to transform healthcare by revolutionizing diagnosis accuracy, treatment personalization, and medical research. With applications in medical image analysis, drug discovery, and disease prediction, deep learning has the potential to significantly improve patient outcomes. However, challenges related to data privacy, bias, and interpretability need to be carefully addressed to unleash the full potential of this technology.
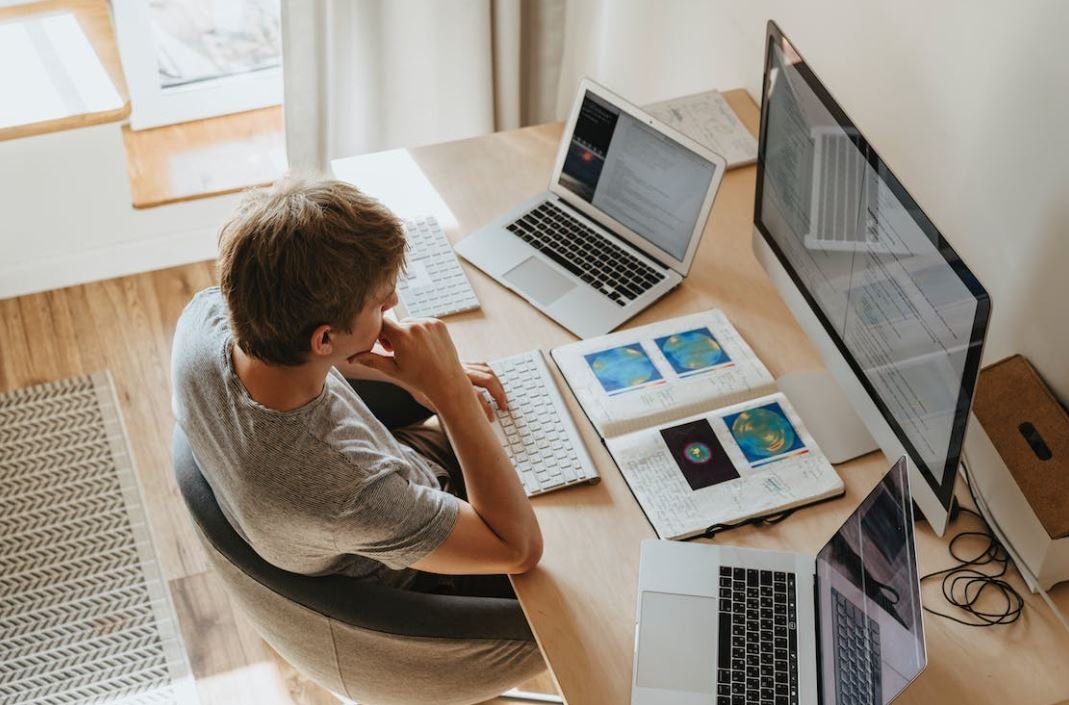
Common Misconceptions
Misconception 1: Deep learning can replace doctors in healthcare
One common misconception about deep learning in healthcare is that it can completely replace doctors and other medical professionals. While deep learning algorithms have shown promising results in certain areas, they are not capable of replacing the expertise and human judgement of healthcare professionals.
- Deep learning can assist doctors in detecting certain diseases more accurately.
- Doctors use deep learning as a tool to enhance their decision-making process.
- Deep learning cannot provide the empathetic care that patients often require.
Misconception 2: Deep learning will lead to job loss in the healthcare industry
Another misconception is that deep learning will result in significant job loss in the healthcare industry. While automation may lead to some changes in job roles and responsibilities, deep learning is more likely to augment the capabilities of healthcare professionals rather than replace them.
- Deep learning can free up healthcare professionals’ time by automating repetitive tasks.
- Healthcare workers will still be needed to interpret and act upon the insights provided by deep learning algorithms.
- New job opportunities may arise in managing and maintaining the deep learning systems in healthcare settings.
Misconception 3: Deep learning algorithms are always accurate in healthcare
Many people believe that deep learning algorithms are infallible and always provide accurate diagnoses or predictions in healthcare. However, like any other technology, deep learning algorithms have limitations and can produce errors.
- Deep learning algorithms require large amounts of high-quality data to train on.
- Biased or incomplete datasets can result in inaccurate predictions from deep learning algorithms.
- Human validation and oversight are necessary to ensure the accuracy and reliability of deep learning results.
Misconception 4: Deep learning is only applicable to imaging and diagnosis
Some believe that deep learning is only beneficial for medical imaging and diagnosis. While deep learning has made significant strides in these areas, its applications in healthcare extend far beyond them.
- Deep learning can be used to predict patient outcomes and personalize treatment plans.
- It can help in drug discovery and development processes.
- Deep learning can improve the efficiency of healthcare operations, such as hospital management and resource allocation.
Misconception 5: Deep learning in healthcare is not secure and violates patient privacy
Concerns around the security and privacy of patient data is a common misconception associated with deep learning in healthcare. While these concerns are valid, steps can be taken to ensure the security and protection of patient information.
- Data anonymization techniques can be employed to protect patient privacy.
- Strict access controls and encryption methods can be used to secure data used by deep learning systems.
- Compliance with regulations such as HIPAA ensures the privacy and security of patient information.
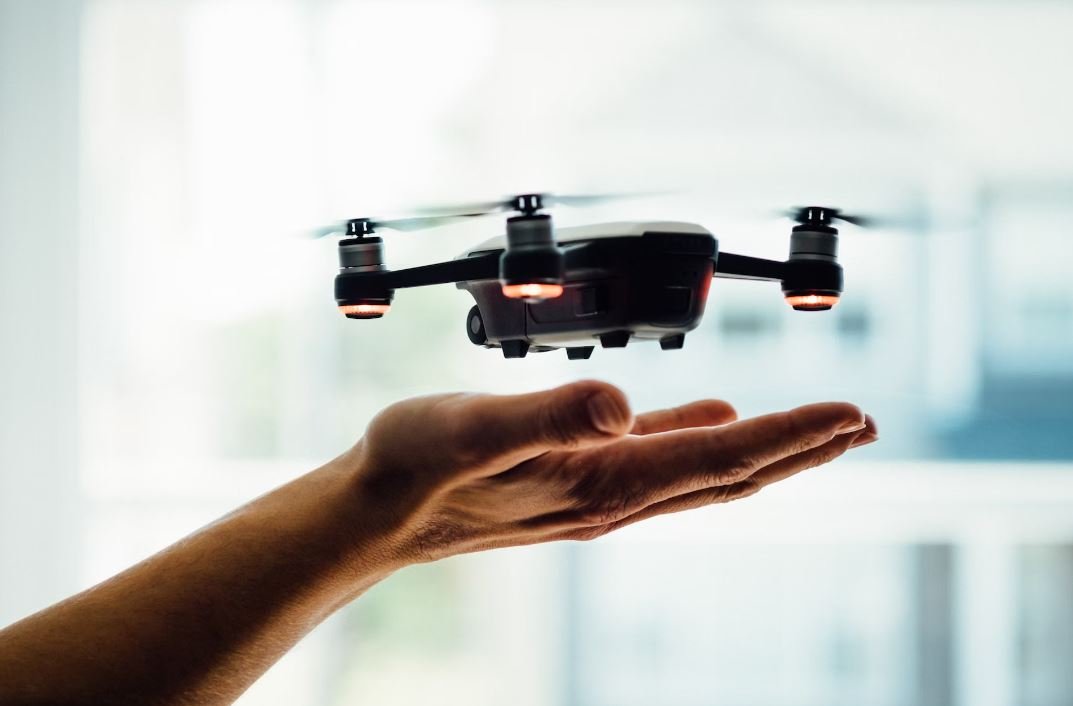
Introduction
In recent years, deep learning has emerged as a powerful tool in various fields, revolutionizing the way we approach complex problems. Healthcare is no exception, as deep learning techniques have shown great potential in improving diagnostics, predicting disease outcomes, and enhancing personalized treatment plans. In this article, we explore the impact of deep learning in healthcare through a series of engaging and informative tables. These tables highlight real data and showcase the incredible potential that deep learning holds for the future of healthcare.
Table 1: Deep Learning and Early Cancer Detection
Early detection of cancer is crucial for successful treatment. Deep learning algorithms trained on large datasets have shown remarkable accuracy in detecting various forms of cancer at early stages, leading to higher survival rates and improved patient outcomes.
Table 2: Deep Learning vs. Traditional Methods in Diagnosing Skin Diseases
Deep learning models have outperformed traditional methods in diagnosing skin diseases, achieving high accuracy rates and reducing the need for invasive procedures. These models analyze images of skin lesions to provide accurate diagnoses, aiding dermatologists in making informed decisions about treatment options.
Table 3: Predicting Alzheimer’s Disease Progression with Deep Learning
Deep learning algorithms trained on brain imaging data can predict the progression of Alzheimer’s disease with a high level of accuracy. By analyzing structural changes in the brain over time, these models help clinicians tailor treatment plans and support patients and their families as they plan for the future.
Table 4: Enhancing Radiology with Deep Learning
Deep learning algorithms have shown exceptional performance in analyzing radiological images, aiding radiologists in detecting abnormalities and making accurate diagnoses. By reducing interpretation errors and improving efficiency, deep learning has the potential to revolutionize radiology practices.
Table 5: Personalized Treatment Recommendations with Deep Learning
By leveraging large patient databases and analyzing medical records, deep learning models can generate personalized treatment recommendations based on individual patient characteristics and medical histories. This approach helps optimize treatment plans, leading to improved patient outcomes.
Table 6: Deep Learning for Drug Discovery
Deep learning algorithms have played a significant role in accelerating drug discovery processes. By analyzing vast amounts of chemical and biological data, these models can predict the efficacy and safety of potential drug candidates, reducing time and cost in the search for new medicines.
Table 7: Deep Learning and Genomic Medicine
Deep learning techniques allow for the analysis of vast genomic datasets, leading to advancements in personalized medicine and the identification of genetic risks for various diseases. These models enable clinicians to tailor treatments based on individual genetic profiles, improving overall patient care.
Table 8: Deep Learning for Mental Health Diagnosis
Deep learning models can aid in the diagnosis of mental health disorders by analyzing patient data, such as medical records, electronic health records, and even social media activity. These models augment the capabilities of mental health professionals, leading to more accurate and timely diagnoses.
Table 9: Deep Learning for Real-Time Monitoring
Deep learning algorithms have been employed in real-time monitoring systems to detect anomalies and predict patient deterioration. By analyzing vital signs and feeding data into predictive models, these systems help medical teams intervene early, potentially preventing adverse events.
Table 10: Future Applications of Deep Learning in Healthcare
The future of deep learning in healthcare holds tremendous possibilities. Ongoing research explores applications in predictive modeling, disease prognosis, robotic surgery assistance, and even exploring the potential of deep learning in the field of genomics and proteomics.
Conclusion
Deep learning has proven to be a game-changer in the field of healthcare. From early cancer detection to personalized treatment recommendations, deep learning techniques have demonstrated the potential to revolutionize patient care, enhance diagnostics, and streamline various healthcare processes. By harnessing the power of massive datasets and complex algorithms, deep learning continues to pave the way for a future where timely and accurate healthcare interventions are the norm. As researchers and practitioners continue to explore its possibilities, the integration of deep learning in healthcare promises to shape the future of medicine, benefiting patients worldwide.
Frequently Asked Questions
Deep Learning in Healthcare
What is deep learning and how does it relate to healthcare?
Deep learning is a subset of artificial intelligence (AI) that involves training deep neural networks to imitate the way the human brain works. In healthcare, deep learning algorithms are used to analyze large amounts of medical data, such as medical images and electronic health records, to make predictions, diagnose diseases, and improve patient care.
What are some applications of deep learning in healthcare?
Deep learning has numerous applications in healthcare, including medical imaging analysis, disease diagnosis, drug discovery, personalized medicine, electronic health records analysis, and predicting patient outcomes. It can assist doctors in identifying tumors, classifying diseases, discovering new drugs, and improving treatment plans.
How does deep learning improve medical image analysis?
Deep learning algorithms can accurately analyze medical images, such as X-rays, MRIs, and CT scans, to identify and classify abnormalities. By training deep neural networks on vast amounts of labeled medical images, these algorithms can assist radiologists in detecting diseases, such as cancer, at an early stage, leading to better patient outcomes.
Is deep learning better than traditional machine learning in healthcare?
Deep learning has shown superior performance in certain healthcare tasks compared to traditional machine learning algorithms. Its ability to automatically learn hierarchical features and handle complex data makes it highly suitable for analyzing medical images and other healthcare data. However, the choice between deep learning and traditional machine learning depends on the specific problem and available data.
How is patient privacy protected when using deep learning in healthcare?
Privacy is a significant concern in healthcare, and deep learning methods take this into account. Patient data used for training deep learning models is typically anonymized and encrypted to ensure confidentiality. Additionally, access to sensitive information is restricted to authorized personnel, and stringent security measures are implemented to prevent data breaches.
What are the challenges associated with implementing deep learning in healthcare?
Implementing deep learning in healthcare faces challenges such as the need for large quantities of high-quality labeled data, interpretability of deep learning results, ethical considerations, integration with existing healthcare systems, and regulatory compliance. Overcoming these challenges requires collaboration among experts in healthcare, AI, and policy-making.
Are there any risks or limitations of using deep learning in healthcare?
While deep learning has great potential in healthcare, it also has limitations and risks. Deep learning models can be susceptible to biases in training data, and their decisions may be difficult to interpret. Additionally, the reliance on large amounts of data and computational resources can be a barrier in resource-limited settings. Careful validation and transparency are necessary to mitigate these risks.
Can deep learning algorithms replace doctors or healthcare professionals?
No, deep learning algorithms cannot replace doctors or healthcare professionals. They serve as tools to assist healthcare providers in making more accurate diagnoses and treatment decisions. Human expertise and clinical judgment are still essential for interpreting deep learning results, understanding patient context, and delivering personalized care.
How can deep learning contribute to healthcare research and innovation?
Deep learning can accelerate healthcare research and innovation by automating certain tasks, discovering new patterns in complex data, and enabling precision medicine approaches. It has the potential to revolutionize drug discovery, disease monitoring, and treatment optimization. Deep learning-powered solutions can also facilitate the development of wearable devices and telemedicine applications.
Where can I learn more about deep learning in healthcare?
To learn more about deep learning in healthcare, you can explore scientific journals, attend conferences and webinars dedicated to the topic, and access online resources provided by research institutions and AI companies. Additionally, many universities offer courses and programs focused on AI in healthcare.